Focus period Lund 2022
Seminar series by visiting scholars, full program
April 20, 10:30 - 12:00
Automatic Control Seminar room (KC 3N27)
How T-cells select targets and get selected for them
Jonathan Desponds, Institute Roche, France
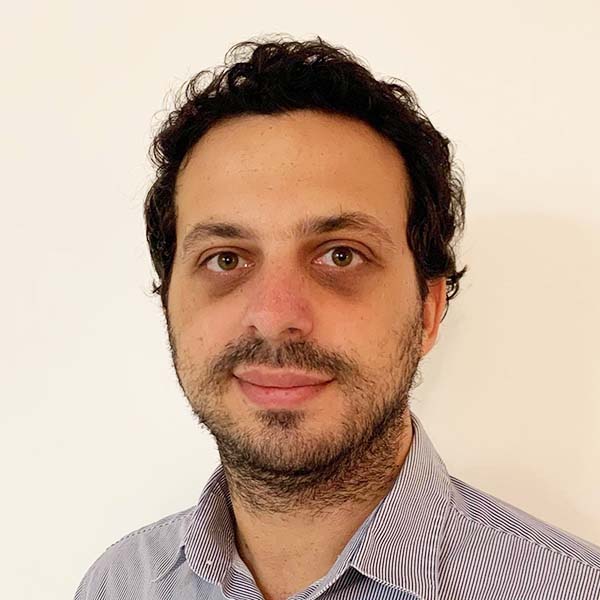
Abstract
The adaptive immune system responds to pathogens by selecting clones of cells with specific receptors. While clonal selection in response to particular antigens has been studied in detail, it is unknown how a lifetime of exposures to many antigens collectively shape the immune repertoire. Using mathematical modeling and statistical analyses of T cell receptor sequencing data, I will describe a quantitative theory of human T cell dynamics compatible with the statistical laws of repertoire organization. I will show that clonal expansions during a perinatal time window leave a long-lasting imprint on the human T cell repertoire, which is only slowly reshaped by fluctuating clonal selection during adult life, with implications for pathogen defense and autoimmunity.
Biography
Trained in mathematics and physics, Jonathan Desponds has been applying quantitative methods to biological and biomedical research since his PhD at ENS in Paris, with applications in the fields of developmental biology, immunology and immuno-oncology. He has worked as postdoctoral scholar at the physics department of UC San Diego and as a fellow at the NSF-Simons center for quantitative biology at Northwestern University. He then joined the Health Data Hub, a French national initiative to gather and link health datasets in the interest of research, as a scientific project manager. Since Octobre 2021, he has been working at Institut Roche, in Paris, to develop and improve cancer immunotherapies.
He has experience applying methods from statistics, probability theory, statistical mechanics and machine learning to inference and modeling of diverse biological processes. Those processes include: transcription regulation, cellular decision-making, selection of adaptive immune cells,spatial organization of developing organisms, population dynamics of immune repertoires and response to cancer immunotherapy.
The role of software and its development in scientific research
Fredrik Bagge Carlsson, Julia Computing, USA
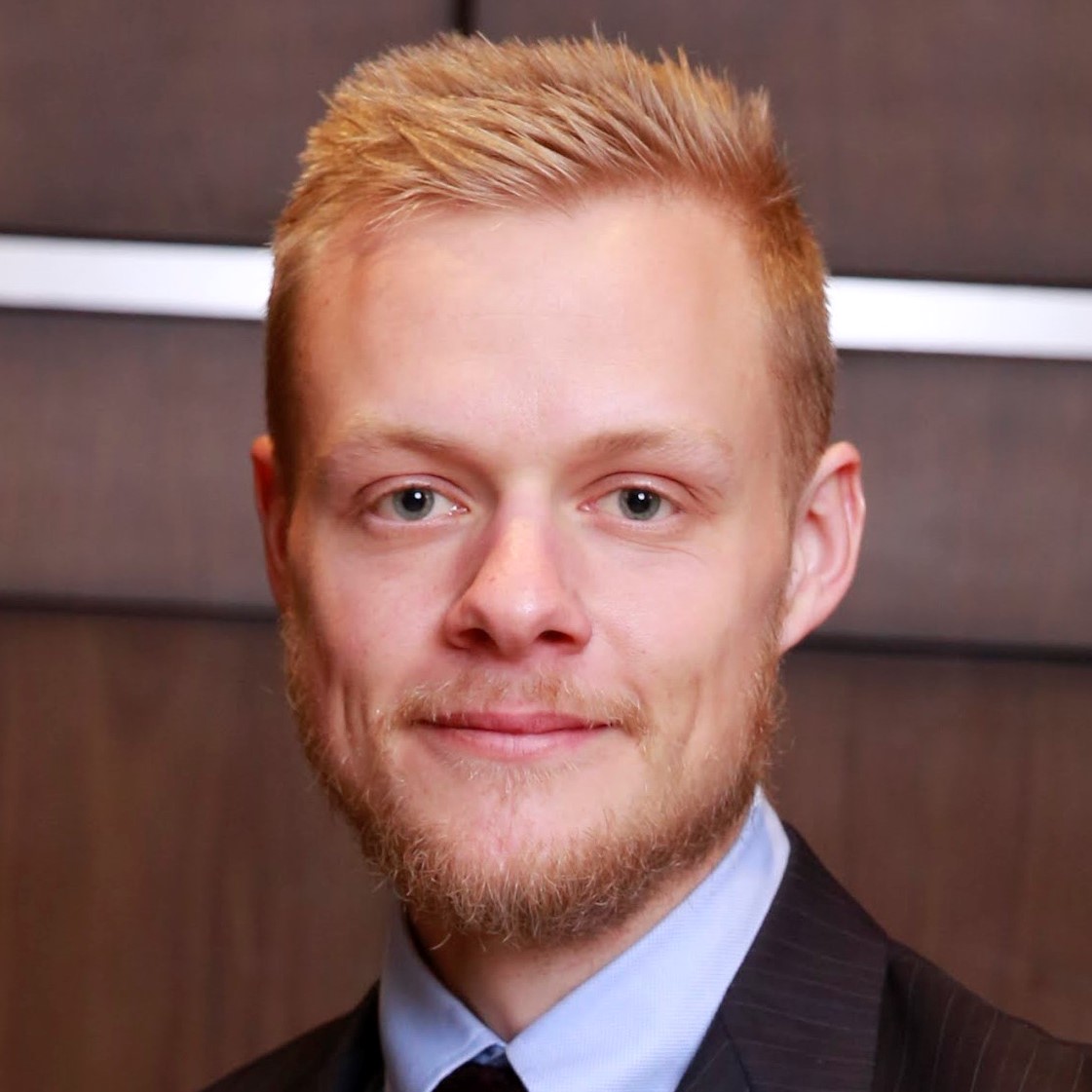
Abstract
In this talk, we will explore how modern computational tools can help bring machine learning and domain-specific modelling together. We will talk about what standard machine learning is good for, and where it often falls short. Hard-earned models and knowledge, built through decades of research, have often been ignored in the era of data-driven discovery, oftentimes for the wrong reasons. Does it have to be this way?
We will approach the problem from a technological viewpoint, and answer questions around the use of simulators of physics and biological systems in machine-learning pipelines, and what it takes to do so efficiently. We will also touch upon the role of open-source and its community in research software creation, and how collaboration over research code can foster greater advancements and yield a sum that is greater than its parts. We will further talk about what the research community can learn from best practices in software development, in particular, we will touch upon the reproducibility problem and how it relates to software.
Biography
Fredrik received his MSc and PhD 2019 from the Dept. Automatic Control in Lund, Sweden, working within the fields of control, machine learning and robotics. Fredrik has since spent a year with the Acoustic Research Laboratory at NUS and subsequently made the transition to industry, working with dynamic modeling, control and programming-language design in a robotics context. Fredrik is currently working with Julia Computing on software tools for acasual modeling, simulation, optimization and control in the Julia programming language.
Fredrik’s personal interests include open-source software, hiking and outdoor cooking.
April 21, 9:15 - 12:00
BMC Segerfalksalen
Mathematical modelling of autoimmune myocarditis and the effects of immune checkpoint inhibitors
Solveig van der Vegt, University of Oxford, England
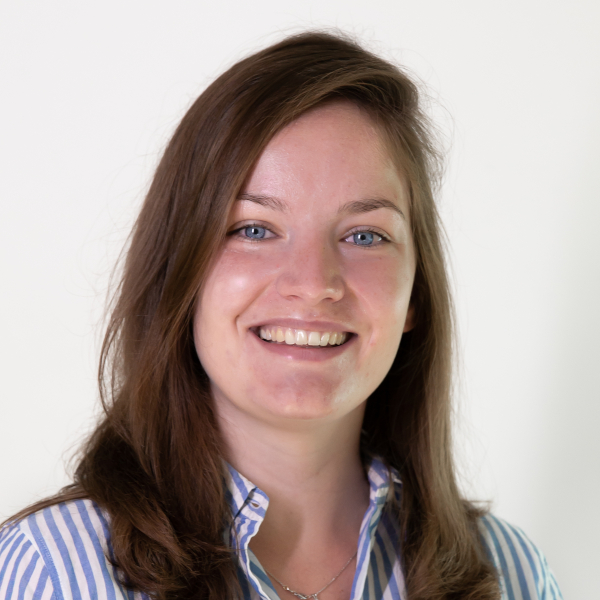
Abstract
Autoimmune myocarditis is a rare, but frequently fatal, side effect of immune checkpoint inhibitors (ICIs), a class of cancer therapies. Despite extensive experimental work on the causes, development, and progression of this disease, much still remains unknown about the importance of the different immunological pathways involved. To further our understanding of the immunological mechanisms underlying autoimmune myocarditis and the cardiotoxicity associated with ICIs, we build the first mathematical model of this disease. We show that this model, when parameterized based on literature insights, can reproduce the qualitative behaviour we expect based on disease characteristics observed in patients.
Balancing the positive effects of ICIs in treating a tumour with the detrimental effects of increasing the risk of autoimmune myocarditi, lends itself well to the use of optimal control theory. We show that, in a scenario where the standard-of-care treatment schedule used for ICIs causes the development of autoimmune myocarditis, optimal control theory can be used to establish a wide range of schedules with which the same number of doses of ICIs can be applied while autoimmune myocarditis is prevented.
Reference Solveig A. van der Vegt, Liudmila Polonchuk, Ken Wang, Sarah L. Waters, Ruth E. Baker (2022). Mathematical modelling of autoimmune myocarditis and the effects of immune checkpoint inhibitors. Journal of Theoretical Biology, 537 (111002). doi:10.1016/j.jtbi.2021.111002.
Biography
Solveig van der Vegt is a fourth-year PhD student at the Wolfson Centre for Mathematical Biology at the University of Oxford. She graduated from Cornell University in 2018 with a BA in Biological Sciences with a minor in Mathematics, and Solveig now combine these two fields in her PhD research. Her main project focuses on modeling autoimmune myocarditis and the effects of immune checkpoint inhibitors on the development and progression of this disease.
A Deep Learning Pipeline for COVID19-related Information Extraction from Scientific Literature
Rafsan Ahmed, Lund University, Sweden
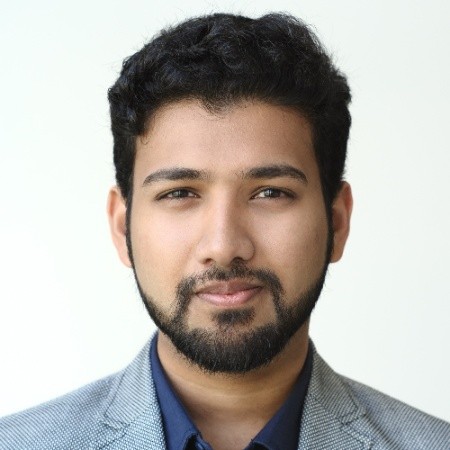
Abstract
The COVID19 pandemic has prompted the biomedical and healthcare field to accelerate the pace of research, which generated an extensive amount of research articles in this domain. The integration of information from such a large body of research could provide key insights into the disease such as biological mechanisms and new approaches for treatment. However, the scale and complexity of the data greatly surpasses human processing abilities. In order to process and extract information from such data, we look into deep learning-based natural language processing that can help to extract and connect this information.
We developed an end- to -end, information extraction pipeline trained on Named Entity Recognition (NER) corpora. The pipeline can process large text collections and incorporates a series of deep learning models trained to identify domain- specific entities such as cell lines, chemicals, diseases, genes and species. The pipeline was then deployed on the CORD19 dataset, a collection of over 400000 research articles on COVID-19.
References
1. Weber, L., Sanger, M., Munchmeyer, J., Habili, M., Leser, U. and Akbik, A. “HUNER: Improving Biomedical NER with pretraining”, Bioinformatics, Volume 36, Issue 1, Jan 2020, 295-302.
2. Wang, L.L., Lo, K., Chandrasekhar, Y., Reas, R. et al. “CORD 19: The Covid 19 Open Research Dataset.” Preprint. ArXiv . 2020; arXiv:2004.10706v2. Published 2020 Apr
3. Lee, J., Yoon, W., Kim, S., Kim, D., Kim, S., So, C.H., and Kang, J. “BioBERT : a pretrained biomedical language representation model for biomedical text mining”, Bioinformatics, Volume 36, Issue 4, 2020, 1234-1240.
Biography
Rafsan Ahmed is a second year PhD student at the Cell Death, Lysosomes and AI (CDLAI) group at Lund University. His research focus is AI in medicine, which spans from using Natural Language Processing (NLP) to discover drug targets for diseases to microscopic cell image segmentation through machine learning models. Previously, he has also worked with network based algorithms to identify cancer driver (causing) genes.
Rafsan did his B.Sc. in Electrical Engineering, which is a completely different world to what he is doing right now. Back then he was building robots and even managed to build an electric car. But towards the end of his undergraduate studies he decided to change tracks and enter into the fascinating field of Machine Learning. Now he spends his days learning about how machine learning can be used to greatly impact the healthcare field (and of course, how to avoid an AI takeover).
Getting personal with epigenetics: towards machine-learning-assisted precision epigenomics
Giovanni Visona. Max Planck Institute for Intelligent Systems Tübingen, Germany.
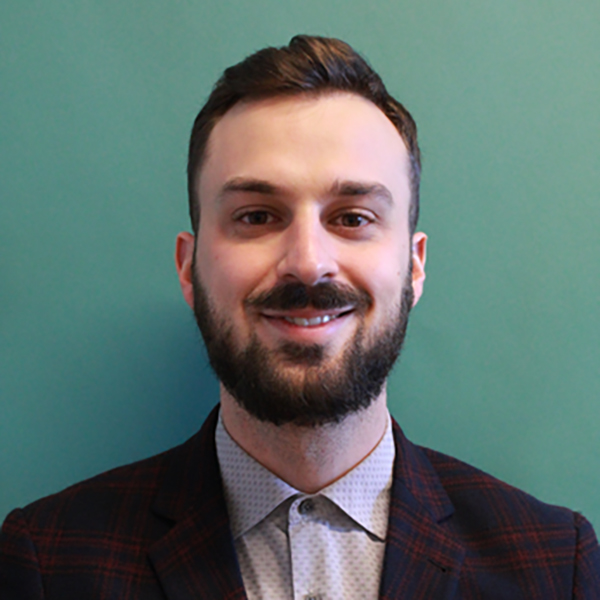
Abstract
Epigenetic modifications are dynamic control mechanisms involved in the regulation of gene expression. Unlike the DNA sequence itself, they vary not only between individuals but also between different cell types of the same individual. Exposure to environmental factors, somatic mutations, and ageing contribute to epigenomic changes over time, which may constitute early hallmarks or causal factors of disease. Epigenetic changes are reversible and, therefore, promising therapeutic targets. However, mapping efforts to determine an individual’s cell-type-specific epigenome are constrained by experimental costs. We developed eDICE, an attention-based deep learning model, to impute epigenomic tracks. In addition to benchmarking
results against state of the art models, I will present a proof of concept for the imputation of personalised epigenomic measurements on the ENTEx dataset, where eDICE correctly predicts individual- and cell-type-specific epigenetic patterns.
Biography
Giovanni graduated in medical physics at the University of Trent (Italy), and moved to machine learning with an MSc at the University of Edinburgh. He joined the Max-Planck Institute for Intelligent Systems in Tübingen at the end of 2019 for a PhD, and is now a part of the Marie Curie Innovative Training Network entitled “Machine Learning Frontiers in Precision Medicine”. In his work Giovanni focus on the application of deep learning and representation learning models to biomedical data, with a particular interest for sequencing data.
April 22, 10:30 - 12:00
Automatic Control Seminar room (KC 3N27)
Trends in optimization for radiation therapy
Michelle Böck. Dana-Farber Cancer Institute and Harvard Medical School, USA
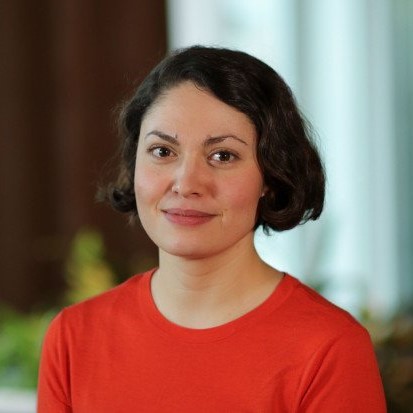
Abstract
Radiation therapy (RT) uses ionizing radiation to deliver a curative dose to the tumor to eradicate tumor cells. Typically, radiation is delivered daily over several weeks which is known as fractionation. Every radiation therapy treatment is proceeded by the process of treatment planning, which involves finding the ‘optimal’ tradeoffs, such as between tumor control and normal tissue sparing, between time-efficiency and dose quality, and between initial plan quality and robustness further into the
treatment. Technological advances and research over the past decades have allowed radiation therapy treatments to gain in complexity and accuracy. The technological advancement especially in dose delivery techniques and the introduction of intensity-modulated radiation therapy in the 1990s gave rise to the use of mathematical optimization due to the largely increased degrees of freedom in machine and treatment parameters.
In this talk, we will discuss topics on (i) increasing the accuracy of optimization tools to increase time
efficiency and accuracy; and (ii) handling uncertainties occurring during the week-long course of radiation treatment through means such as stochastic programming and robust optimization.
First, the accuracy of optimization tools can be improved by changing the underlying objective functions and optimization approaches to better mimic the clinical intent of the physician. Achieving a tradeoff between the dose delivered to the tumor and healthy tissue sparing is a lengthy process as it involves several iterations. Second, variations occurring during the fractionated treatment if not addressed can lead to underdose of the tumor and/or overdose of healthy tissue which may compromise overall treatment quality. Thus, addressing the individual uncertainties through means of stochastic programming and robust optimization combined with adaptive reoptimization of the treatment plan can mitigate the impact of these uncertainties on the intended dose distribution and treatment quality.
Biography
Michelle Böck, Ph.D. is a postdoctoral research fellow in the Department of Medical and Biophysics at the Dana-Farber Cancer Institute and Harvard Medical School in Boston, Massachusetts. Her main research and industry experience lie at the intersection of mathematics, physics, and medicine. She currently works in both clinical and theoretical research to improve radiation therapy treatment planning methods to increase clinical efficiency and accuracy of optimization tools. Michelle received her Ph.D. Degree in Applied & Computational Mathematics from KTH Royal Institute of Technology, Stockholm, Sweden, and her Master’s Degree in Engineering Physics from the Vienna University of Technology (TU Wien). Michelle’s Ph.D. research was done in a collaboration between the KTH Department of Mathematics and RaySearch Laboratories. During her doctoral studies, she developed and studied mathematical models based on stochastic programming and robust optimization to handle interfractional anatomical variations occurring during radiation therapy. After having received her Ph.D. degree in the summer of 2019, Michelle continued to work on optimization methods in the research department at RaySearch Laboratories before joining the Dana-Farber Cancer Institute and Harvard Medical School in early 2020.
Deciphering the roles of sex hormones in the human immune system
Camila R. Consiglio. Karolinska Institute, Sweden
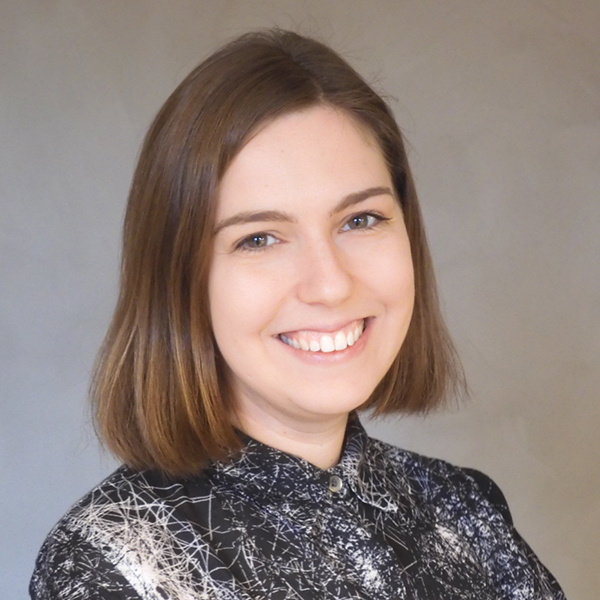
Abstract
Growing evidence indicates that biological sex impacts human immune responses, modulating susceptibility and severity to immune-related diseases. Females generally mount more robust immune responses than males, resulting in lower frequency and severity of infections and cancer, greater incidence of autoimmunity, and increased immune responses upon vaccination. While sex differences are attributed to the combined effects of sex hormones, genetics and societal factors, distinguishing the specific contribution of these factors in humans is challenging. Here, we addressed the precise contribution of sex hormones to human immune function by analyzing a cohort of subjects undergoing either Female-to-Male (FtM) or Male-to-Female (MtF) sex reassignment therapy with cross hormone treatment. Systems-level immunomonitoring was performed through mass cytometry, RNA-Sequencing and plasma proteome profiling of blood samples at baseline and following 3 and 12 months of treatment. We observed that testosterone affects immune plasma proteins differently between transitions. During FtM transition, the increase in testosterone levels correlated with a proinflammatory plasma proteome characterized by increased EN-RAGE, TNF and IL-17C; conversely, testosterone levels correlated with lower inflammatory profile during MtF transition, with decreased IL-12b, CXCL11 and TNFb. Testosterone induced an immune compositional change in FtM subjects over the course of treatment, with plasmacytoid dendritic cell (pDC) counts decreasing with testosterone levels. pDC phenotypic changes were also observed, where pDCs expressing lower HLA-DR and increased CD33 protein expression were associated with testosterone levels in both FtM and MtF transitions. Furthermore, testosterone levels modulated gene expression differently among the transitions. Both an IFNa response gene set and an antibody response predictive gene signature were enriched with testosterone levels in FtM subjects, indicative of more robust immune responses. In contrast, these gene sets were de-enriched with testosterone levels in MtF subjects. Our results shows that testosterone treatment has broad consequences to the human immune system, and significantly modulates important players in antiviral immunity and inflammatory response in a transition-dependent manner.
Consiglio, C.R. (1,2), Sardh, F. (3), Tadepally, L. (1,2), Pou, C. (1,2), Wang, J. (1,2), Mugabo, C.H. (1,2), Chen, Y. (1,2), Kämpe, O. (3), Landegren, N. (3,4), Brodin, P. (1,2)
1. Karolinska Institutet, Department of Women and Children’s Health, Solna, Sweden, 2. Science for Life Laboratory, Solna, Sweden, 3- Karolinska Institutet, Department of Medicine, Solna, Sweden, 4. Uppsala Universitet, Department of Medical Biochemistry and Microbiology, Uppsala, Sweden
Biography
Camila Consiglio is a Marie Skłodowska-Curie Fellow in the Brodin Lab at Karolinska Institutet (KI), Sweden, where she applies systems-level computational approaches to understand human immune function in health and disease. Originally from Brazil, Camila obtained her BS in Biomedicine and MSc in Genetics from Federal University of Rio Grande do Sul, Brazil. She holds a PhD degree in Immunology from Roswell Park Comprehensive Cancer Center (RPCCC), Buffalo, USA.
The overarching goal of Camila’s research is to understand how immune variation contributes to disease in humans. Growing evidence reveals that biological sex is an important driver of immune variability. Camila’s PhD research took advantage of murine models and pharmacological inhibitors to uncover how sex hormones regulate immunity in cancer (RPCCC, USA) and infection (9-month research stay at Institut Pasteur, France). Building on her Ph.D. work, she is now utilizing systems immunology approaches to dissect the contribution of sex hormones to human immune function as a MSCA fellow at KI.
April 25, 10:30 - 12:00
Automatic Control Seminar room (KC 3N27)
The ratio of CD8+ lymphocytes to CD68+CD163+ macrophages is prognostic in immunogenic tumors and predicts immunotherapy response
Artur Mezheyeuski, Uppsala University, Sweden
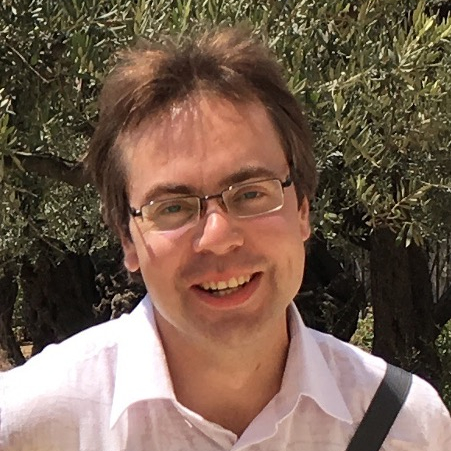
Abstract
Immune cells in the microenvironment shape tumor development and progression. Through in situ
analyses we assessed 15 immune cell classes in 352 colorectal cancers and identified a simple
prognostic signature based on the ratio of anti-tumoral CD8+ lymphocytes to tumor-supportive
CD68+CD163+ macrophages in the tumor microenvironment. The prognostic ability of this
signature was superior to the state-of-art immune score and was also demonstrated in four other
tumor types. Single-cell analyses identified these CD68+CD163+ macrophages as the source of
complement C1q, and the ratio of CD8A to C1QA gene expression levels in bulk RNA predicted
survival in five tumor types. In single cell analyses, RNA-based versions of the signature also
predicted response to checkpoint inhibitor therapy. This supports broad clinical applicability of
immune scores considering CD68+CD163+ macrophages as prognostic and predictive biomarkers in common cancers.
Biography
Born in Riga, Latvia and performed medical education (MD) and training at Riga’s Stradina University (Riga, Latvia) and later in Belarusian State Medical University (Minsk, Belarus) where AM became a specialist in pathology in 2008.
Supported by an exchange grant, AM conducted PhD training at the Karolinska Institute, Sweden, where he focused on the analyses of malignant tissue and its elements. After PhD training AM extended his efforts of spatial tissue analysis into tumour immunology. AM have set up a state-of-the-art multi-marker immunofluorescence method at host department and developed an analytical pipeline to address the spatial aspects and to evaluate cell-to-cell interaction and neighbouring patterns of different immune cell subclasses. AM have founded a Sweden-registered company providing pathology and image-analysis expertise for R&D (HistoOne AB). Research interest: the implementation of high-resolution spatial tissue analysis, with a special focus on tumor immunology.
Patient risk stratification and biomarker discovery in mantle cell lymphoma
Lavanya Lokhande Lund University, Sweden.
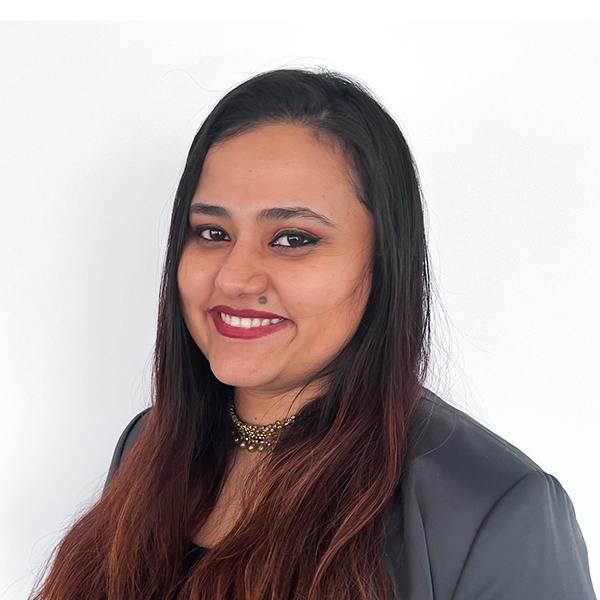
Abstract
For precision medicine, improved prognostic tools are needed to guide the treatment selection process and personalization. Understanding patient-to-patient biological variation and heterogeneity is the fundamental key for personalization and can be gained through high-throughput evaluation of clinical samples. With this agenda, various omic platforms have been developed, for multifaceted objectives using different clinical sample types. New platforms further require examination and development of bioinformatic pipelines to ensure accurate discovery. Often, pipelines can be specific to the technological platform, but several analytical workflows can be adapted for similar data outputs.
We have investigated how the immune system interacts with mantle cell lymphoma (MCL), through investigations of the tumor-immune microenvironment (TIME) but also the soluble immune response to the tumor cells. MCL is a rare and aggressive B-cell lymphoma subtype (median survival of 3-5 years). The current standard of care for diagnostic MCL is immunochemotherapy including targeting CD20 and autologous stem cell transplantation. However, a plethora of immune-targeting strategies, including checkpoint inhibitors and CAR T-cells are under investigation in clinical trials. Exploratory omic studies, not only help discern the biology but further guide patient risk classification and treatment selection.
In our previous work, we have evaluated primary and relapsed patient samples using GeoMx digital spatial profiler (DSP, Nanostring Inc.) for tissues and affinity-based protein microarray, IMMRay (Immunovia AB) for serum proteomics, respectively. In downstream analysis, several bioinformatic pipelines and machine learning algorithms were used to identify biomarker signatures that were associated with clinicopathological parameters, known risk factors and patient outcome. Based on our examination of different data outputs, we have highlighted challenges with data processing that need to be addressed, which include i) the need for biomarker signatures as opposed to single biomarkers to reach higher accuracy in risk predictions, ii) the challenge to account for intra-patient heterogeneity while predicting inter-patient variation and iii) the need for multi-omics integration to allow a deeper understanding based on more complex data from multiple molecular layers.
Biography
Lavanya is part of the CanFaster doctoral program, part of the European Union’s Marie Skłodowska-Curie COFUND programme, which aims to promote applied medical research with triple helix collaboration between academia, healthcare and the industry. She holds a BS-MS dual degree from Indian Institute of Science Education and Research (IISER) Pune, India and is currently a final year PhD fellow at the Department of Immunotechnology, Lund University.
Her work is centered around application of newly developed omics-based technologies based on serum proteomics and tissue-based spatial proteomics and transcriptomics, for biological exploration of Mantle Cell lymphoma (MCL), a rare and aggressive B-cell cancer subtype. Her research involves developing bioinformatic pipelines based on machine learning algorithms to identify patterns in the datasets which are correlated to clinicopathology and outcome. The final aim is to develop better patient risk stratification models and prognostic biomarker signatures.
April 26, 10:30 - 12:00
Automatic Control Seminar room (KC 3N27)
How bacteria generate beneficial phenotypic heterogeneity through mixed positive/negative feedback loops
Torkel Loman. University of Cambridge, England.
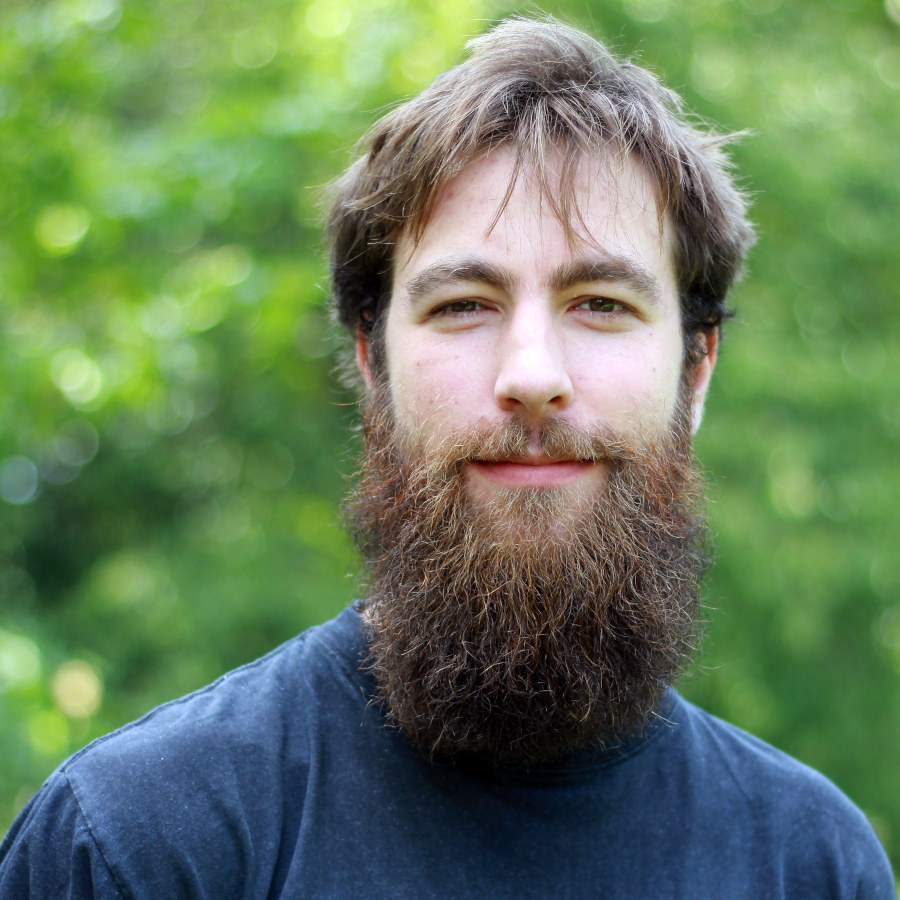
Abstract
Bacterial sigma factors (a class of transcription factors) are prominent regulators of stress response. Understanding sigma factor behaviours is important in settings where we wish to kill bacteria (e.g. food decontamination and disease treatment) through the application of some stress (e.g. heat or antibiotics). Every species typically maintain several different types of sigma factors, each governing a specific stress response. Through single-cell fluorescent microscopy studies, it has been noted that sigma factor responses often are highly heterogeneous, even across isogenic populations. It has been suggested that this is a part of a bet-hedging strategy, whereby displaying a varied response, the population can optimally adapt itself to future uncertainty. We have studied two different sigma factor systems in Bacillus subtilis, each displaying a distinct form of response heterogeneity. By combining stochastic mathematical modelling of their regulatory pathways with experimental measurements of their response activities, we explain how the two responses can be generated through the amplification of natural biochemical noise. Next, we note that most sigma factor circuits contain a mixed positive/negative feedback loop. Through a general model of such a circuit, we show how this motif can generate a range of different response behaviours (including both previously observed, and novel, ones). We show how the model’s parameters (i.e. the system’s biological properties) affect which behaviour is produced. This demonstrates a general mechanism to generate phenotypic heterogeneity, which can also be generalised to non-sigma factor systems containing mixed positive/negative feedback loops.
Biography
Torkel Loman is a final year PhD student at the Sainsbury Laboratory, at the University of Cambridge. In his research is uses mathematical models to study sensory pathways in bacteria, while also working in close cooperation with experimentalists. The primary focus is stochastic models and the investigation of how intrinsic cellular noise can be harnessed by the bacteria to create advantageous phenotypes. He is also a codeveloper of the Catalyst.jl chemical reaction network modelling package. Torkel has previously studied at the programs for engineering mathematics, molecular biology, and bioinformatics at Lund University.
Faster Single Cell Analysis
Rasmus Henningsson. Lund University. Sweden
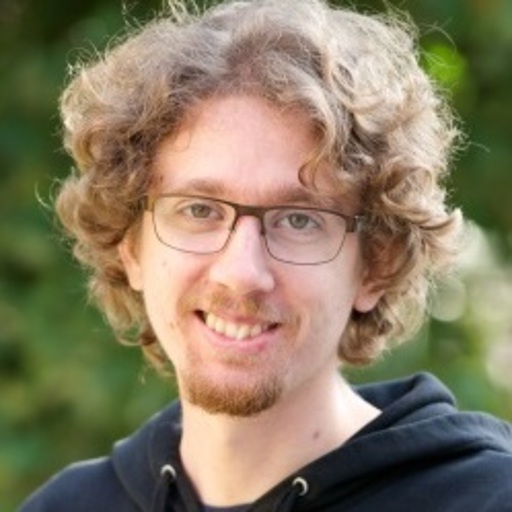
Abstract
Using Single Cell RNA Sequencing, it is today possible to generate data sets with gene expression levels for 30k genes in hundreds of thousands of cells. Explorative analysis of these rich data sets is important – but challenging using existing tools that are slow and require lots of RAM (even if many genes are thrown out). We show that even though the normalized data matrix is not sparse, we can still exploit the sparsity of the original data matrix to vastly reduce RAM usage and runtimes. In particular, we compute the truncated SVD of the normalized data matrix, which serves as noise reduction, dimension reduction and a starting point for downstream analysis.
Biography
Rasmus Henningsson’s research interests are centered around high-dimensional biological data in general and Leukemia in particular. He is currently developing new methods for dimension reduction, analysis and visualization of single cell RNA-seq data. He got his PhD degree in applied mathematics at Lund University in 2018, working on dimension reduction, viral evolution and Leukemia.
May 12, 09:00-09:45
Automatic Control Seminar room (KC 3N27)
Network interactive exploration of a clinical breast cancer cohort
Nadir Sella, Institute Roche, France
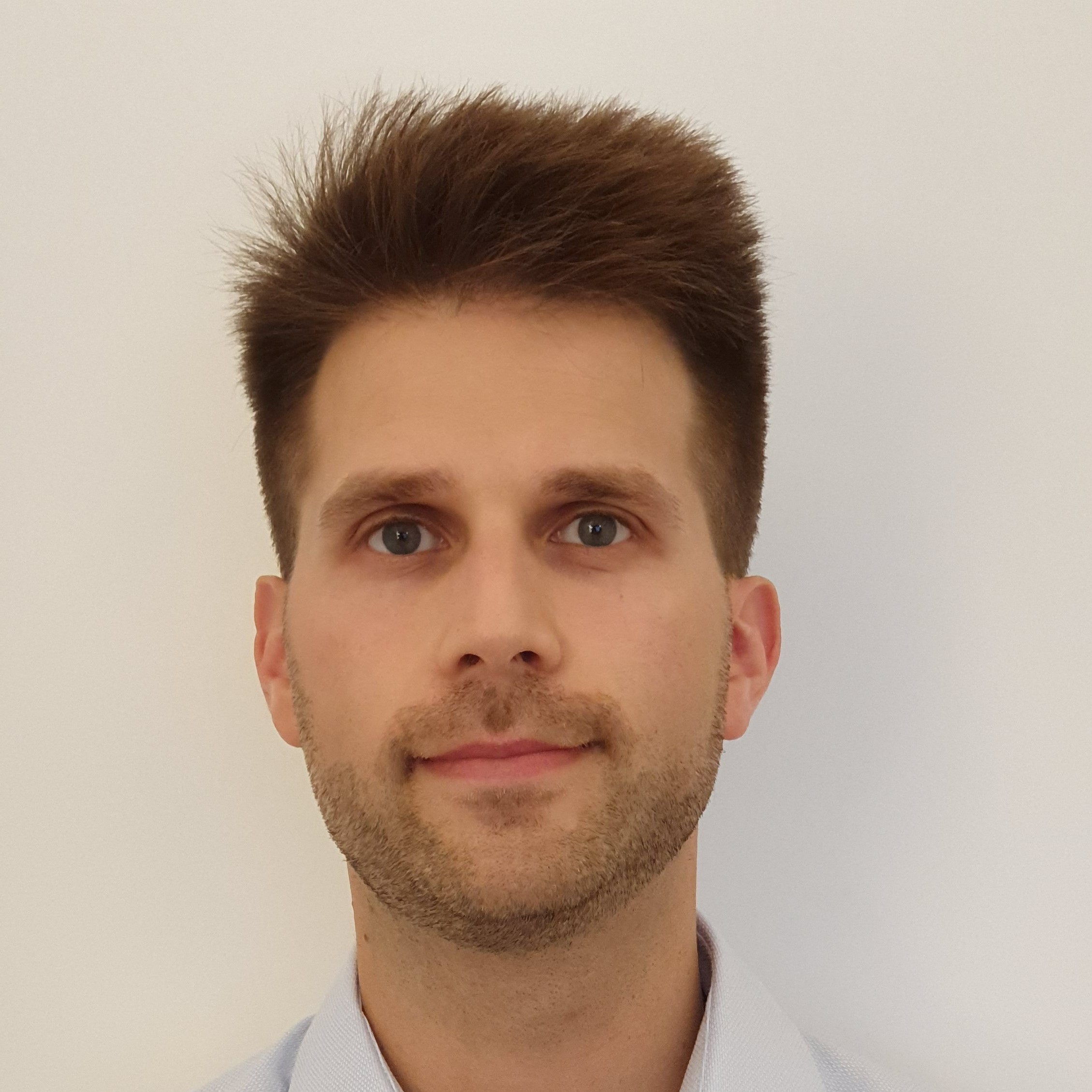
Abstract
With the rapidly increasing amount of information available in health databases, there is a real need for innovative interactive tools specifically designed for the exploration of health data, which remains underexploited, due to data heterogeneity and complexity. We report a novel interactive graphical interface providing the front end of a machine learning inference server (MIIC). MIIC interactive interface facilitates the visualization and comprehension of expected as well as unsuspected relationships between clinically relevant variables. We demonstrate the interest of MIIC interactive interface by exploring the clinical network of a large cohort of breast cancer patients treated with neoadjuvant chemotherapy (NAC).
Biography
Nadir is currently working at Roche Institute in Paris (France) as a postdoctoral researcher, focusing on the analysis of health data in cancer and neurodegenerative diseases. He got his PhD at Curie Institute, developing a new method (MIIC) to infer large networks from observational data, with applications to gene network reconstruction, protein structure, neurodegenerative diseases and cancer cohorts.
After his PhD, Nadir worked as a postdoctoral researcher in Institute Curie analyzing possible causal effects of comedications in response to chemotherapy treatment in breast cancer, with a particular focus on the analysis of large real life cohorts, where by definition randomization between treatment groups is not achieved.
May 12, 10:30 - 12:00
Automatic Control Seminar room (KC 3N27)
Covariate modelling – a tool for individualization of therapy
Jesper Sundell, Lund University, Sweden
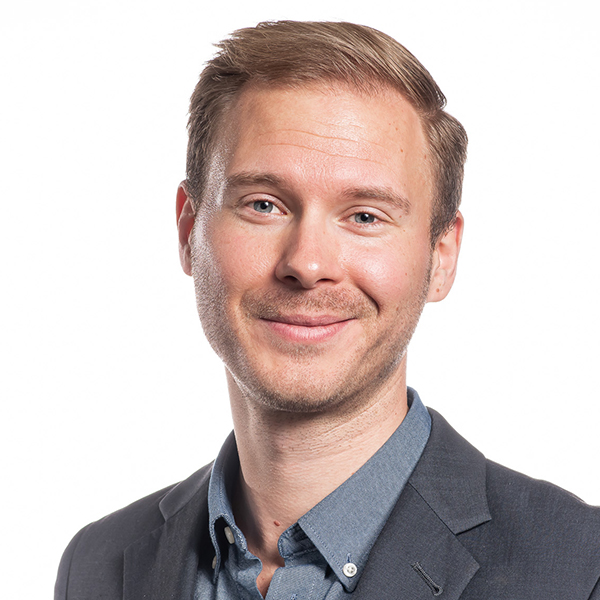
Abstract
Clinical utility of drugs is a balance between efficacy and toxicity. An administered drug dose drives drug exposure in the patient, which, in turn, results in an effect. The pharmacokinetics (PK) and pharmacodynamics (PD) of therapeutics guides the selection of clinical doses in drug development. PK/PD modelling and simulation is commonly applied for data analysis and extrapolation to support the dose selection. However, variability in PK and/or PD parameters between individuals may result in variability in treatment outcome with regard to therapeutic success and toxicity. An approach to identify and quantify sources of variability in PK or PD is covariate modelling. Identification of clinically relevant covariates aids identification of populations at risk of inadequate therapeutic outcome and may be used to guide appropriate modifications to therapy.
Covariate models are components of non-linear mixed effects models, which are typically used to describe the PK and PD of drugs. Covariates include patient specific factors such as weight, creatinine clearance or genetics that improve the prediction of drug concentration or effects (both desired and adverse). This seminar concerns concepts of covariate modelling and its potential in relation to personalized medicine.
Biography
Jesper Sundell holds a M.Sc. in pharmacy received from Gothenburg University. His PhD thesis, which he defended in 2021, focused on how patient specific factors can be used to guide therapy in tuberculosis/HIV patients. His research interests include pharmacometric modelling and individualization of pharmacotherapy.
TCR-induced transcriptional signatures instruct with rapidity and precision thymocyte selection
Jonathan Desponds, Institute Roche, France
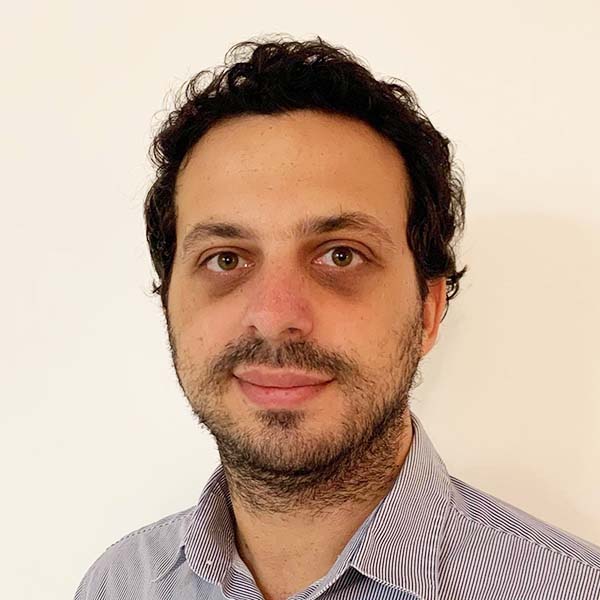
Abstract
The adaptive immune system responds to pathogens by selecting clones of cells with specific receptors. While clonal selection in response to particular antigens has been studied in detail, it is unknown how a lifetime of exposures to many antigens collectively shape the immune repertoire. Using mathematical modeling and statistical analyses of T cell receptor sequencing data, I will describe a quantitative theory of human T cell dynamics compatible with the statistical laws of repertoire organization. I will show that clonal expansions during a perinatal time window leave a long-lasting imprint on the human T cell repertoire, which is only slowly reshaped by fluctuating clonal selection during adult life, with implications for pathogen defense and autoimmunity.
Biography
Trained in mathematics and physics, Jonathan Desponds has been applying quantitative methods to biological and biomedical research since his PhD at ENS in Paris, with applications in the fields of developmental biology, immunology and immuno-oncology. He has worked as postdoctoral scholar at the physics department of UC San Diego and as a fellow at the NSF-Simons center for quantitative biology at Northwestern University. He then joined the Health Data Hub, a French national initiative to gather and link health datasets in the interest of research, as a scientific project manager. Since Octobre 2021, he has been working at Institut Roche, in Paris, to develop and improve cancer immunotherapies.
He has experience applying methods from statistics, probability theory, statistical mechanics and machine learning to inference and modeling of diverse biological processes. Those processes include: transcription regulation, cellular decision-making, selection of adaptive immune cells,spatial organization of developing organisms, population dynamics of immune repertoires and response to cancer immunotherapy.
May 13, 09:00 - 09:45
Automatic Control Seminar room (KC 3N27)
Deep learning for life sciences
Nikolay Oskolkov, Lund University, Sweden
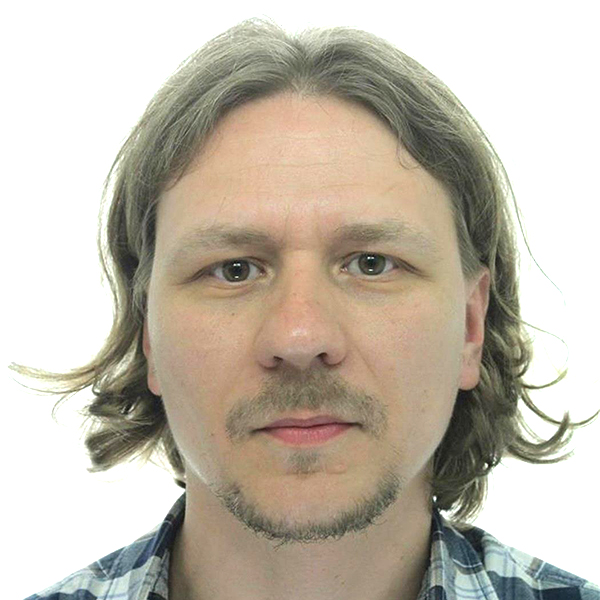
Abstract
In this seminar, I am going to discuss whether we have sufficient amounts of data in Life Sciences for AI applications such as Deep Learning (DL). I am going to give a few concrete examples of using DL in my projects for biological image analysis, single cell omics, ancient and modern genomics as well as microbiome analysis and data integration.
Biography
Nikolay Oskolkov, PhD, is a bioinformatician at Lund University, Biology department, and National Bioinformatics Infrastructure Sweden (NBIS) at Science for Life Laboratory (SciLifeLab). He received his PhD in theoretical physics in 2007, and changed his research focus for bioinformatics and biomedical research in 2012. He worked on different research projects varying from clinical medicine to evolutionary biology and ancient genomics analysis. His research interests involve statistical analysis, machine learning and data science.
May 13, 10:30 - 12:00
Automatic Control Seminar room (KC 3N27)
Pharmacometric covariate modeling using symbolic regression networks
Ylva Wahlquist, Lund University, Sweden
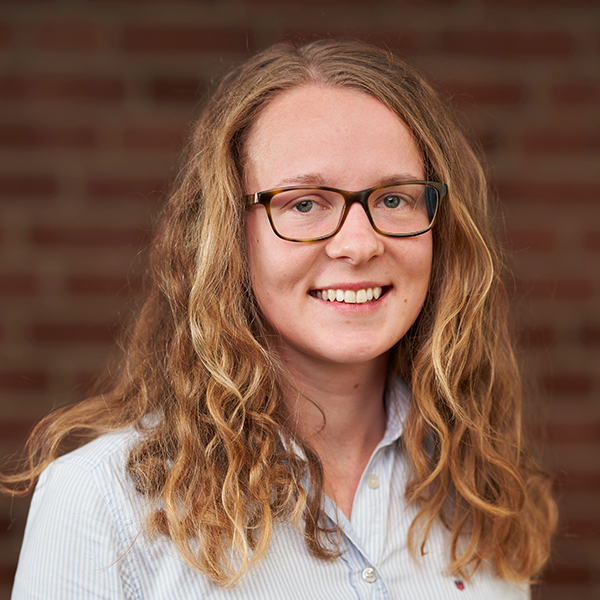
Abstract
A central challenge within pharmacometrics is to establish a relation between pharmacological model parameters, such as compartment volumes and diffusion rate constants, and known population covariates, such as age and body mass. There is rich literature dedicated to the learning of functional mappings from the covariates to the model parameters, once a search class of functions has been determined. However, the state-of-the-art selection of the search class itself is ad hoc. We demonstrate how neural network-based symbolic regression can be used to simultaneously find the function form and its parameters. The method is put in relation to the literature on symbolic regression and equation learning. A conceptual demonstration is provided through examples, as is a road map to full-scale employment to pharmacological data sets, relevant to closed-loop anesthesia.
Biography
Ylva Wahlquist is a PhD student at the Department of Automatic Control since 2020 at Lund University, Sweden. In her research, she studies modeling and control of hemodynamic parameters, particularly within the field of closed-loop anesthesia and heart-lung transplantation. Particularly, she investigates how pharmacometric covariate modeling can be used to minimize the effects of inter-patient variability in the drug response to anesthetics. From January to March 2022, Ylva is visiting a research group at UBC, Vancouver, Canada, where she continues her work on closed-loop anesthesia.
Evaluating Hybrid States of the Epithelial-Mesenchymal Transition in Cancer Metastasis Using Single-Cell RNA-Sequencing
MeiLu McDermott, University of Southern California, USA
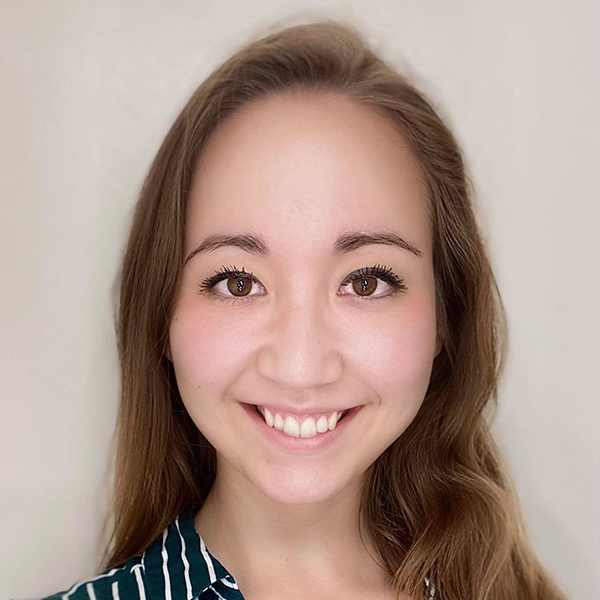
Abstract
The epithelial-mesenchymal transition (EMT) is considered one of the primary mechanisms of cancer metastasis. EMT is a biological process where a cell’s phenotype changes from adherent (epithelial), such as in a primary tumor, to becoming migratory and immune-evasive (mesenchymal), such as a circulating tumor cell. Our research investigates properties of
hybrid-EMT cells, which have characteristics of both the E state and M state.
Recent research has implicated that these hybrid-EMT cells may have the most metastatic potential. We analyze single-cell RNA-seq datasets to determine signature genes in hybrid-EMT cells across multiple cancers. With this scRNAseq data, we create and fit an ODE model that describes rates of transition between cell populations across states of EMT. Using this ODE model, we can discover genes that affect rates of transition between EMT states – comparatively increasing or decreasing the rate of EMT in a certain cancer. This research provides a discovery mechanism for genes across multiple cancers that influence EMT and consequently metastasis.
Biography
MeiLu is a PhD Research Student at Adam MacLean Labat University of Southern California. Works include analysis of experimental single-cell RNAseq data withPythonand R, creation of ODE models to study dynamics of EMT in cancer and hematopoietic stem cell differentiation with Matlab and Julia. MeiLu was a Research Associate at Leo Wang Labat City of Hope (2017-2019), where she created an analysis pipeline for high-throughput mass spectrometry proteomics and phosphoproteomics and performed bioinformatics analysis including gene ontology, pathway analysis with IPA and KEGG, and differential expression analysis with limma.
May 16, 10:30 - 12:00, 13:00-13:45
BMC Segerfalksalen
Deep learning for scoring lung damage on histology images
Salma Kazemi Rashed, Lund University, Sweden
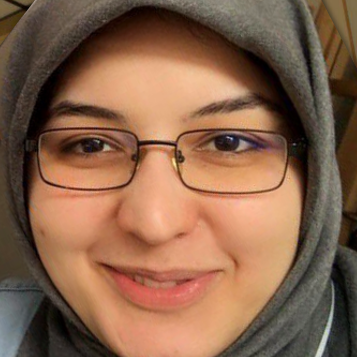
Abstract
Acute respiratory distress syndrome (ARDS) is one of the life-threatening complications in COVID-19 patients for which there is no optimal treatment. Currently, only two methods, mechanical ventilation (MV) and extracorporeal membrane oxygenation (ECMO), are used as treatment but the best way of applying them to minimize lung damage is still being studied. This is often done in a porcine model where the extent of lung damage as well as the effectiveness of the treatment is assessed by carefully examining histology sections of lung tissue. The amount of damage is quantified by scoring various damage features such as the amount of hemorrhage (bleeding), inflammatory cell influx, thickening of the alveolar wall, and others. This scoring is a time-consuming, expensive, and error-prone task, and inconsistency between different scorers is a common and serious problem. To overcome these shortcomings, we have used deep learning tools, specifically convolutional neural networks trained with transfer learning. First, we trained a binary classifier which accurately separated healthy from damaged tissue. This was then refined into a 3-class classifier which distinguished between high, medium, and low damage. Finally, a series of regression models was trained for scoring seven features that reflect the extent of lung damage. The trained regression models show a correlation of up to 0.9 with the ground truth and almost 80% accuracy. This was similar to or better than the performance of human researchers.
Rashed, S.K.1, Silva, I.A.N.2,3,4, Wagner, D.E.2,3,4 & Aits, S.1,5.
1 Cell Death, Lysosomes and Artificial Intelligence group, Department of Experimental Medical Science, Faculty of Medicine, Lund University, Lund, Sweden;
2 Lung Bioengineering and Regeneration (LBR), Department of Experimental Medical Sciences, Lund University, Lund, Sweden;
3 Wallenberg Center for Molecular Medicine, Lund University, Lund, Sweden;
4 Stem Cell Center, Lund University, Lund, Sweden;
5 Lund Institute of Advanced Neutron and X-ray Science, Lund, Sweden.
Biography
Salma Kazemi Rashed received a B.Sc. degree in Electrical engineering, communications from the University of Tehran in 2014 and a M.Sc. degree in Telecommunications engineering in 2017 (also in Tehran). She then spent four years from 2014–2018 as a Research Assistant with a main research focus on interactive machine learning and distributed algorithms and their applications in cellular networks. Her interest in machine learning and its many applications in biomedicine prompted her to enter the field of bioinformatics. She received her second M.Sc. degree in bioinformatics from Lund University, Lund, Sweden, in 2019. She is now a second-year PhD student in the Cell death, Lysosome and Artificial Intelligence group at the Department of Experimental Medical Science at Lund University. Her research focuses on deep learning applications in biomedical science with the main focus on lysosome-dependent cell death, environmental toxins and COVID19. She primarily works on computer vision for microscopy and histology images, natural language processing and genetic data analysis.
Spatial correlation for study of tumour tissue organisation with spatial transcriptomics
Lara Jerman, Centre de Recherche des Cordeliers, France
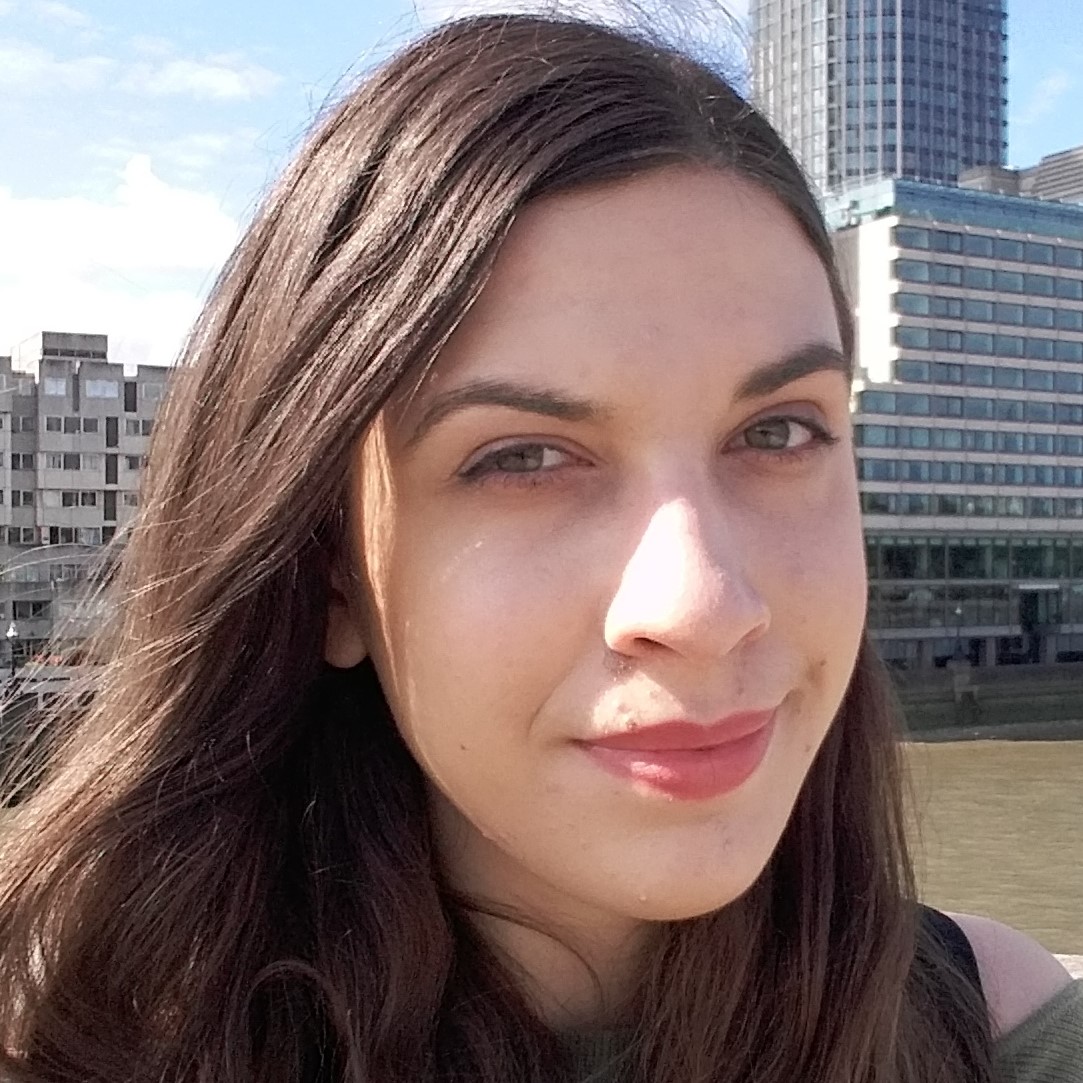
Abstract
Biological tissue is composed of an array of interacting cell populations and typically shows a precise spatial organisation, directly related to its function. This organisation is regulated by complex mechanisms, notably physical and molecular signals. The spatial gradients of some proteins, for example, are known to be essential for the spatial patterning of particular tissues. A powerful way to study such spatial organisation of, in our case, tumour tissue, is by spatial transcriptomics.
The Visium technology is able to provide us with thousands of pixel-level (typically aggregating between 1 and 10 cells) gene expression profiles, for which coordinates on the corresponding tissue section are also known. This data enables us to measure the spatial distribution of gene expression and furthermore – through cell type deconvolution – also allows us to study the spatial distribution of cell types. We can leverage this information to identify multicellular spatial
structures (e.g. tertiary lymphoid structures). Identifying these is essential to obtain an in-depth understanding of the tumour microenvironment spatial organisation and related cellular dialogues.
For effective study and comparison of these spatial distributions and patterns, we need concepts such as spatial correlation and autocorrelation. These have been previously introduced and addressed in other contexts and fields, such as ecology and geography. In this seminar, we will review the ways they have been used the context of spatial transcriptomics and elsewhere, as well as address the specific technological concerns.
Biography
Lara Jerman is starting her PhD studies in 2022 at Sorbonne University in co-supervision between the Cordeliers Research Center and Institut Roche in Paris. She graduated from the University of Ljubljana, obtaining a bachelor’s degree in biochemistry in 2017 and a master’s degree in mathematics and computer science in 2020. During her studies, she carried out a research visit to the European Bioinformatics Institute (EMBL-EBI), and later visited the University of Cambridge as a Junior Fellow of the American Slovenian Education Foundation. She also spent a year in industry research as a data scientist working on cancer drug biomarker development at Genialis.
Her PhD project focuses on studying the relationship between intra-tumoral heterogeneity and local immune contexture of lung cancers through spatial transcriptomics.
Getting personal with epigenetics: towards machine-learning-assisted precision epigenomics
Giovanni Visona, Max Planck Institute for Intelligent Systems Tübingen, Germany
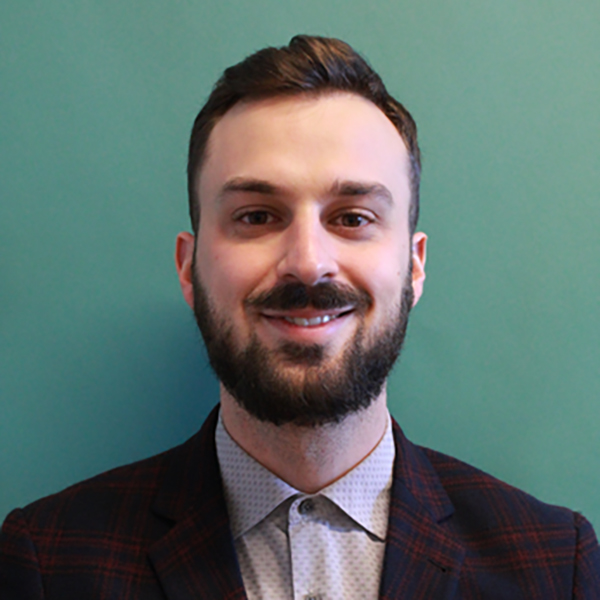
Abstract
Epigenetic modifications are dynamic control mechanisms involved in the regulation of gene expression. Unlike the DNA sequence itself, they vary not only between individuals but also between different cell types of the same individual. Exposure to environmental factors, somatic mutations, and ageing contribute to epigenomic changes over time, which may constitute early hallmarks or causal factors of disease. Epigenetic changes are reversible and, therefore, promising therapeutic targets. However, mapping efforts to determine an individual’s cell-type-specific epigenome are constrained by experimental costs. We developed eDICE, an attention-based deep learning model, to impute epigenomic tracks. In addition to benchmarking results against state of the art models, I will present a proof of concept for the imputation of personalised epigenomic measurements on the ENTEx dataset, where eDICE correctly predicts individual- and cell-type-specific epigenetic patterns.
Biography
Giovanni Visona graduated in medical physics at the University of Trent (Italy), and moved to machine learning with an MSc at the University of Edinburgh. Giovanni joined the Max-Planck Institute for Intelligent Systems in Tübingen at the end of 2019 for a PhD, and is now part of the Marie Curie Innovative Training Network entitled “Machine Learning Frontiers in Precision Medicine”. In his work, Giovanni focus on the application of deep learning and representation learning models to biomedical data, with a particular interest for sequencing data.
May 18, 10:30 - 12:00
Automatic Control Seminar room (KC 3N27)
Immune infiltration diversity for precision oncology
Meghan Ferrall-Fairbanks, University of Florida, USA
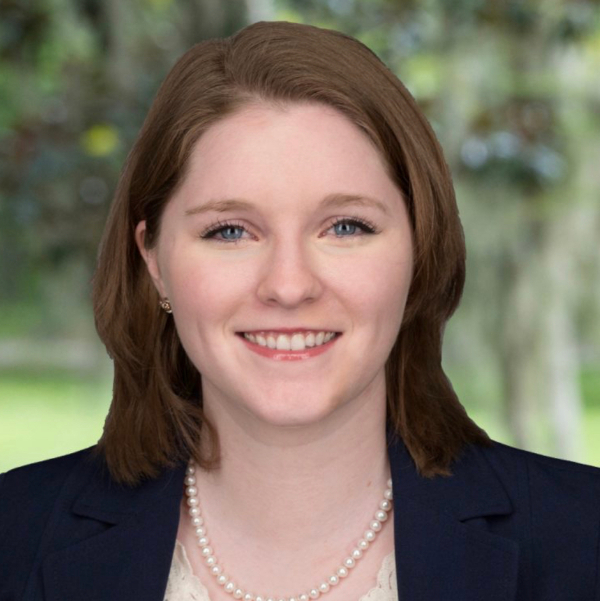
Abstract
Modern immunotherapy agents function to kill tumors by releasing the host (patient’s) immune system from inhibitory stimuli. The arrival of immune checkpoint inhibitors (ICIs) has precipitated a tremendous research effort aiming to accurately characterize the tumor-immune microenvironment (TIME) and explore potential biomarkers to predict ICI response, for which robust markers have been largely elusive. To initiate an anti-tumor immune response, tumor-specific neoantigens must first be recognized by a receptor on an infiltrating lymphocyte. The complementarity determining region-3 (CDR3) is a highly variable region in the receptor that provides a complementary binding surface for antigens and largely determines the antigen specificity. To better understand the tumor ecology of this TIME, we quantified tumor infiltration across three distinct clear cell renal cell carcinoma (ccRCC) patient tumor cohorts using CDR3 sequence recovery counts from tumor-infiltrating lymphocytes and a generalized diversity index (GDI) for CDR3 sequence distributions. GDI comes from ecology and can be understood as a curve over a continuum of diversity scales that allows nuanced characterization of sequence distributions and captures richness, evenness, and subsampling uncertainty, along with other important metrics of immune cell clonality. In ccRCC tumors, we found significant clinicopathologic associations in patients with larger and more aggressive tumors having increased richness of recovered tumoral CDR3 sequences, specifically in those from T-cell
receptor alpha and B-cell immunoglobulin lambda light chain. Furthermore, even in a white, male-dominant cancer type, point-estimates of GDI revealed significantly increased CDR3 diversity in non-white and female patients, suggesting that these patients have genetic variances in their immune receptor repertoire and therefore may respond differently to immunotherapies.
This study brought together researchers with backgrounds in applied mathematics, biomedical engineering, immunology, data science, oncology, pathology, as well as surgery, to show that different GDI point estimates that emerge from a spectrum of scales of heterogeneity, can reveal unique information about tumors and patients and identified a new approach to help stratify patient-based differences in immune infiltration diversity and further guide precision oncology.
Biography
Meghan Ferrall-Fairbanks, Ph.D. is an Assistant Professor of Biomedical Engineering in the J. Crayton Pruitt Family Department of Biomedical Engineering at the University of Florida (UF). Dr. Ferrall-Fairbanks received her Bachelor of Science degree in Mechanical Engineering with a biomechanics minor from UF in 2012. She earned her Ph.D. in the joint Department of Biomedical Engineering at Georgia Tech and Emory University in 2017. Her dissertation research focused on integrating wet-lab experiments and computational methods to tease apart complex enzyme-on-enzyme interactions in proteolytic networks up-regulated in tissue destructive diseases. Following her time in Atlanta, Dr. Ferrall-Fairbanks was an Applied Postdoctoral Fellow in the Department of Integrated Mathematical Oncology at Moffitt Cancer Center and Research Institute. Her research at Moffitt focused on using mathematical modeling to study single-cell heterogeneity and clonal dynamics in cancer; while there she worked on multi-disciplinary projects, with modelers, basic scientists, and clinicians addressing top questions in cancer research. Dr. Ferrall-Fairbanks’ current research focuses on using quantitative biology approaches to cultivate a mechanistic understanding of tumor heterogeneity and evolution to optimize cancer treatment strategies. Ecology and evolutionary forces in cancer allows a tumor to alter its growth and metastasis. The Battling Evolution through Adaptive Therapies (BEAT) Cancer Lab integrates computational, mathematical, and wet-lab experimental techniques to investigate tumor heterogeneity at the molecular, tissue, and systems levels.
Julia for Quantitative Systems Biology
Elisabeth Roesch, University of Melbourne, Australia
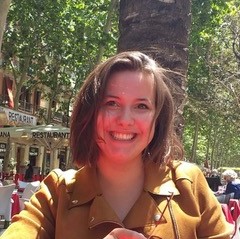
Abstract
Background/objectives
Quantitative Systems Pharmacology (QSP) is a field of growing importance. The use of sophisticated QSP models is becoming more and more essential within the pharmaceutical industries. QSP models help optimise the efficiency and effectiveness of processes in drug discovery as well as drug development and hence enable scientists to safe valuable time during critical stages of their work. In QSP, we often encounter non-trivial problems: systems are large (biological system of interest), models are complex (mathematical concepts) and data is big (computational approaches). This combination creates interdisciplinary, hard problems to describe and solve. Having the right tools to address them is important: from a software perspective, this translates in the need for a programming language with simple and clear syntax and high performance — like the Julia Language.
Methods and Results
Here, we present the programming language Julia. We describe why we believe Julia is the ideal language for QSP. First, we focus on the three main features of Julias language design: 1. speed, 2. abstraction and 3. metaprogramming. We explain what they are, why they are important and how they translate in ease of use and performance advances for users, specifically relevant to scientists in QSP. Second, we describe Julia’s rich package ecosystem. We highlight packages providing functionalities and methodologies relevant for QSP as well as domain specific packages. Third, we provide further insights into the adaption process of Julia. We provide an overview of helpful material on getting started with Julia and moving projects from other languages to Julia. Last, we describe additional tools and products that build on top of the programming language Julia, which are especially helpful for scaling projects of industry size (JC products).
Discussion and Conclusion
In QSP, we often describe large biological systems with complex mathematical models and work with big data. From a software perspective, this combination can create problems that are difficult to describe and solve. Programming languages with accessible syntactic descriptions (such as R, Python or MATLAB) are often the preferred choice in the life science community. However, they are not able to deliver the performance needed in sophisticated QSP studies. Using a second programming languages (such as C/C++ or Fortran) under the hood, or in a second, fast version of the code after the high effort of translating whole algorithms and projects, is not an uncommon solution for solving performance issues. We believe users should not have to use two languages or choose between ease-of-use and high performance. Julia is built to be easy to program in and fast — and hence ideal for QSP.
Biography
Elisabeth Roesch is a systems biologist by training. She is interested in modelling life science systems using computer science, mathematics and statistics. Currently, she works with Julia Computing. Here, she focuses on bridging the interests of pharmaceutical clients and internal product designers and software engineers. She helps clients realise and unlock the full potential of Julia and Julia Computing products for their individual projects.
Prior to this, Elisabeth undertook her graduate studies at Ludwig Maximilian University Munich and Technical University Munich (BSc), and her postgraduate studies at Imperial College London (MSc) and University of Melbourne (PhD – under examination). In her research, she explores hybrid models in systems biology: she merges mechanistic and machine learning approaches in one model and she uses them in the setting of decision making processes in developmental biology.