Wednesday, September 25
10:15 - 11:00
A message passing perspective of data assimilation
So Takao, Postdoctoral scholar at the California Institute of Technology (USA)
Abstract
Data assimilation is a key tool in various scientific and engineering disciplines, where the goal is to improve our prediction of a dynamically evolving state from noisy, incomplete observations. In many realistic situations, this procedure can be challenging, requiring us to make crude approximations to the inference method when the dynamical system is nonlinear or high dimensional. On the other hand, approximate inference methods based on local message passing has been developed in the field of statistical machine learning that allows inference to be made at scale and furthermore amenable to modern parallel computing paradigm. In this talk, I will discuss a few directions on how message passing algorithms can help to enhance data assimilation systems from both the perspective of variational inference and scaling up to high dimensions.
Biography
So Takao is a postdoctoral scholar at the California Institute of Technology, working on the intersection of machine learning and data assimilation. His interests involve (1) developing efficient inference techniques for probabilistic models such as Gaussian processes and stochastic partial differential equations, (2) developing machine learning models with physical, geometric or topological inductive biases, and (3) applying machine learning techniques to problems in weather or climate science. During 2021-2023, he was a Senior Research Fellow in Machine Learning for Climate Science at the UCL Sustainability and Machine Learning group. There, he lead the Met Office Academic Partnership workgroup on “Data science methodology for weather and climate” and intiated various collaborations across departments to work on problems related to climate change. He received his PhD in 2020 from the Department of Mathematics at Imperial College London, where he wrote his thesis on structure-preserving fluid models and MCMC techniques on Lie groups, advised by Prof. Darryl Holm.
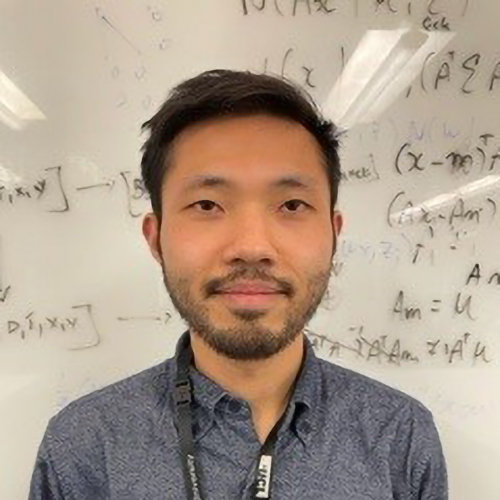
11:15 - 12:00
The best of two worlds? Combining traditional dynamic models with Machine Learning models
Adrian Gustafson, Postdoctoral Researcher at Lund University (Sweden)
Abstract
For a long time, dynamic numerical models with a strong theoretical foundation have been the basis for predictions of future states of for instance climate, forests or carbon stores. These models have been developed over decades and have been thoroughly tested. Nonetheless, this means that they may also include built-in biases and structural uncertainties of core parts that were developed as data for development and parameterisation was limited. New machine learning (ML) techniques have quickly become more popular due to increased computing power to train them and through frameworks simplifying their development. While the new ML models require large datasets for training, they present new opportunities for representing the data without the need for explicit programming. Furthermore, their development and testing can be done outside of the larger numerical frameworks which promote modularity and testability.
In this talk, I will discuss experiences from a project combining the two approaches. Within this project, I have replaced mortality parameterisations within one large-scale numerical framework for simulating global terrestrial ecosystems with a Deep Neural Net trained on forest inventory data. I will discuss both the opportunities and challenges of these hybrid models.
Biography
Adrian Gustafson is a Researcher at the Department of Physical Geography and Ecosystem Science at Lund University. He gained his BSc in Conservation biology from Göteborg University. However, his interest in large spatial patterns and modelling drove him to study Physical Geography and Ecosystem Science as an MSc. He later finished his PhD in Environmental Science at Lund University in 2022. In the PhD thesis, Adrian worked with large-scale dynamic ecosystem models, and coupled vegetation-climate models applied over the Arctic. Since then he has worked on merging dynamic and machine-learning models into hybrid models for improved predictions of tree mortality with subsequent effects on carbon budgets and forest structure.
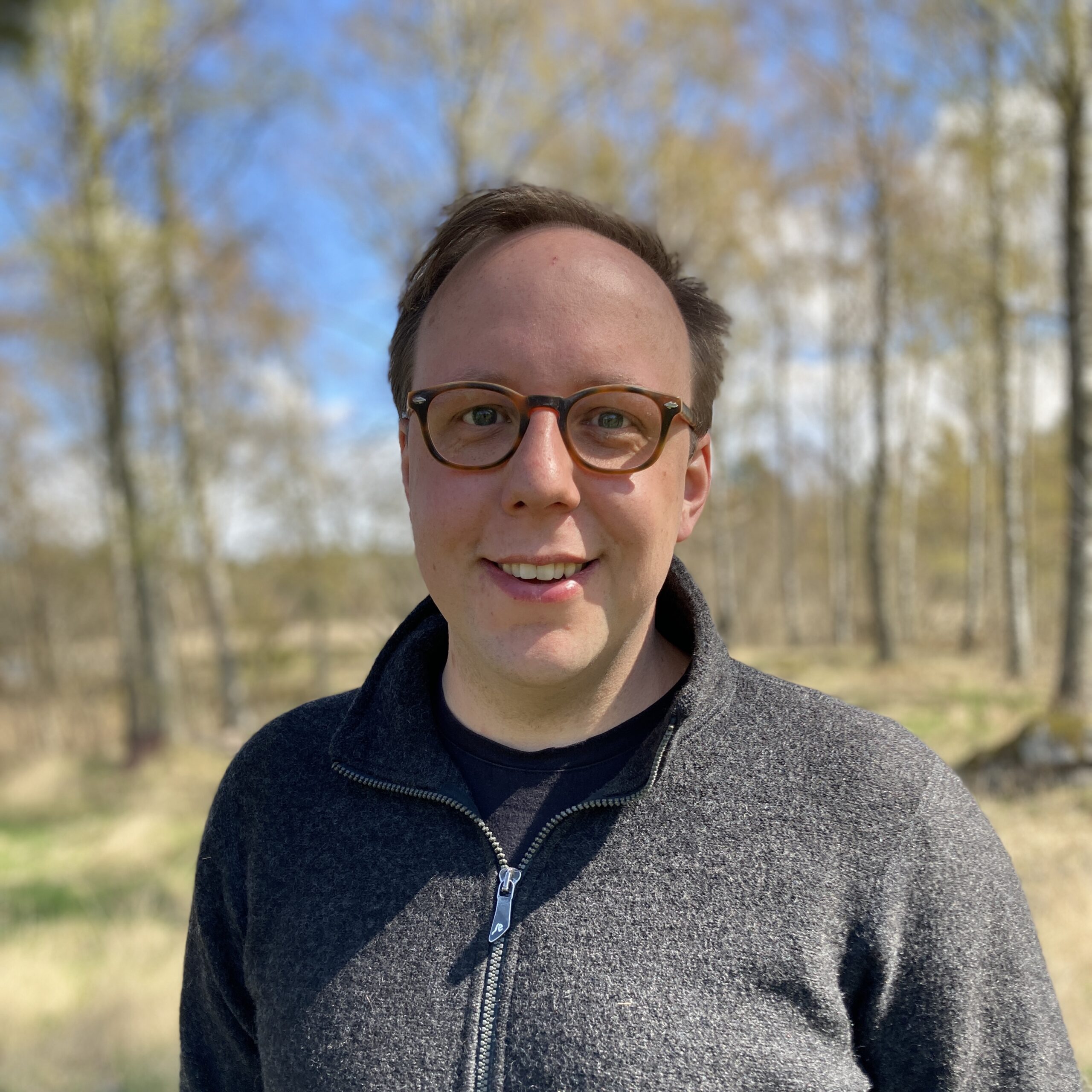
Thursday, September 26
10:15 - 11:00
Unraveling the Dynamics of European Heatwaves: Climate Drivers, Quantile Regression, and Causal Machine Learning Approaches
Deborah Bassotto, PhD Student at University of Valencia (Spain)
Abstract
Heatwaves are becoming more frequent and intense across Europe, driven by climate change. In these ongoing projects, we aim to improve the detection and understanding of the key dynamical and thermodynamical drivers behind these events. We first examine how the drivers of heatwaves shift under different climate scenarios by comparing present and future conditions using CMIP6 models. This analysis provides insights into the evolving role of climate change in shaping heatwave dynamics. Next, we explore the application of Quantile Regression (QR) with pinball loss to model extreme events. The underlying idea is that QR should allow us to capture the full distribution of heatwave predictors and offers a flexible, data-driven approach to identifying emerging patterns in heatwave behavior. Finally, we discuss potential ways to integrate causal machine learning that could enable a deeper understanding of the complex interactions driving heatwaves/cascading effects and improve the accuracy of future predictions.
Biography
Deborah Bassotto has a keen interest in various geoscience topics, particularly when explored through the lens of machine learning. Her research interests span atmospheric and oceanographic physics, ecology, as well as climate informatics and causality. Currently, she is based at the University of València in Spain, where she’s delving into the complexities of predicting and attributing climate extremes.
Her educational background includes a Bachelor’s degree in Geoscience and Environment (Université de Lausanne, Switzerland), followed by a Master’s degree in Climate Physics (Utrecht University, the Netherlands). Subsequently, Bassotto undertook an internship at Université de Lausanne, where she explored the drivers of heatwaves in Europe across historical and future models. Before this, she gained valuable experience in geochemistry at Utrecht University and contributed to research on microplastics in the Black Sea during her time in Italy.
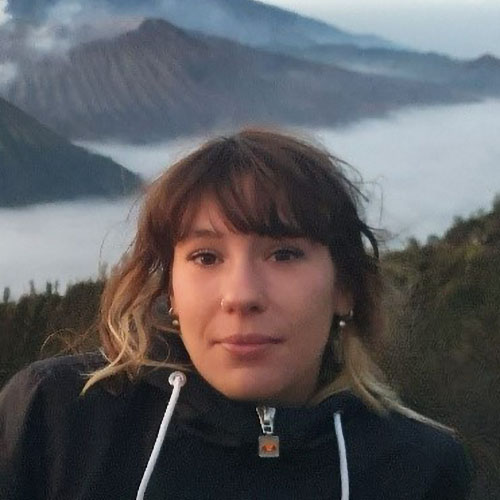
11:15 - 12:00
DETEX, a machine learning-based extreme event emulator
Ramón Franco Fuentes, Research scientist at SMHI (Sweden)
Abstract
We have developed an ML-based algorithm (DETEX) to detect from dynamical and thermodynamical variables at coarse resolution (GCM) where and when an extreme precipitation event will occur at a higher resolution (RCM).
DETEX uses as predictors specifically the zonal and meridional components of the wind (ua, va), the air temperature (ta) and the specific humidity (hus) at 1000hPa, 850hPa, 700hPa, 500hPa and 250hPa atmospheric levels. Furthermore single levels of cloud cover, precipitation, soil moisture and sea level pressure from EC-Earth3-Veg. All these variables were interpolated to a 1-degree resolution.
DETEX predicts extreme precipitation events’ time and spatial location exceeding the overall percentile 99th at 0.1-degree resolution. This implies that the algorithm increases the resolution ten times regarding the resolution of the input data.
The accuracy of two DETEX models, one autoencoder and one purely convolutional is assessed by comparing several evaluation indices for dichotomous predictions.
Biography
Ramón is a climate researcher at the Swedish Meteorological and Hydrological Institute since 2016. Before, his current position he was a postdoctoral fellow at the International Centre for Theoretical Physics in Trieste, Italy where he carried out several regional and global studies using mainly the Regional Climate Model RegCM. He did his PhD at the Ensenada Center for Advanced Studies and Higher Education in Baja California, Mexico. His current lines of research are related to global climate variability and change, particularly on how global modes of variability impact the regional climate. Lately, he has started to work on implementing machine learning methods to climate science, particularly focusing on applying and developing ML methods for downscaling coarse resolution global model data to high resolution, which provides more detailed climate information for regional studies. He is also working on algorithms to detect extreme events from climate variables, using as predictors the current and previous states of the atmosphere. He is currently leading two work packages in the Horizon projects OptimESM and AI4PEX, applying ML methods for climate studies.
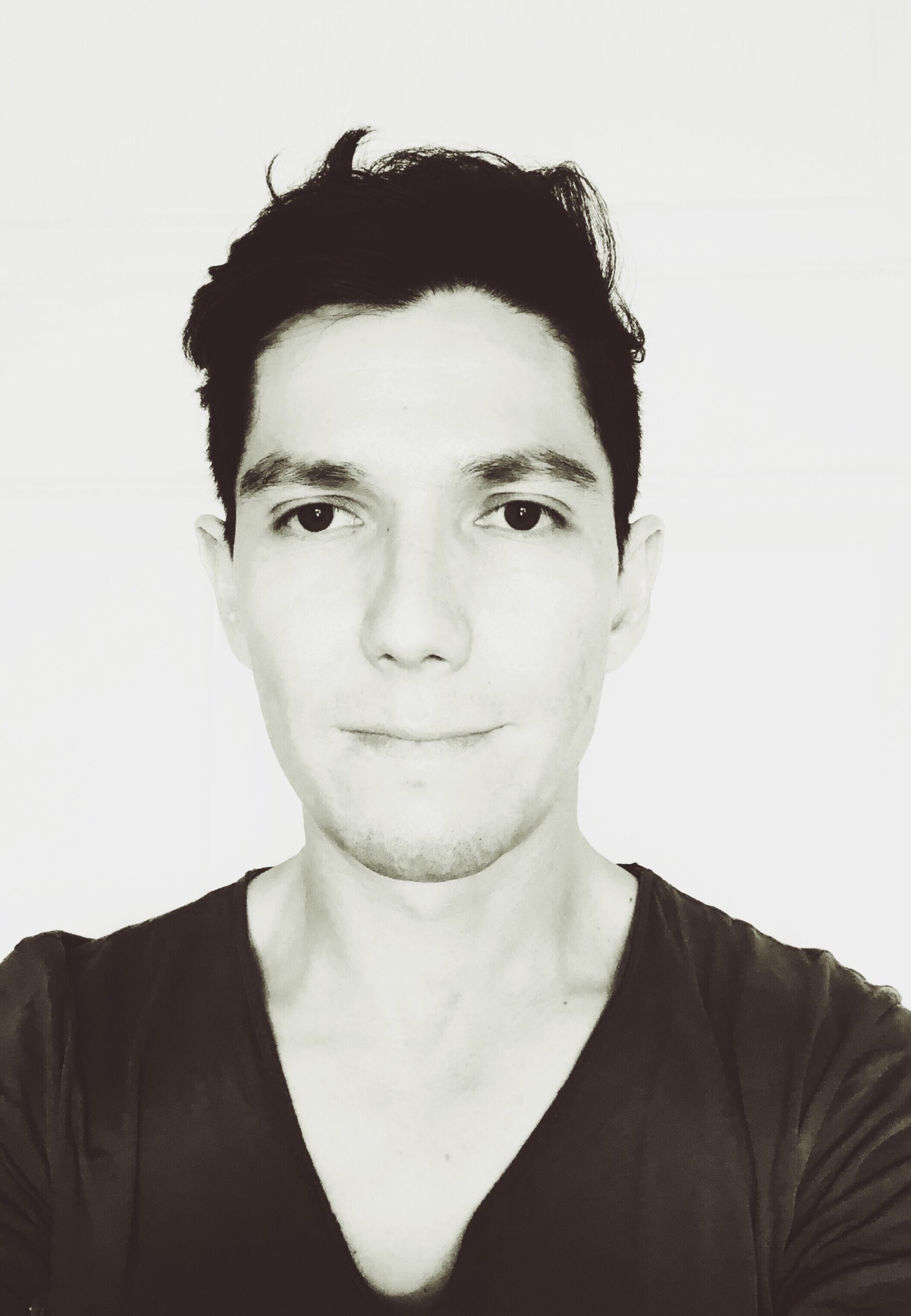
Monday, September 30
Conference Room, Visionen
10:15 - 11:00
Simulating the seasonal variation of rainfall in East Africa and its potential future change in the CORDEX-CORE experiment
Wilhelm May, Researcher at Lund University (Sweden)
Abstract
Agriculture in East Africa is generally rain-fed and, thus, depends on the occurrence of precipitation. Given its location around the equator, the occurrence of precipitation in East Africa is characterized by marked seasonality with either one rainy season centred around local summer in the regions that are further away from the equator (except for the Horn of Africa) and two rainy seasons in the regions near the equator and at the Horn of Africa in boreal autumn and boreal spring.
In the first part of this study, the seasonal variation of rainfall in East Africa is described for observations of daily precipitation and the representation of the seasonal variation of rainfall in a set of regional climate simulations from the CORDEX-CORE experiment for Africa is assessed. These are simulations with three different regional climate models, either forced with observed lateral meteorological boundary conditions or with data from global climate simulations with three different models. The realistic representation of the seasonal variation of rainfall in East Africa in the regional climate simulations is crucial for the validity of future climate scenarios simulated by the respective models and, thus, the potential future impacts on agriculture in East Africa.
In the second part, the potential future changes in the seasonal variation of precipitation in East Africa are assessed from the CORDEX-CORE experiment for the RCP8.5 scenario, focusing on the combinations of regional climate models and driving global climate models that represent the seasonal variation of precipitation in East Africa realistically.
Biography
As a climate scientist, Wilhelm May has been working on various aspects of climate variability and change, including the Indian summer monsoon or heavy precipitation events. He is especially interested in the coupling between the land surface and the atmosphere. He has also worked with adaptation to climate variability and change in Zambia and Western Africa. Currently, he is also working on the role of agricultural systems (Push-Pull technology) for mitigating climate variability and change in East Africa as well as on the effects of forest policies in the Nordic countries for the carbon stocks and the biodiversity in forest ecosystems. In his scientific work he use both various types of observational data and simulations with climate models as well as simulations with a terrestrial ecosystem model recently.
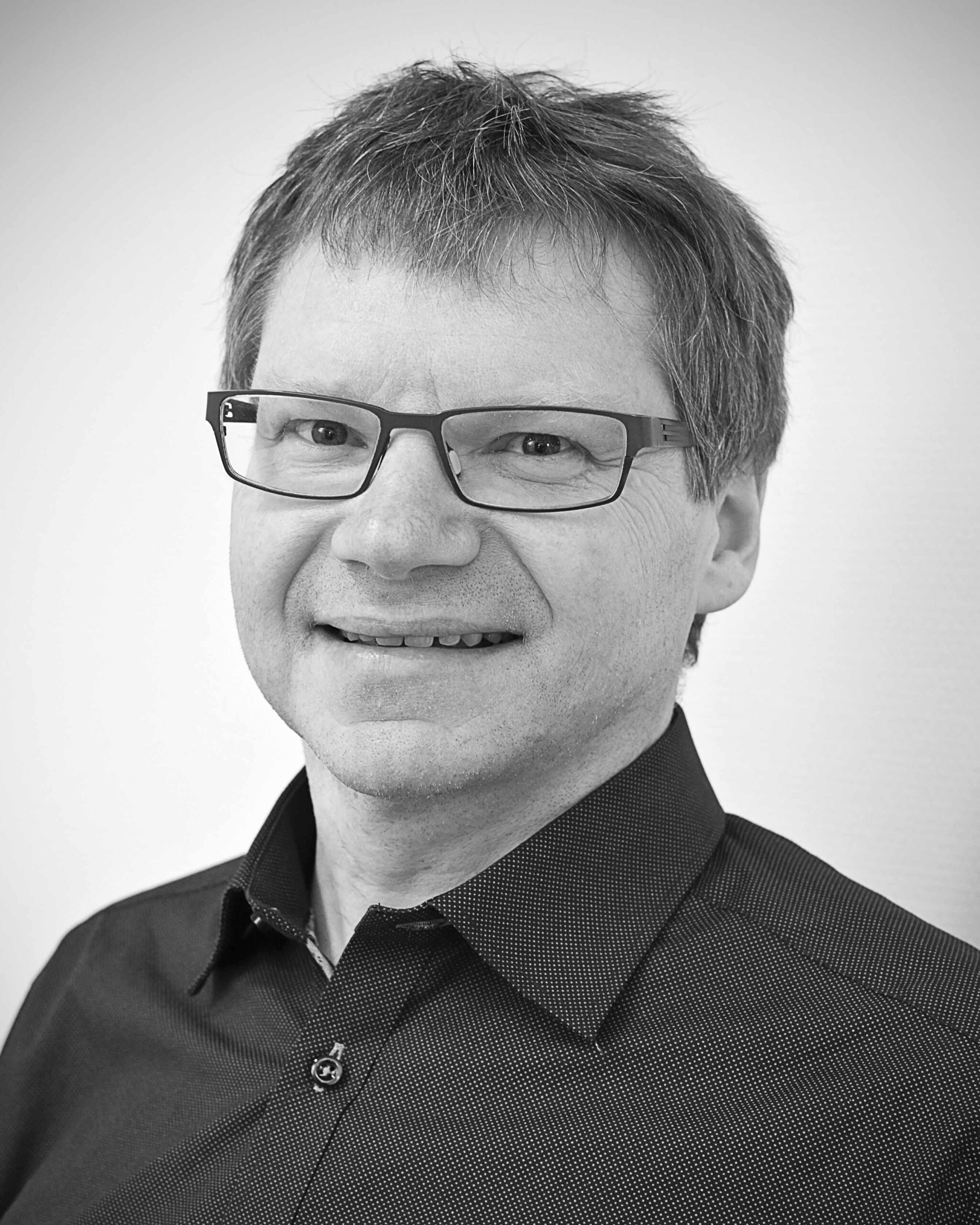
11:15 - 12:00
Can data-driven models beat physics-based numerical models in forecasting weather extremes?
Leonardo Olivetti, PhD student at Uppsala University (Sweden)
Abstract
In recent years, data-driven weather forecast models have shown promising advancements, often surpassing traditional physics-based numerical models in various standard performance metrics. However, recent studies question the ability of data-driven models to provide reliable forecasts of extreme weather events.
In this talk, I will address this concern by first outlining the theoretical limitations of global data-driven models concerning extreme events, and then evaluating the performance of leading deep learning models in practice. Specifically, we will examine how these models compare to physics-based counterparts in forecasting near-surface temperature and windspeed extremes on a global scale.
Our findings suggest that data-driven models can compete with physics-based models even in terms of extreme weather forecasts. However, the choice of best model for extreme events appears to be strongly influenced by factors such as geographical region, type of extreme event, and lead time.
Associated preprint: https://egusphere.copernicus.org/preprints/2024/egusphere-2024-1042/
DOI: https://egusphere.copernicus.org/preprints/2024/egusphere-2024-1042/
Biography
Leonardo Olivetti is a PhD student in the Department of Earth Sciences at Uppsala University. He is a member of the CENAE (Compound Climate Extremes in North America and Europe: from Dynamics to Predictability) research group, led by Gabriele Messori. Leonardo’s research focuses on assessing and enhancing the predictability of near-surface weather extremes through data-driven methods. His current work involves evaluating the effectiveness of end-to-end AI-based approaches in accurately forecasting windstorms and cold spells.
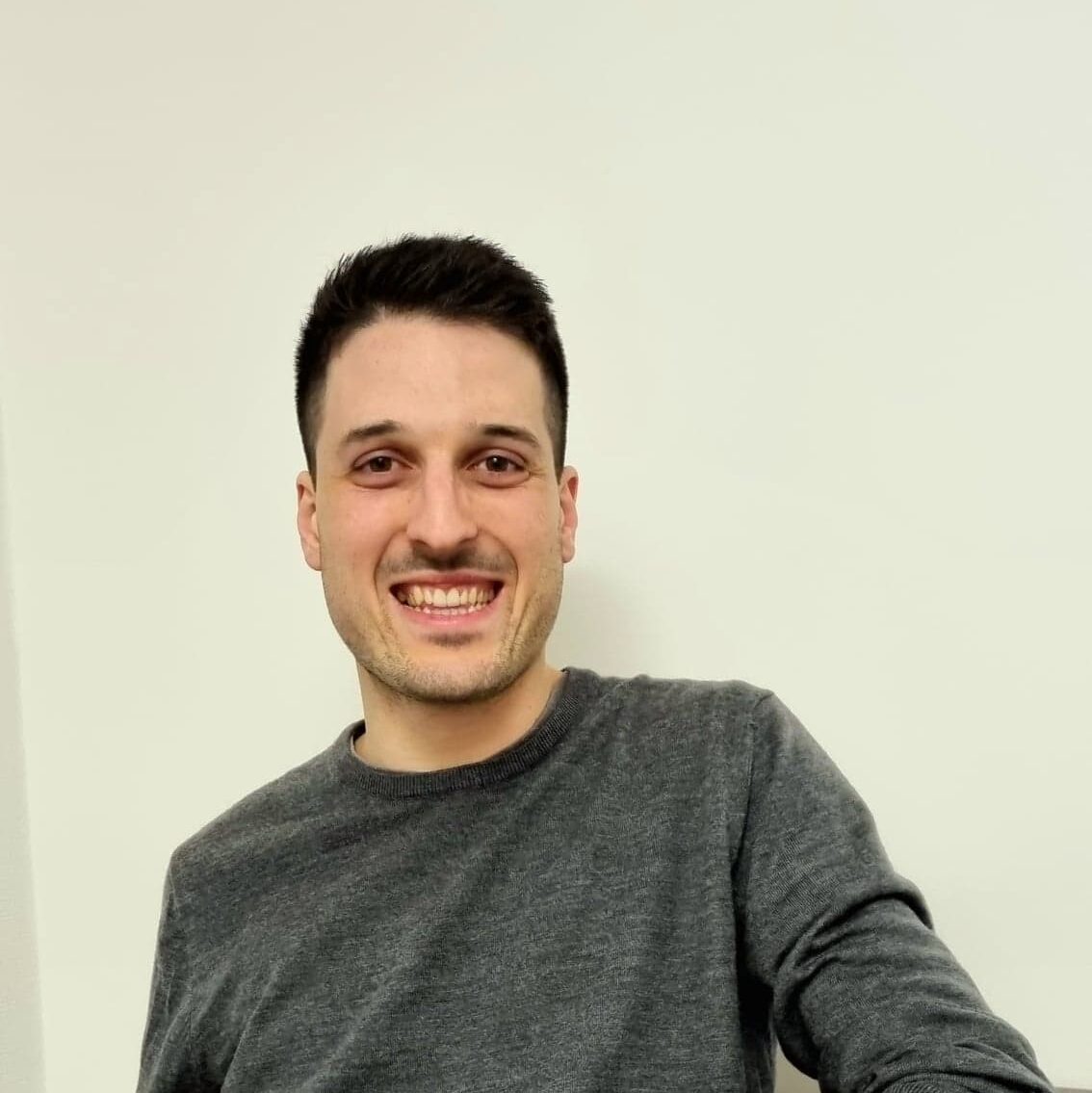
Wednesday, October 2
Conference Room, Visionen
10:15 - 11:00
Visual Analytics Pipeline to Improve Impact-Based Weather Warning Systems
Carlo Navarra, Research Engineer at Linköping University (Sweden)
Abstract
Extreme weather events, such as flooding, are expected to increase in frequency and intensity. Therefore, the prediction of extreme weather events, assessment of their local impacts in urban environments, and implementation of adaptation measures are becoming high-priority challenges for local, regional, and national agencies and authorities. This presentation features a visual analytics (VA) pipeline for the identification and exploration of extreme weather events and their impacts from volunteered geographic information texts and images retrieved from social media. The VA pipeline incorporates three main steps: (1) data collection, (2) image/text classification and analysis, and (3) visualization and exploration through an interactive visual interface.
Biography
Carlo Navarra is a research engineer at the Department of Thematic Studies – Environmental Change and the Centre for Climate Science and Policy Research at Linköping University. His research interests include Information and Geographical Visualization, and Visual Analytics.
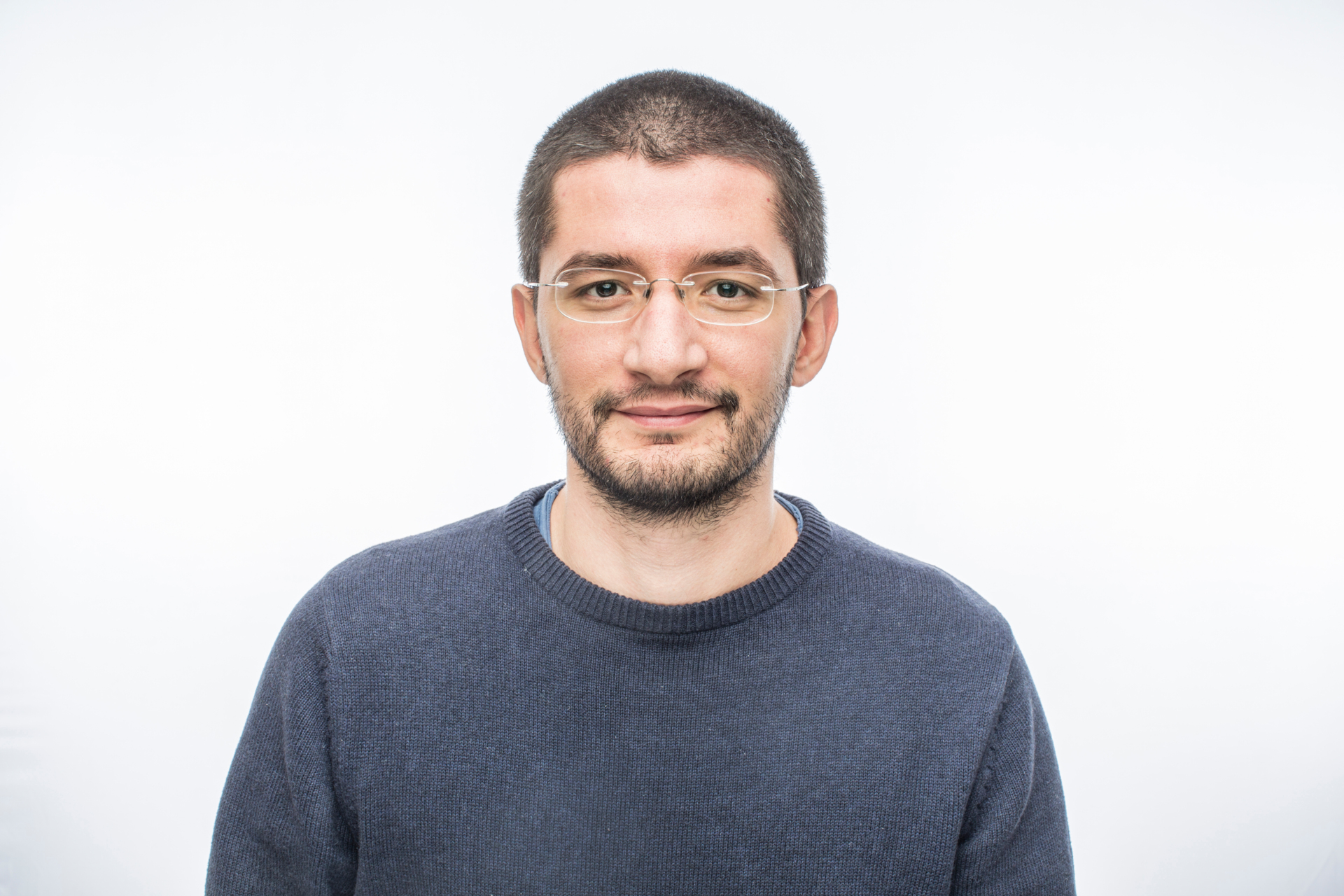
11:15 - 12:00
Machine learning emulation of forest carbon dynamics: an LPJ-GUESS application
Carolina Natel de Moura, Postdoctoral Researcher at KIT (Germany)
Abstract
Machine learning (ML) emulators have emerged as powerful tools to address the computational demands of process-based models, allowing for rapid predictions without sacrificing accuracy. In our study, we compared random forests and neural networks to approximate the carbon dynamics simulated by the LPJ-GUESS dynamic global vegetation model in forest ecosystems. Our trained emulators successfully captured both global and biome-specific carbon dynamics under several climate scenarios; however, we observed trade-offs between model performance and interpretability. The random forest-based emulator slightly outperformed the neural network predictions in overall accuracy, but the neural networks extrapolated better to climate scenarios not seen during training and provided more physically consistent predictions, as verified by model interpretability. In this talk, I will present our main findings, including the relative strengths of each ML model in terms of generalisation and physical consistency, as well as the challenges encountered in developing the emulators.
Biography
Postdoctoral Researcher at the Institute of Meteorology and Climate Research, Atmospheric Environmental Research (IMK-IFU), Karlsruhe Institute of Technology (KIT), Germany.
Carolina Natel de Moura joined the Global Land Ecosystem Modelling group at KIT as an Alexander von Humboldt Postdoctoral Fellow in January 2022. Since January 2024, she is a KIT YIG Prep Pro Postdoctoral Fellow. Her research interests lie in addressing the impacts of climate change on forest ecosystems by using machine learning (ML). She has been developing an ML emulator of a state-of-the-art ecosystem model (LPJ-GUESS) to accelerate simulations of global forest carbon stocks and fluxes under climate change. She completed her Ph.D. studies at the Federal University of Parana (Brazil) in 2021. During her PhD, she was awarded a Swiss Excellence Scholarship as a guest student at the University of Zurich, where she applied both conceptual (bucket-type model) and deep learning methods to hydrological modelling under changing climate conditions.
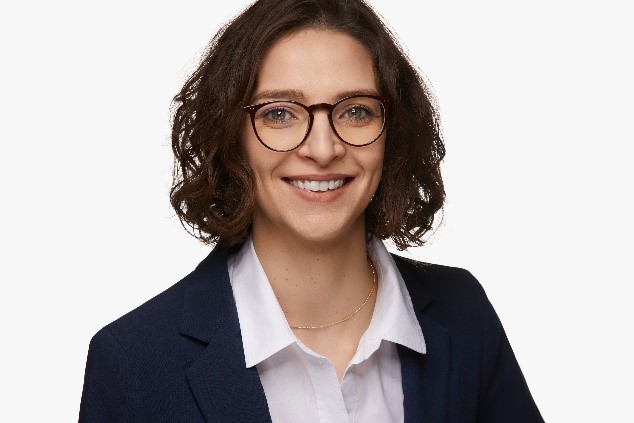
Monday, October 14
Conference Room, Visionen
10:15 - 11:00
Improving Representations of Ice Crystals in Atmospheric Models: Insights from in situ Imagery and Machine Learning
Joseph Ko, Postdoctoral Research Scientist at Columbia University (USA)
Abstract
Ice clouds are difficult to model due to large (spatiotemporal) scale disparities and an incomplete understanding of microphysics. A key ice microphysical property is particle habit (i.e., shape). At the single-particle scale, habit influences processes such as growth, collection, radiation interactions, and settling velocity. At the bulk-cloud scale, habit influences the radiative transfer, spatial distribution, smaller scale dynamics and ultimately the evolution of ice clouds. One way we can constrain the representation of ice crystal habit in models is to use in-situ measurements from airborne field campaigns. More specifically, cloud particle imagers (CPI) have been deployed in numerous airborne field campaigns, but limited analyses have been conducted using the vast dataset of millions of images.
In this work, we leverage both supervised and unsupervised ML to unravel the underlying microphysical diversity of ice cloud crystals. In Part 1 of this talk, I present how we use computationally generated, “synthetic” ice crystals to train models that can predict 3-D properties (e.g., mass, surface area) from 2-D images. Preliminary results show that ML models outperform linear models for predictive tasks, and we find that even non-deep-learning algorithms (e.g., random forest) produce models that can infer certain 3-D properties with high accuracy. In Part 2, we explore how representation learning can be used to extract meaningful information from CPI images in an unsupervised manner. As a concrete example, I will demonstrate how generative models can distill crystal representations down to their most important latent components, leading to quantitative improvements in downstream tasks such as unsupervised classification. Specifically, we use variational autoencoders and the k-means algorithm to classify CPI images without any labels. Furthermore, we attempt to disentangle and interpret the underlying factors of variation in the latent space using disentangled representation learning. In conclusion, I will discuss how ML-driven knowledge discovery will improve ice microphysical parameterizations in atmospheric numerical models such as climate models.
Biography
Joe Ko is a postdoctoral research scientist at the NSF Learning the Earth with AI and Physics (LEAP) Center at Columbia University. His current research involves using machine learning to constrain ice microphysical parameterizations using in-situ observations and cloud-scale LES simulations. He received his PhD in Environmental Engineering with an MS in Computer Science from the University of Southern California, and a BS/MS in Environmental Engineering from the University of California, Berkeley. In addition to cloud microphysics, he is also interested in interdisciplinary research topics such as urban climate, local to regional climate adaptation and mitigation, and air quality.
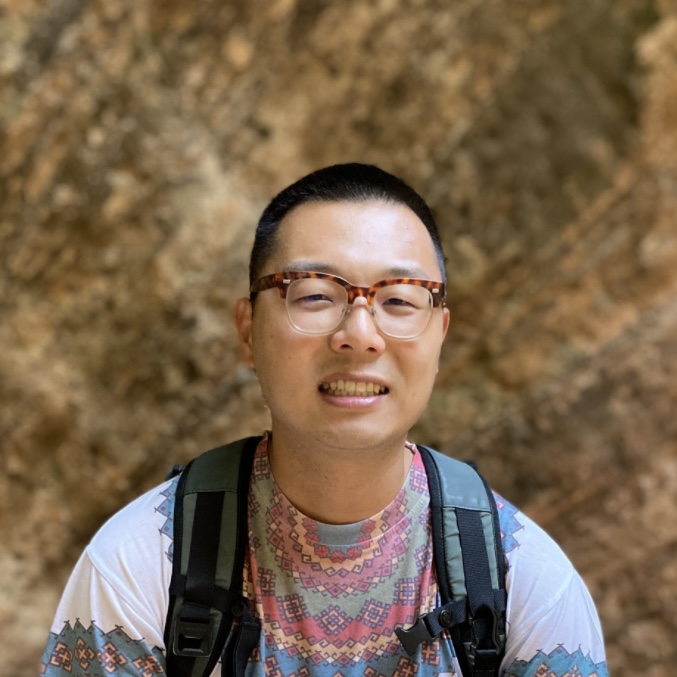
11:15 - 12:00
Hybrid climate simulations: embedding machine-learned sub-grid variability to improve biases
Dan Giles, Senior Research Fellow at University College London (UK)
Abstract
Physics-based climate models exhibit known long-standing biases. The coarse grid resolution required by the computational constraint of integrating over long time scales introduces the need for parameterisation schemes to capture sub-grid scale dynamics. This work embeds a machine learning model within Atmospheric General Circulation (AGCM) models to tackle long-standing precipitation biases by interacting with the existing parameterisation schemes. A multi-output Gaussian Process (MOGP) is trained on high resolution (km scale) simulation data to predict the sub-grid scale variability of temperature and specific humidity profiles. The MOGP model is then coupled in-situ with a coarse resolution AGCM to form a hybrid approach, with the predicted sub-grid variability used to perturb the climate grid cell profiles at fixed timesteps. In this talk, we present proof of concept work showcasing the benefits of embedding a data-driven model within a simplified AGCM. Additionally, we will discuss tentative results from integrating this approach within the Community Earth System Model (CESM) running on heterogeneous computing systems.
Biography
Dr. Dan Giles is a Senior Research Fellow in machine learning for weather and climate science at the Centre for AI in University College London. He acts as a theme lead for UCL’s Met Office Academic Partnership. His research interests focus on the intersection of data-driven approaches, numerical techniques and high-performance computing in the area of ocean and atmospheric sciences. Of particular interest are data assimilation problems and the coupling of machine learning and physics-based solvers. He obtained his PhD at University College Dublin for the development of fast computational methods for tsunami modelling, winning the Bertram Broberg medal for the best PhD thesis in the College of Engineering, Mathematical and Physical Sciences.
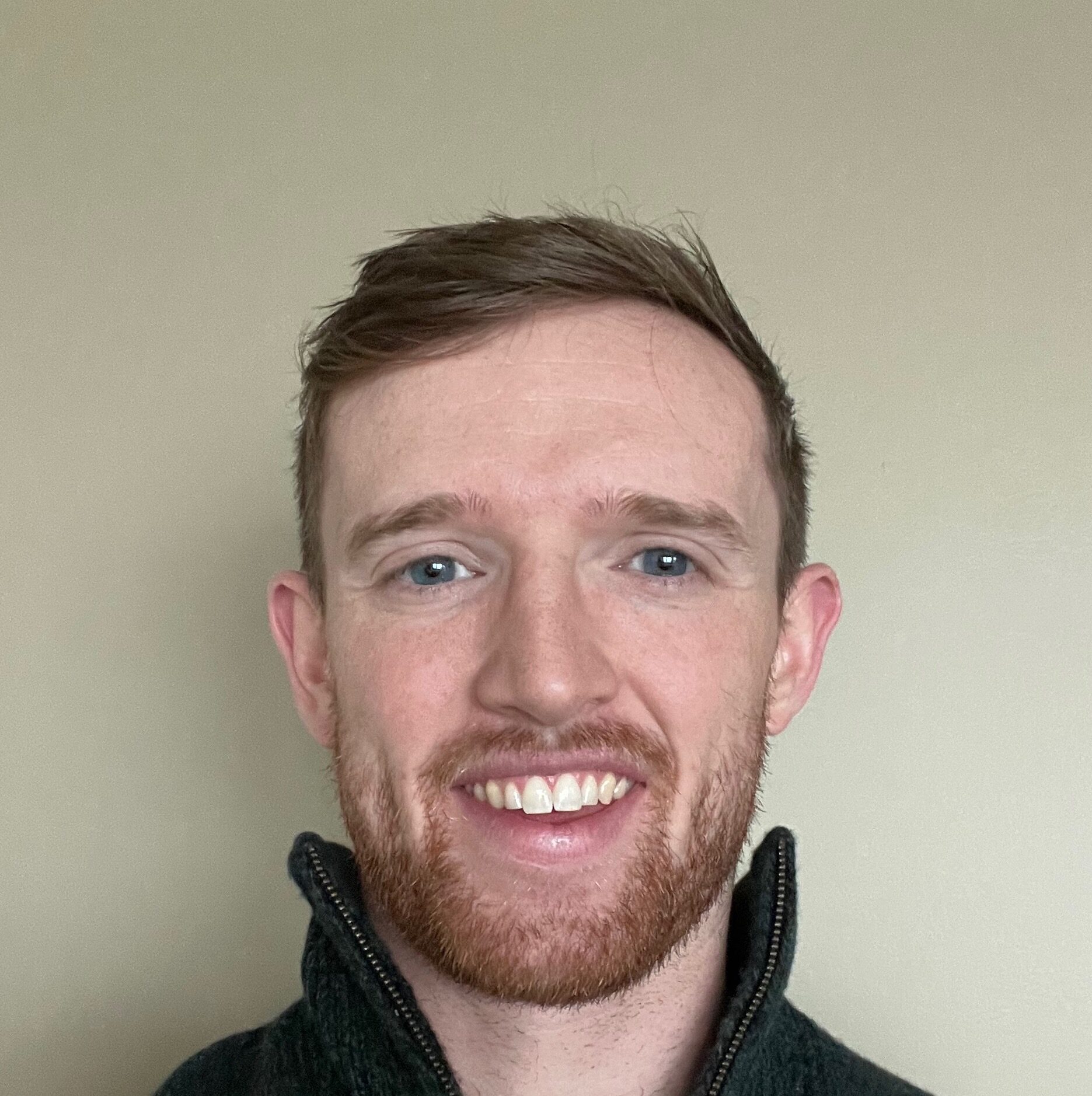
Tuesday, October 15
Conference Room, Visionen
10:15 - 11:00
Coupling a radiative transfer emulator and self-supervised neural network training for the retrieval of sun-induced fluorescence from hyperspectral imagery
Jim Buffat, PhD Student at Forschungszentrum Jülich (Germany)
Abstract
The remote assessment of photosynthetic activity of plants is interesting for various scientific and ecological applications. The retrieval of sun-induced fluorescence (SIF) from passively sensed hyperspectral remote sensing data has been identified as key corner stone to mapping photosynthetic dynamics efficiently over large areas of land. ESA’s upcoming Earth Explorer satellite mission FLEX that aims to provide global hyperspectral imagery for SIF retrieval has sparked an increased interest in the development of physically based retrieval models. Physically constrained SIF retrieval makes use of radiative transfer models (RTMs) to invert the measured at-sensor radiance to top-of-canopy fluorescence emission. In this talk, a SIF retrieval method is presented that aims at a tight integration of RTM simulation and self-supervised neural network training to reduce the prohibitive computational cost of physical models deployed to continuous data streams from hyperspectral data acquisition. To this end, we couple an emulator of large-scale radiative transfer simulations with a lightweight encoder-decoder neural network architecture. The method was developed and successfully tested on high-quality data of the HyPlant sensor, the airborne demonstrator sensor for FLEX in typical field conditions as well as in more challenging terrain. In an application and validation of our method with data of the spaceborne DESIS sensor we furthermore could show that our approach yields consistent SIF estimates even in spectrally low-resolved imagery. As a consequence, this new method potentially expands the number and design requirements of airborne and spaceborne sensors capable to provide accurate fluorescence products.
Biography
Jim Buffat is a doctoral student supervised by Hanno Scharr and Uwe Rascher at the Forschungszentrum Jülich institutes IAS-8: Data Analytics and Machine Learning and IBG-2: Plant Sciences. He received his Master’s degree in Geography with a specialization in Remote Sensing in 2021 from the University of Zurich following a BSc in Physics. His research interests include machine learning for optical remote sensing applications. His thesis focuses on deep learning-based retrieval of sun-induced fluorescence from airborne and spaceborne hyperspectral imagery.
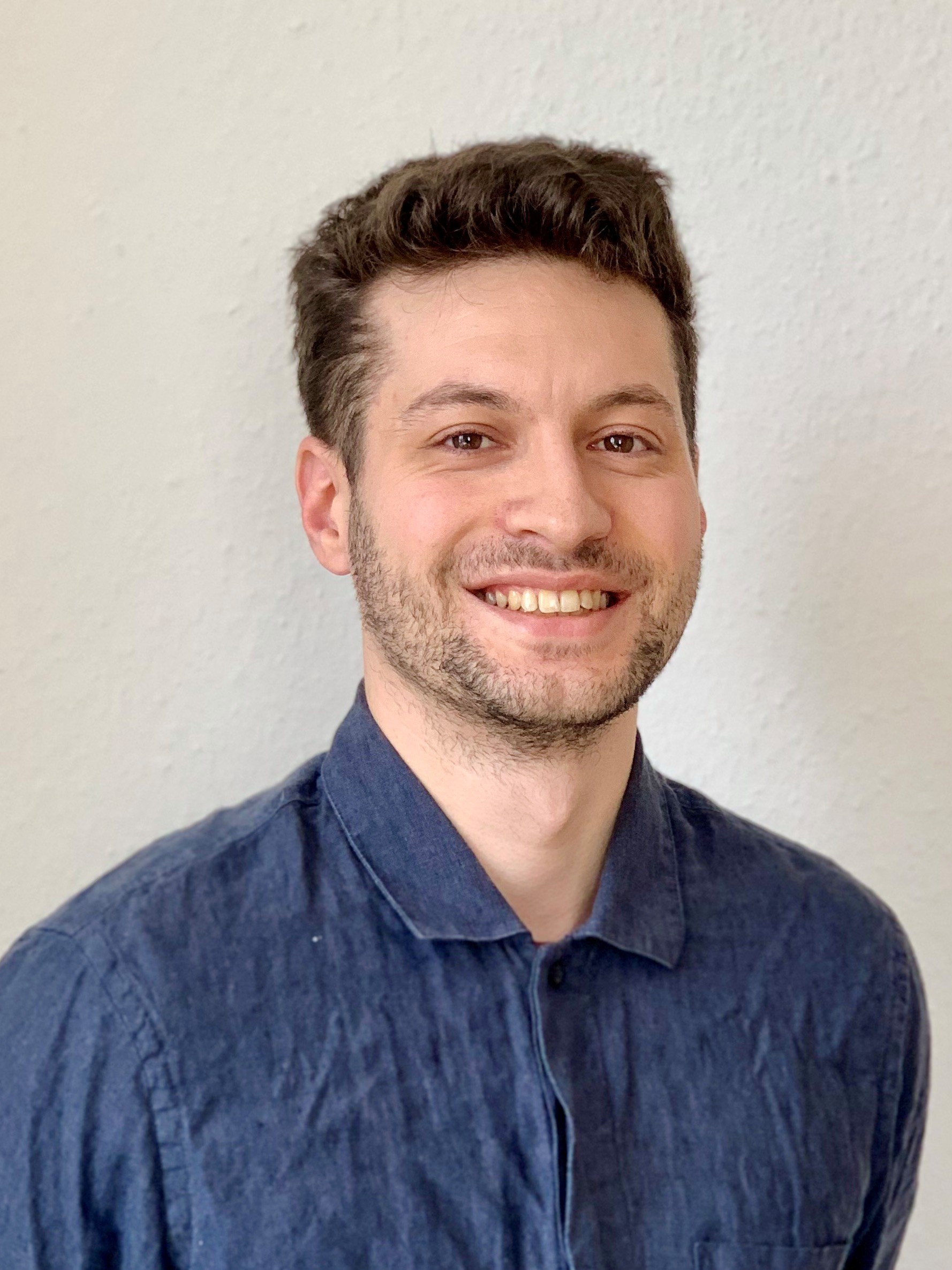
11:15 - 12:00
From Playing Satellite Geoguesser to Effective Location Embeddings
Marc Rußwurm, Assistant Professor at Wageningen University (The Netherlands)
Abstract
Learning representations of geographical space is vital for any machine learning model that integrates geolocated data, spanning application domains such as remote sensing, ecology, or epidemiology. Additionally, location information can support transfer and meta-learning strategies to train and deploy machine learning models across different countries and continents. This talk investigates the question how to construct and train a location encoding neural network to learn an implicit neural (geo)representation of coordinates. The first part covers our recent ICLR paper that presents an location encoder with Spherical Harmonic basis functions and sinusoidal representation networks (Siren). The second part then shows that these location encoders can then be trained on a contrastive geolocalization pretext task similar to playing the popular Geoguesser game. This results in effective location embeddings that can be efficiently integrated in other neural networks and improve prediction performance on diverse location dependent tasks including temperature prediction, animal recognition, and population density estimation.
Biography
Marc Rußwurm is Assistant Professor for Machine Learning and Remote Sensing at Wageningen University. His background is in Geodesy and Geoinformation, and he obtained a Ph.D. in Remote Sensing Technology at TU Munich. As a postdoctoral researcher, he joined the Environmental Computational Science and Earth Observation Laboratory at EPFL, Switzerland. His research interests are developing modern machine learning methods for real-world remote sensing problems, such as classifying vegetation from satellite time series and detecting marine debris in the oceans. He is interested in domain shifts and transfer learning problems naturally arising from geographic data.
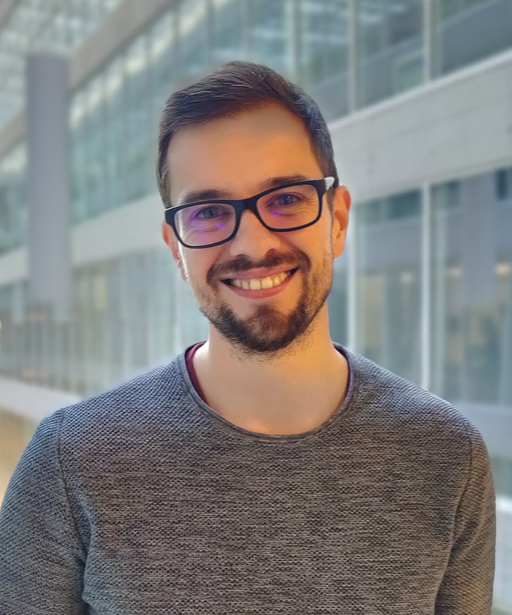
Wednesday, October 16
Conference Room, Visionen
10:15 - 11:00
Visual Analytics Pipeline to Improve Impact-Based Weather Warning Systems
Carlo Navarra, Research Engineer at Linköping University (Sweden)
Abstract
Extreme weather events, such as flooding, are expected to increase in frequency and intensity. Therefore, the prediction of extreme weather events, assessment of their local impacts in urban environments, and implementation of adaptation measures are becoming high-priority challenges for local, regional, and national agencies and authorities. This presentation features a visual analytics (VA) pipeline for the identification and exploration of extreme weather events and their impacts from volunteered geographic information texts and images retrieved from social media. The VA pipeline incorporates three main steps: (1) data collection, (2) image/text classification and analysis, and (3) visualization and exploration through an interactive visual interface.
Biography
Carlo Navarra is a research engineer at the Department of Thematic Studies – Environmental Change and the Centre for Climate Science and Policy Research at Linköping University. His research interests include Information and Geographical Visualization, and Visual Analytics.
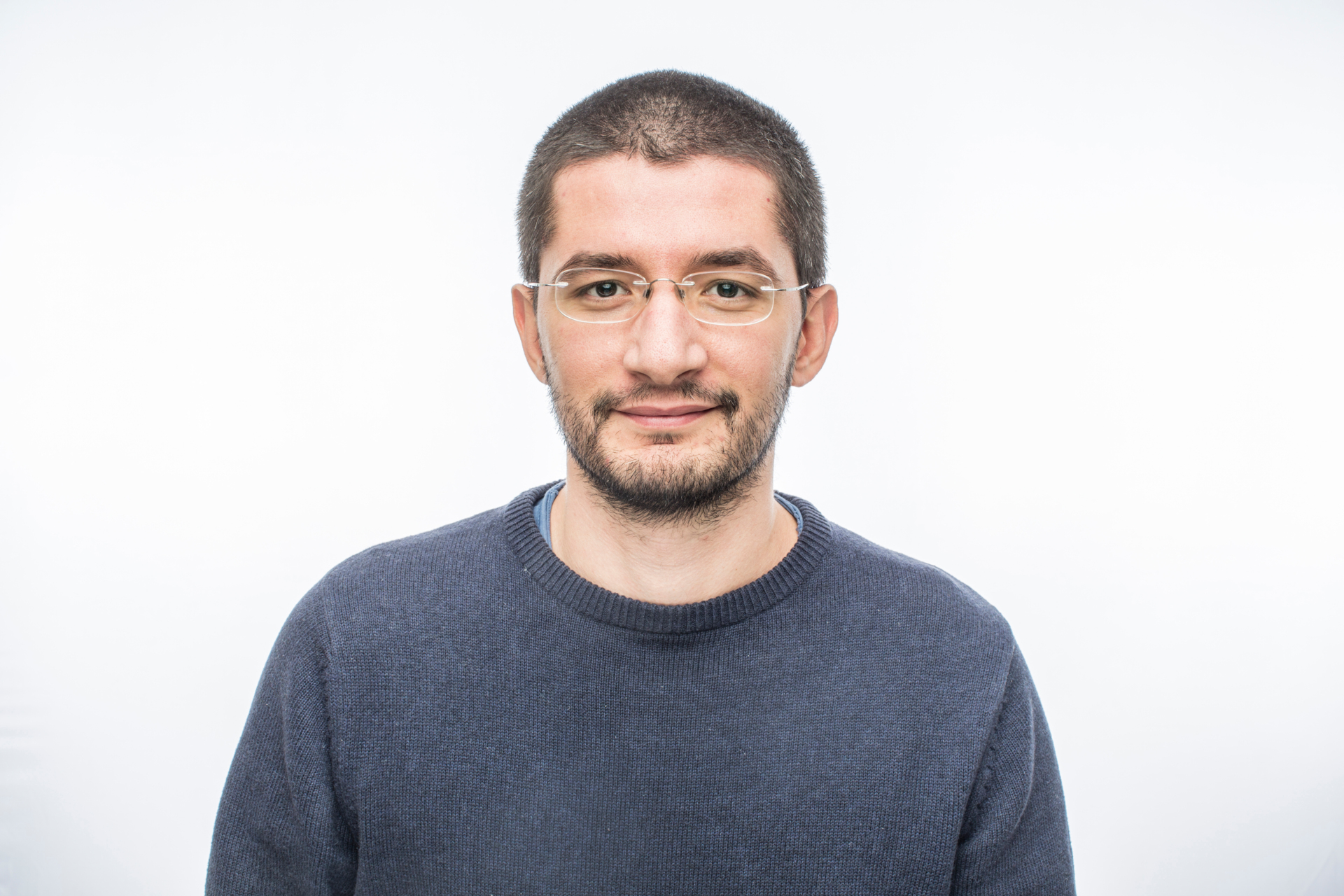
11:15 - 12:00
Data assimilation for Earth system models; challenges and ML remedies
Tomas Landelius, Researcher at SMHI (Sweden)
Abstract
Data assimilation in land, ocean, and atmosphere modeling is hindered by high-dimensional state spaces, nonlinearities, and complex error structures. This talk explores the potential of ML to address these issues. Autoencoders can reduce dimensionality, while diffusion or normalizing flows can transform non-Gaussian error distributions into more manageable forms. Self-supervised learning holds promise for capturing the interactions between land, ocean, and atmosphere. Additionally, generative models can efficiently provide ensembles for uncertainty quantification and probability estimates, potentially replacing computationally expensive physical models with faster ML surrogates. This presentation will showcase these challenges using examples from ongoing research projects at SMHI, emphasizing ML-driven solutions. Finally, the need for data assimilation as we know it is put into question.
Biography
Tomas Landelius has a PhD in Computer Vision from Linköping University (LiU). His thesis focused on reinforcement learning and he helped develop the first machine learning undergraduate course at LiU in 1996. Following his work at LiU, Tomas came to the Swedish Meteorological and Hydrological Institute (SMHI) in 1997. Here he developed a solar radiation model using an MLP to model the cloud effects. During his time at SMHI Tomas has been active in several national and EU projects concerning reanalysis (UERRA), data assimilation (IMPREX) and machine learning (DestinationEarth), often with connections to renewable energy sources. Tomas is a SMHI representative in the Swedish reference group for Horizon Europe cluster 4 – Digital, Industry & Space and in the International Energy Agency’s Photovoltaic Power Systems Program Task 16: Solar Resource for High Penetration and Large Scale Applications. Recently he started collaborating on machine learning for weather and climate prediction with the Division of Statistics and Machine Learning, Department of Computer and Information Science at LiU. A result of this is the first graph-based neural weather prediction model for limited areas. Currently Tomas is taking part in European efforts to make machine learning weather prediction models a natural part of the toolbox of a national meteorological service. In the future he’d like to explore the implications of the manifold hypothesis for probabilistic data assimilation and latent space prediction using sequential Monte Carlo methods and solutions to the Liouville and Fokker-Plank equations.
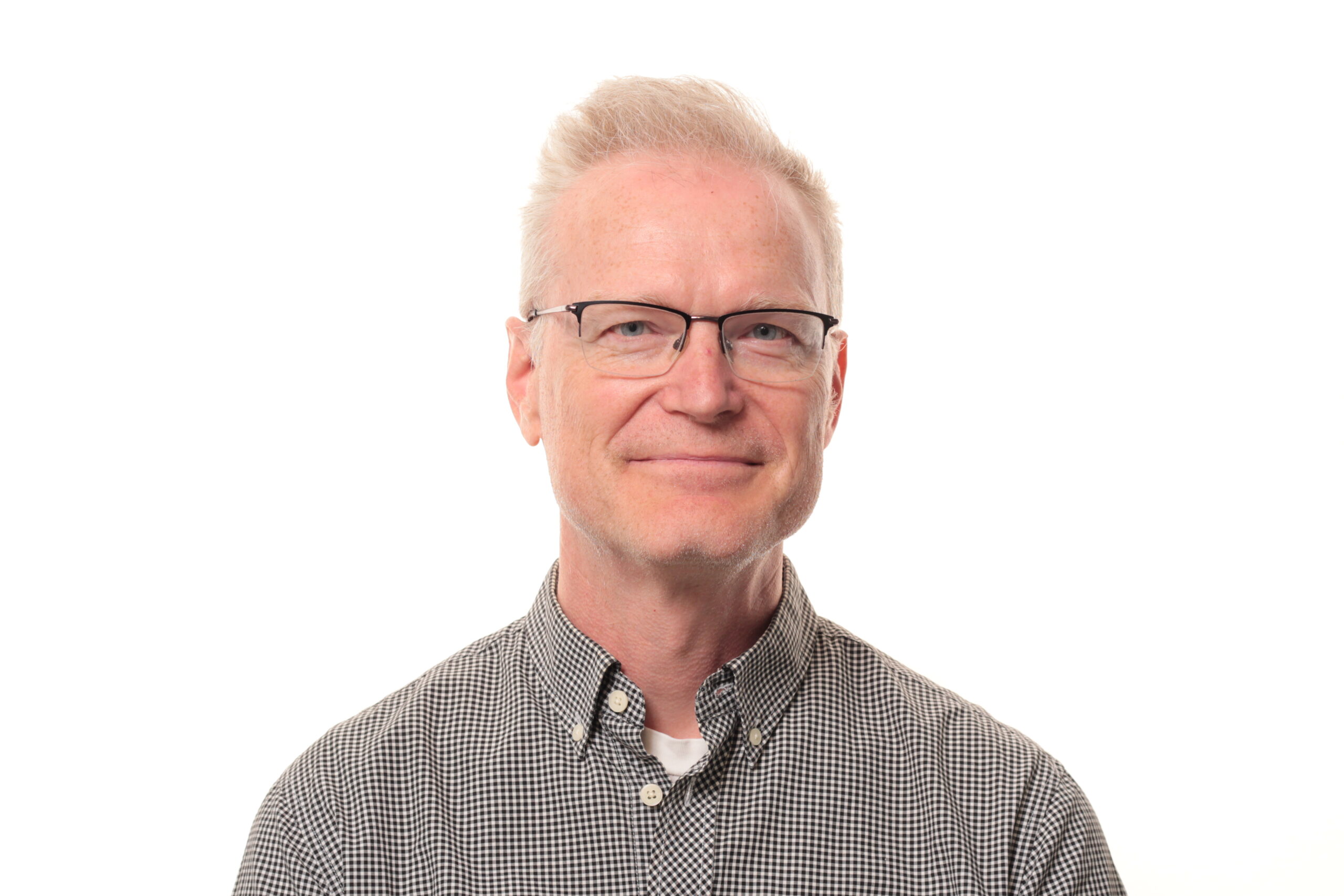
Thursday, October 17
Conference Room, Visionen
10:15 - 11:00
Climate-resilient snowpack estimation
Marianne Cowherd, PhD Student at UC Berkeley (USA)
Abstract
As we progress into the 21st Century, warmer temperatures and shifting atmospheric circulation patterns are expected to lead to notable alterations in the timing, quantity, and spatial distribution of hydrological processes. This change affects water security and infrastructure and is also expected to negatively impact our ability to measure these same effects. In other words, climate change is detrimental to our ability to observe climate change. In this project, we use snowpack measurement networks in the United States as a case for exploring a) how network-based in situ measurements will change in representativity in the future and b) how we can use machine learning and gridded meteorological products to remedy the decline in sensor network usefulness. To do this, we investigate the performance of the Snow Telemetry network under a suite of dynamically downscaled CMIP6 models. We then use the WRF data to develop artificial intelligence-based models of snow distribution under future climates. Our primary focus is to discern the relative significance of model complexity and observational data in constraining snowpack estimates, especially when applied to downscaled climate models over the WUS. Our findings indicate that sufficiently intricate data-driven models are likely to sustain the accuracy of snowfall and snowpack estimates even under no-analog future climate scenarios. Additionally, these models can handle novel spatial and temporal correlations between predictors of snowfall and end-of-winter snowpack, allowing for reliable estimation in the face of unprecedented atmospheric conditions. By employing nimble artificial intelligence-based models, adept at incorporating partial and multi-modal snowpack information, we can effectively tackle these challenges. This approach ensures the resilience of end-of-winter snowpack estimation in the WUS, even as the climate evolves into uncharted territory. Lastly, we discuss strategies for similar implementations in other networks of environmental sensors; sensor networks and field measurements tend to follow political boundaries due to funding structures but hydrology does not recognize the same borders.
Biography
Marianne Cowherd is a PhD candidate and Computational Sciences Graduate Fellow in the UC Berkeley Department of Environmental Science, Policy, and Management. Her recent work addresses how hydrologic measurements must respond to change in the environment from climate change and wildland fire. This includes using artificial intelligence to help snow measurement and water supply prediction keep pace with climate change.
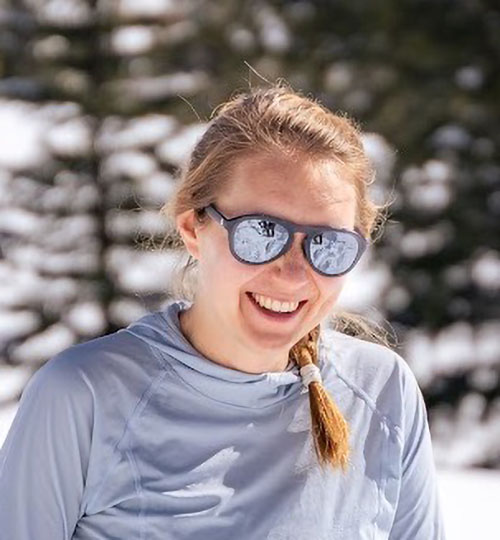
Friday, October 18
Conference Room, Visionen
10:15 - 11:00
Automatic pruning of ill-conditioned parameters in weather models using Gaussian processes
So Takao, Postdoctoral scholar at the California Institute of Technology (USA)
Abstract
Parameter tuning is a major challenge in heavily parameterised weather models, which are typically sensitive to the choice of parameters – a slight perturbation from a “good” parameter value can lead to the model being too stiff, or worse, have solutions that blow up in finite time. In this talk, I will present an experimental approach to automatically prune these so-called “bad” parameters by considering inverse problems in the context of a recently developed method of solving PDEs with Gaussian processes. I will present initial experimental results along with some theoretical results to back our observations.
Biography
So Takao is a postdoctoral scholar at the California Institute of Technology, working on the intersection of machine learning and data assimilation. His interests involve (1) developing efficient inference techniques for probabilistic models such as Gaussian processes and stochastic partial differential equations, (2) developing machine learning models with physical, geometric or topological inductive biases, and (3) applying machine learning techniques to problems in weather or climate science. During 2021-2023, he was a Senior Research Fellow in Machine Learning for Climate Science at the UCL Sustainability and Machine Learning group. There, he lead the Met Office Academic Partnership workgroup on “Data science methodology for weather and climate” and intiated various collaborations across departments to work on problems related to climate change. He received his PhD in 2020 from the Department of Mathematics at Imperial College London, where he wrote his thesis on structure-preserving fluid models and MCMC techniques on Lie groups, advised by Prof. Darryl Holm.
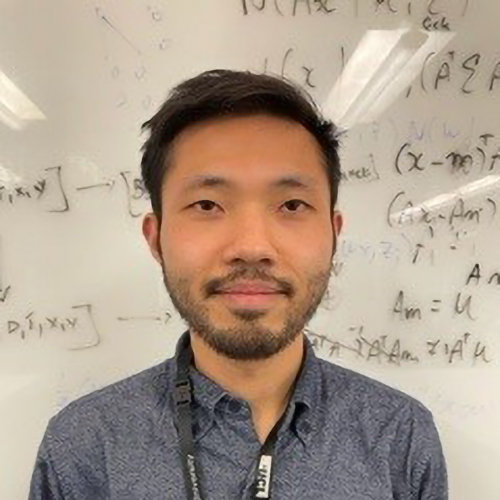
11:15 - 12:00
Gaining insight into forest disturbances using Machine Learning
Carolina Natel de Moura, Postdoctoral Researcher at KIT (Germany)
Abstract
Recent advances in machine learning (ML) and remote sensing technology have opened up new possibilities for forest monitoring. This talk will explore how deep learning, integrated with high-resolution satellite imagery, is being used to improve our understanding of natural and human disturbances in forests. This approach provides insights that can support the development of strategies to adapt forests to the challenges posed by both climate change and human pressures.
Biography
Postdoctoral Researcher at the Institute of Meteorology and Climate Research, Atmospheric Environmental Research (IMK-IFU), Karlsruhe Institute of Technology (KIT), Germany.
Carolina Natel de Moura joined the Global Land Ecosystem Modelling group at KIT as an Alexander von Humboldt Postdoctoral Fellow in January 2022. Since January 2024, she is a KIT YIG Prep Pro Postdoctoral Fellow. Her research interests lie in addressing the impacts of climate change on forest ecosystems by using machine learning (ML). She has been developing an ML emulator of a state-of-the-art ecosystem model (LPJ-GUESS) to accelerate simulations of global forest carbon stocks and fluxes under climate change. She completed her Ph.D. studies at the Federal University of Parana (Brazil) in 2021. During her PhD, she was awarded a Swiss Excellence Scholarship as a guest student at the University of Zurich, where she applied both conceptual (bucket-type model) and deep learning methods to hydrological modelling under changing climate conditions.
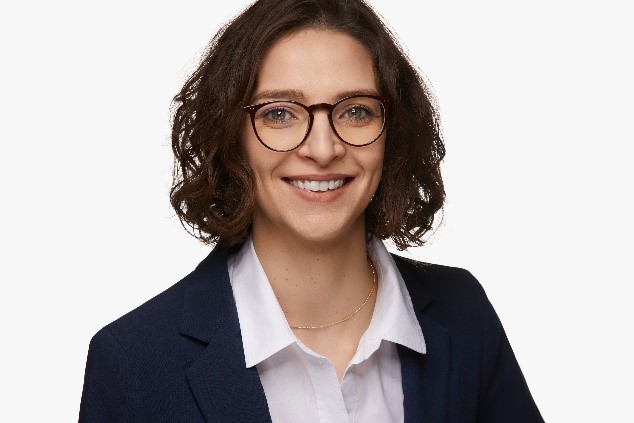