Photos from the symposium
Detailed program
October 7, 2024
17:00 - 19:00
Munkkällaren
Welcome reception at Munkkällaren
A welcome drink and some hors d’oeuvres will be served.
Day 1 – October 8, 2024
08:30 - 09:00
Registration
09:00 - 09:15
Opening
Organizing Committee
09:15 - 10:00
Why all emergent constraints are wrong but some are useful – a machine learning perspective
Peer Nowack, Karlsruhe Institute of Technology (KIT)
Abstract
Global climate change projections, such as those from the Coupled Model Intercomparison Project phase 6 (CMIP6), are still subject to substantial modelling uncertainties. A variety of Emergent Constraints (ECs) have been suggested to address these uncertainties, but remain heavily debated in the scientific community. Still, the central idea behind ECs to relate future projections to already observable quantities has no real substitute. Here I discuss machine learning (ML) approaches for new types of controlling factor analyses (CFA) as a promising alternative. The principal idea is to use ML to find climate-invariant relationships in historical data, which also hold approximately under strong climate change scenarios. On the basis of existing big data archives such as those from the CMIPs, these climate-invariant relationships can be validated in perfect-climate-model frameworks. From a ML perspective, I argue that CFA are promising for three reasons: (a) they can be objectively validated both for present-day data and future data and (b) they provide more direct – by design physically-plausible – links between historical observations and potential future climates compared to ECs and (c) they can take higher dimensional relationships into account that better characterize the still complex nature of large-scale emerging relationships. I highlight these advantages for a few examples, including global cloud feedback and the stratospheric water vapour feedback.
Biography
Peer Nowack holds the Chair for Artificial Intelligence (AI) in Climate and Environmental Sciences since March 2023. His group works on a diverse range of scientific challenges in climate science and atmospheric chemistry. For this, he combines machine learning techniques, numerical Earth system models, and Earth observations (e.g., satellite data) in innovative ways.
Before taking up his position at KIT, Peer Nowack worked for almost 10 years in the UK. Most recently, he held a permanent position as Lecturer in Atmospheric Chemistry and Data Science at the University of East Anglia (UEA), which he took up in 2020 after leading a junior research group at Imperial College London. In 2016, Peer Nowack had completed his PhD at the University of Cambridge following his undergraduate studies in Interdisciplinary Sciences at ETH Zurich (Switzerland). During summer 2022, he was a guest researcher at the National Center for Atmospheric Research (USA).
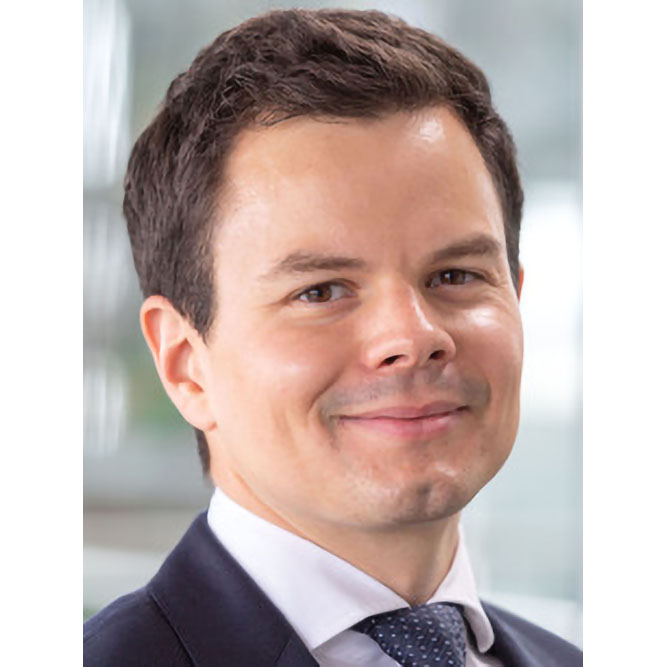
10:00 - 10:30
Coffee
10:30 - 11:15
Leveraging Machine Learning at the Rossby Centre: From Seasonal Forecasting to High-Resolution Downscaling
Ramón Fuentes Franco, SMHI
Abstract
The climate research unit (Rossby Centre) of SMHI is currently working on developing Machine Learning tools to improve seasonal forecasts and to perform high-resolution climate simulations using coarse-resolution predictors from Global Climate Models (downscaling).
Seasonal forecasts are important for different sectors of society such as agriculture and renewable energy. However, the skill of seasonal forecasts is still limited. Improving these forecasts is thus of uttermost importance.
We have developed an ML-based algorithm to improve operational seasonal forecasts. This Python framework is called Predictive Research for Enhanced Climate Information SystEm (PRECISE).
PRECISE uses as predictors atmospheric variables from any operational seasonal forecast system. From the CMCC operational forecast, we used the ensemble mean for the predictor and initialised on December 1st, predicting the whole month of December. We predict monthly precipitation for December, and use as ground truth the observed precipitation from ERA5. The model used by PRECISE is an eight-layer deep convolutional neural network (DCNN). To be able to train the DCNN, we performed data augmentation. The results show that PRECISE increases the correlation between the observed and predicted precipitation in the whole European continent compared to the operational system CMCC3.5. With correlations r>0.8 in big portions of southern and western Europe.
In turn, for the downscaling, stakeholders require information on climate change risk at local scales. Statistical downscaling (SD) methods are computational cheap compared to dynamical regional models. Deep learning (DL)-based techniques have emerged as a promising approach for SD because of their ability to learn complex spatial features from large spatiotemporal datasets. Within the EU-project OptimESM, we developed a SD-methodology based on fully convolutional neural networks.
To test the method, we downscaled ERA5 data (25 km resolution) to 5.5 km for a pan-European area, using CERRA data as training data, for temperature and precipitation. Results are promising and biases small compared to dynamical regional downscaling. The next step is to extend the methodology for downscaling global models to high resolution.
Biography
Ramón is a climate researcher at the Swedish Meteorological and Hydrological Institute since 2016. Before, his current position he was a postdoctoral fellow at the International Centre for Theoretical Physics in Trieste, Italy where he carried out several regional and global studies using mainly the Regional Climate Model RegCM. He did his PhD at the Ensenada Center for Advanced Studies and Higher Education in Baja California, Mexico. His current lines of research are related to global climate variability and change, particularly on how global modes of variability impact the regional climate. Lately, he has started to work on implementing machine learning methods to climate science, particularly focusing on applying and developing ML methods for downscaling coarse resolution global model data to high resolution, which provides more detailed climate information for regional studies. He is also working on algorithms to detect extreme events from climate variables, using as predictors the current and previous states of the atmosphere. He is currently leading two work packages in the Horizon projects OptimESM and AI4PEX, applying ML methods for climate studies.
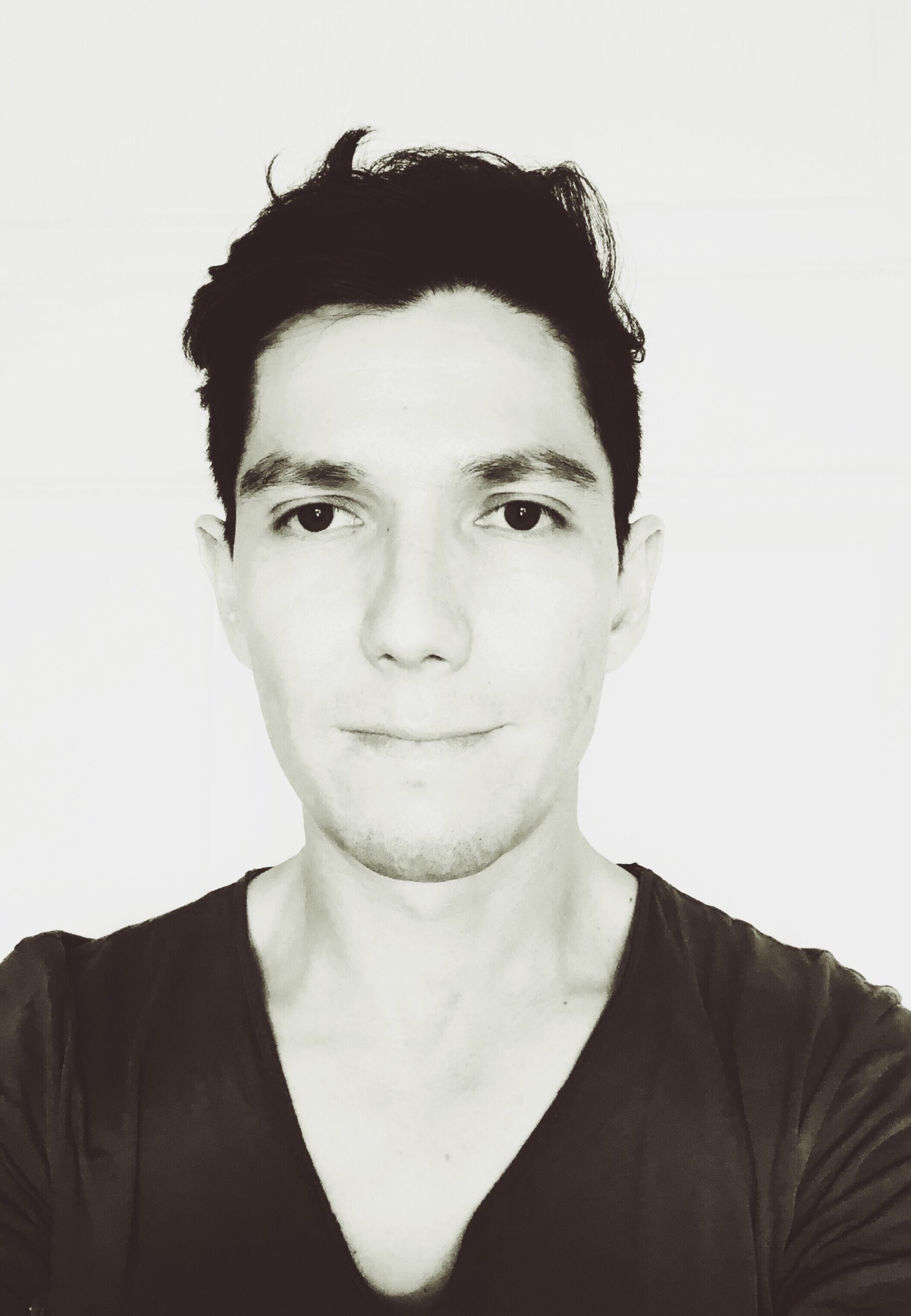
11:15 - 12:00
Confronting Climate Change with Generative and Self-supervised Machine Learning
Claire Monteleoni, INRIA Paris / University of Colorado Boulder
Abstract
The stunning recent advances in AI chatbots rely on cutting-edge generative deep learning algorithms and architectures trained on massive amounts of text data. Generative deep learning has also shown remarkable results when trained on video data and on combinations of different data types (i.e., multi-modal). The recent advances in generative deep learning can also benefit a variety of applications for addressing climate change. For example, generative deep learning trained on climate and weather data can be a powerful tool in generating an ensemble of weather predictions and in quantifying the uncertainty of long-term projections of climate change.
As opposed to text and video, the relevant training data for this domain includes weather and climate data from observations, reanalyses, and even physical simulations. As in many massive data applications, creating “labeled data” for supervised machine learning is often costly, time-consuming, or even impossible. Fortuitously, in very large-scale data domains, “self-supervised” machine learning methods are now actually outperforming supervised learning methods. In this talk, I will survey our lab’s work developing generative and self-supervised machine learning approaches for applications addressing climate change, including detection and prediction of extreme weather events, and downscaling and temporal interpolation of spatiotemporal data. Our methods address problems such as forecasting the path and intensity of tropical cyclones, renewable energy planning, and projecting future sea-level rise.
Biography
Claire Monteleoni is a Choose France Chair in AI and a Research Director at INRIA Paris, a Professor in the Department of Computer Science at the University of Colorado Boulder, and the founding Editor in Chief of Environmental Data Science, a Cambridge University Press journal launched in December 2020. She has previously held positions at University of Paris-Saclay, CNRS, George Washington University, and Columbia University. She completed her PhD and Masters in Computer Science at MIT and was a postdoc at UC San Diego. She holds a Bachelor’s in Earth and Planetary Sciences from Harvard. Her research on machine learning for the study of climate change helped launch the interdisciplinary field of Climate Informatics. She co-founded the International Conference on Climate Informatics, which turned 13 years old in 2024, and has attracted climate scientists and data scientists from over 20 countries and 30 U.S. states. She gave an invited tutorial: Climate Change: Challenges for Machine Learning, at NeurIPS 2014. She currently serves on the U.S. National Science Foundation’s Advisory Committee for Environmental Research and Education, and as Tutorials co-Chair for the International Conference on Machine Learning (ICML) 2024.
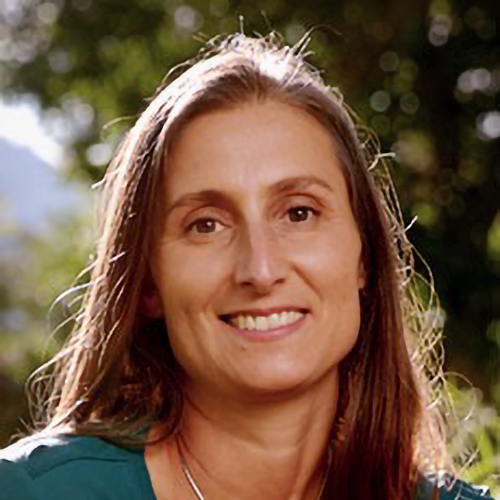
12:00 - 13:30
Lunch
13:30 - 14:15
Presentation by the Focus Period Visiting Scholars
14:15 - 15:00
Challenges and opportunities in the representation of land surface processes driving terrestrial carbon and water dynamics
Nuno Carvalhais, Max Planck Institute for Biogeochemistry
Abstract
Process representation and generalization are longstanding challenges in describing carbon and water dynamics in terrestrial ecosystems. Despite our confidence in the conceptual understanding of processes underpinning the observed responses we fall short on defining robust model structures and parameterizations, while testing structural assumptions. Here is set forth a perspective for leveraging machine learning approaches to maximize the use of observations within physically robust representations of carbon cycle dynamics. This perspective is based on examples that challenge assumptions about terrestrial ecosystems that drive process parameterization; and on the ideas of process abstraction and of emulation to bridge the scaling gap between process understanding, its representation, and observations. Examples focus on vegetation processes of photosynthesis and ecosystem carbon losses ranging from local to global scales. Ultimately, a forward perspective on the issues of equifinality and attribution are dependent not only on model structures and observational constraints but also on the scrutiny of results across performance metrics.
Biography
Nuno Carvalhais is a scientist at the Max Planck Institute for Biogeochemistry, where he leads research in the field of Earth system science. With a strong background in environmental science and ecology, Nuno focuses on understanding the dynamic interactions between terrestrial ecosystems and the global carbon and water cycles. His work integrates observational data, theoretical insights, process-based modelling and machine learning to unravel how land ecosystems respond to and influence climate change and variability. He holds a PhD from the New University of Lisbon (Portugal), has been visiting scientist at NASA – Goddard Space Flight Center, and Boston University, and is also a member of the ELLIS unit Jena. Nuno’s research interests include terrestrial ecosystem dynamics; global biogeochemical, carbon and water cycles; model-data fusion approaches; model evaluation and development; machine learning; hybrid modelling; vegetation remote sensing; Earth system feedbacks.
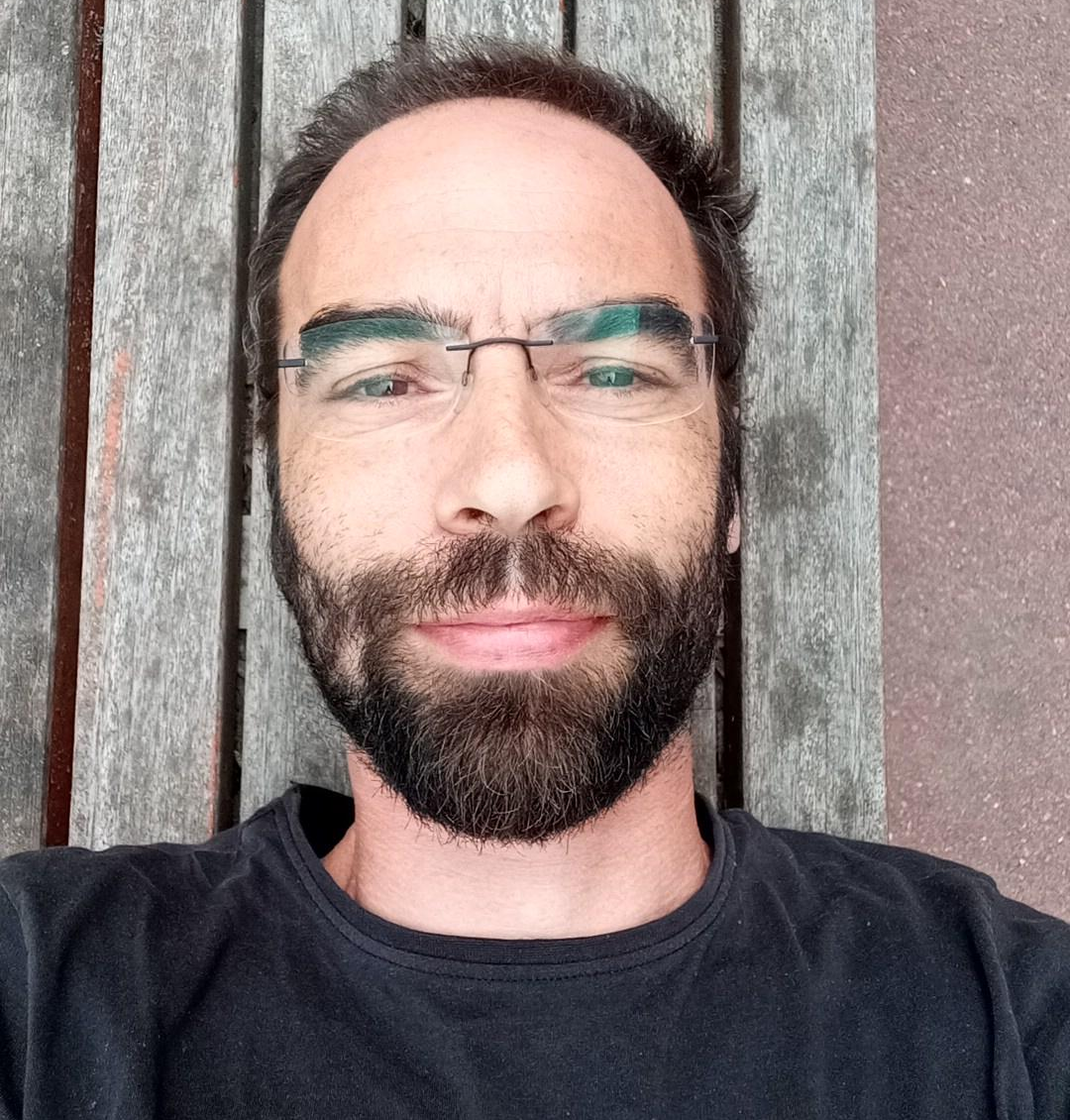
15:00 - 15:30
Coffee
15:30 - 16:15
Forecasting weather with neural PDEs
Markus Heinonen, Aalto University
Abstract
Neural PDEs have surfaced in the last decade as a new perspective on modelling dynamics by learning the governing equations as a black-box neural network. While succesful on systems of limited complexity, large-scale demonstrations have been lacking. Recently large transformer models have been developed with impressive weather forecasting performances, but largely with little consideration of the underlying physics. We consider global weather as a continuous-time PDE with mass-preserving dynamics, and show how small convolution networks can learn weather evolution with good accuracy. In this talk I will summarise recent approaches in data-driven PDE weather prediction models.
Biography
Markus Heinonen is an Academy Research Fellow at Aalto University, Finland with a PhD from University of Helsinki in 2013. His research interests are centered on probabilistic machine learning with emphasis on understanding uncertainty of deep learning with Bayesian perspectives to neural networks. In addition he has worked on incorporating neural networks and Gaussian processes into learning ODE, SDE and PDE dynamics.
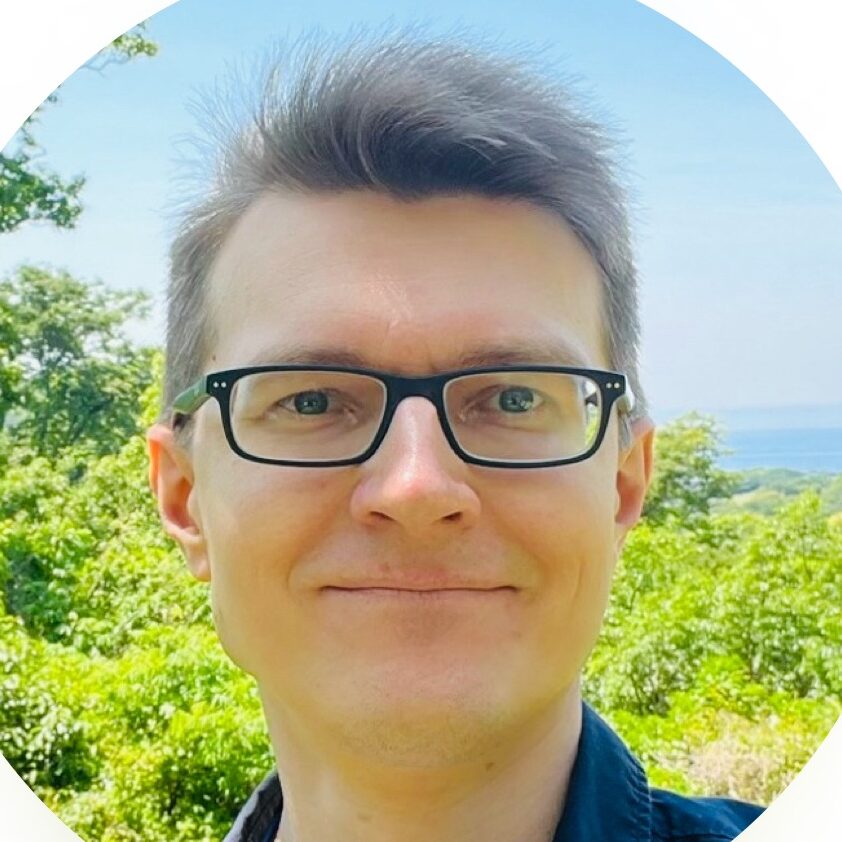
16:15 - 17:00
Learning cloud processes across scales using scientific machine learning
Kara Lamb, Columbia University
Abstract
Clouds remain one of the greatest sources of uncertainty in predicting future climate, as they involve complex, non-linear processes that extend from the submicron scale to the kilometer scale. Our current ability to model clouds is limited by significant uncertainties, particularly in the intricate microphysical processes that govern the interaction and growth of cloud droplets and ice crystals, as well as in accurately modeling clouds across the relevant temporal and spatial scales for climate. Recent advances in scientific machine learning offer promising methods to address these challenges. I will discuss several recent studies applying these methods to cloud processes. First, I will discuss how physics-informed machine learning and recently developed equation discovery methods can be used to reduce structural uncertainty in models of ice growth in the atmosphere using in situ observations from laboratory experiments and airborne field campaigns. Second, I will discuss how data-driven reduced order modeling can be used to develop simplified (bulk) microphysics schemes in an unsupervised manner from more detailed microphysical models. Finally, I will discuss how these methods can be used to learn relevant information from high resolution global storm resolving models, to improve the prediction of precipitation extremes by representing cloud processes at the spatial scales needed to accurately predict processes at the climate scale.
Biography
Dr. Kara Lamb is an Associate Research Scientist at Columbia University in the NSF Learning the Earth with Artificial Intelligence and Physics (LEAP) Center. Her research lies at the intersection of observations and high-resolution modeling, with the goal of improving climate model parameterizations of aerosol and cloud processes. She combines traditional process-based approaches with data science, scientific machine learning, and reduced-order modeling. She was on the science team for the NASA KORUS-AQ and AToM aircraft campaigns and the NOAA FIREX Firelab study, was the lead mentor for the 2022 Frontier Development Laboratory Europe challenge on Aerosols, and is a member of the AMS STAC Committee on AI Applications to Environmental Science.
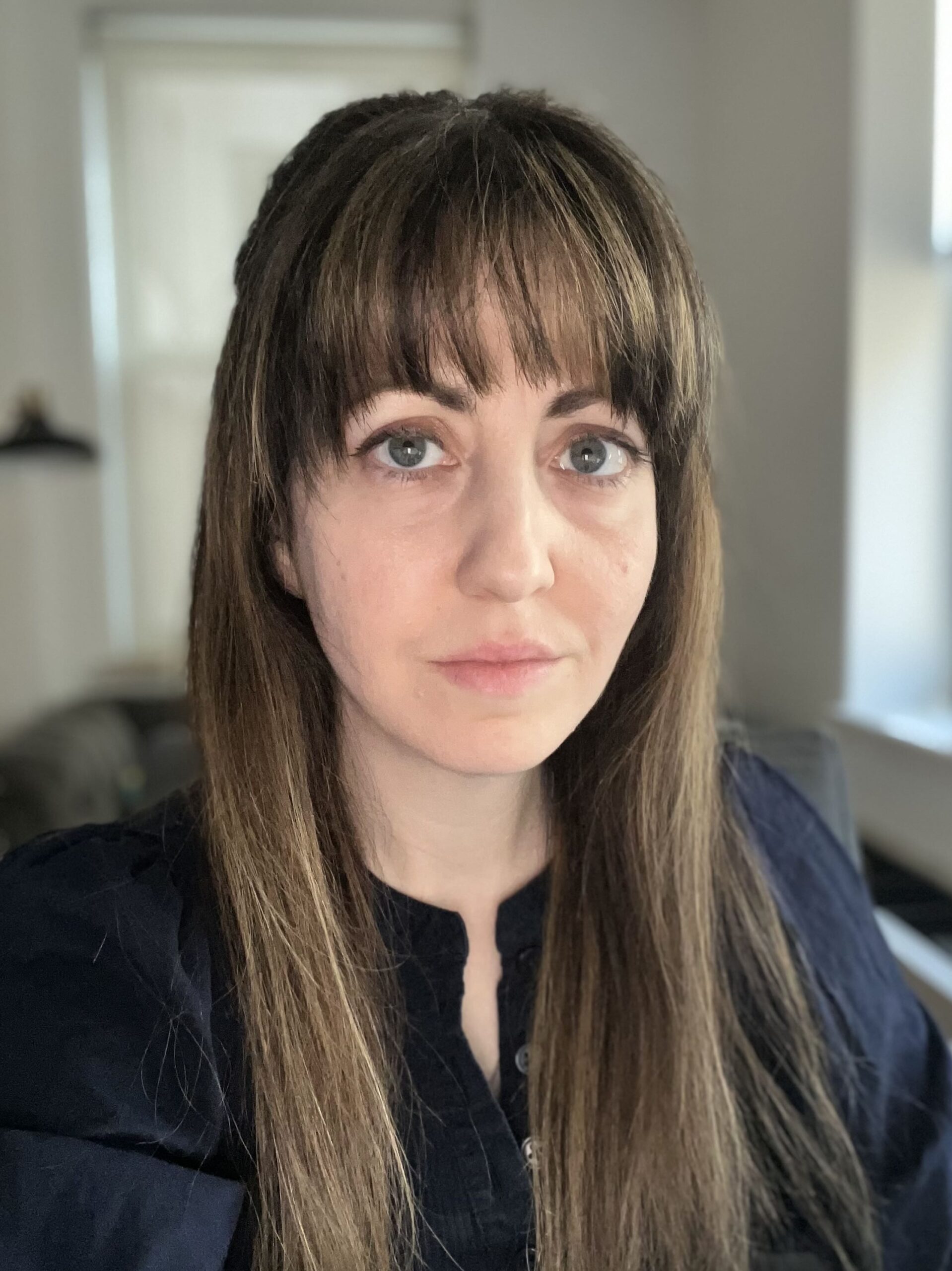
Day 2 – October 9, 2024
09:15 - 10:00
On the need for more machine learning in Environmental Sciences
David Bastviken, Linköping University
Abstract
Our capacity to reach sustainability depend on effective environmental analysis. This is key for identifying needs, goals, and policies, to prioritize efforts and validate outcomes, and to implement effective environmental legislation. Traditional environmental monitoring is costly per data-unit generated while funding for developing more cost-effective monitoring methodology have been limited in the environmental domain. In the era of escalating environmental change – with negative consequences for health, water and food supply, and triggering conflicts broadly on Earth – there are increasing demands on environmental analysis to support effective mitigation efforts. Yet, we now see clear cases for which legislation to combat climate change is difficult to implement because of lack of reliable monitoring methods.
Potential benefits of transforming environmental monitoring by learning from the rapidly growing data acquisition and processing capacity in other domains are tremendous. Remote sensing is developing rapidly, yet face important challenges. Ground-based environmental monitoring is still key but lag behind the general rapid technical development. Machine learning has a fundamental role to play to address these challenges. Many fantastic associated initiatives have been made and are emerging where the environmental data are rich enough. However, also other environmental domains that are still less data-rich need attention, in turn calling for methodological development. At the same time, when applying machine learning on ecological systems that are not primarily designed or governed by humans, and for which complex multi-species biological processes are key drivers, close attention to fundamental process understanding is important for methodological priorities and reliability. This presentation will depart from the above general picture, bring additional biogeochemical context, and provide some climate science-related examples illustrating the critical needs for more extensive integration of machine learning in environmental sciences.
Biography
Prof. Bastviken leads or have been leading multiple research projects regarding e.g., biogeochemical cycling of carbon, nutrients, and halogens, innovative technologies and approaches to measure associated processes including greenhouse gas fluxes and formation of disinfectant by-products in water treatment. Bastviken has been main- or co-advisor of >20 PhD students, supervised >65 Master or Batchelor Theses, and published >150 scientific peer-reviewed publications. His work addresses multiple spatiotemporal dimensions and covers local to global scales. Prof. Bastviken is a European Research Council (ERC) grantee and has been chairing the Swedish Research Council VR advisory group on research infrastructures for the environmental, climate, earth, and space sciences. Bastviken further serves as elected Vice Chair in the Research Council of Formas (the Swedish government research council for sustainable development). He contributed as a Lead Author to the 2019 Refinement to the 2006 IPCC Guidelines for National Greenhouse Gas Inventories.
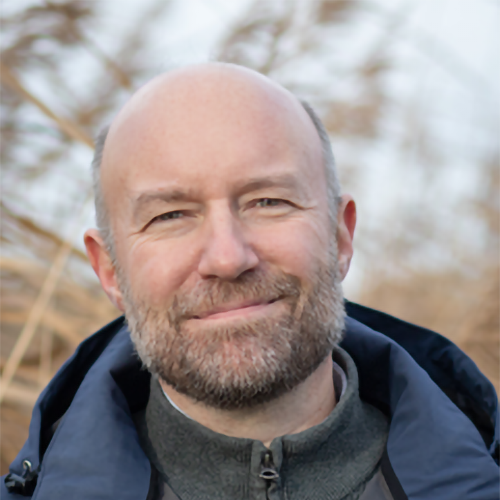
10:00 - 10:30
Coffee
10:30 - 11:15
On the benefit of explainable machine learning for the agricultural and environmental sciences
Ribana Roscher, Forschungszentrum Jülich and University of Bonn
Abstract
Machine learning methods have been increasingly used in scientific disciplines for some time. In addition to high accuracy, one desired goal is to understand how a particular decision was made. To achieve this goal and obtain explanations, knowledge from the domain is needed that can be integrated into the model or applied post-hoc. One example in this talk will focus on detecting wilderness characteristics with explainable machine learning and show that machine learning can not only be used to learn models that should align with our existing knowledge but can also lead to new insights.
Biography
Ribana Roscher is a Professor of Data Science for Crop Systems at the University of Bonn, Germany, and heads the same-titled group at the Institute of Bio- and Geosciences at Research Center Jülich, Germany. Until 2022, she was a Junior Professor of Remote Sensing with the University of Bonn. Before she was a Postdoctoral Researcher with the University of Bonn, the Julius-Kühn Institute, Siebeldingen, Germany, Freie Universität Berlin, Germany, and the Humboldt Innovation, Berlin, Germany. In 2015, she was a Visiting Researcher with the Fields Institute, Toronto, Canada, and in 2018 she was a Visiting Researcher with UCLA Institute for Pure & Applied Mathematics, Los Angeles, USA. Her research focus is pattern recognition and machine learning specifically for applications in agricultural and environmental sciences. She currently chairs the IAPR Technical Committee 7 ‘Remote Sensing and Mapping’ and the ISPRS Working Group ‘Machine Learning for Geospatial Data’.
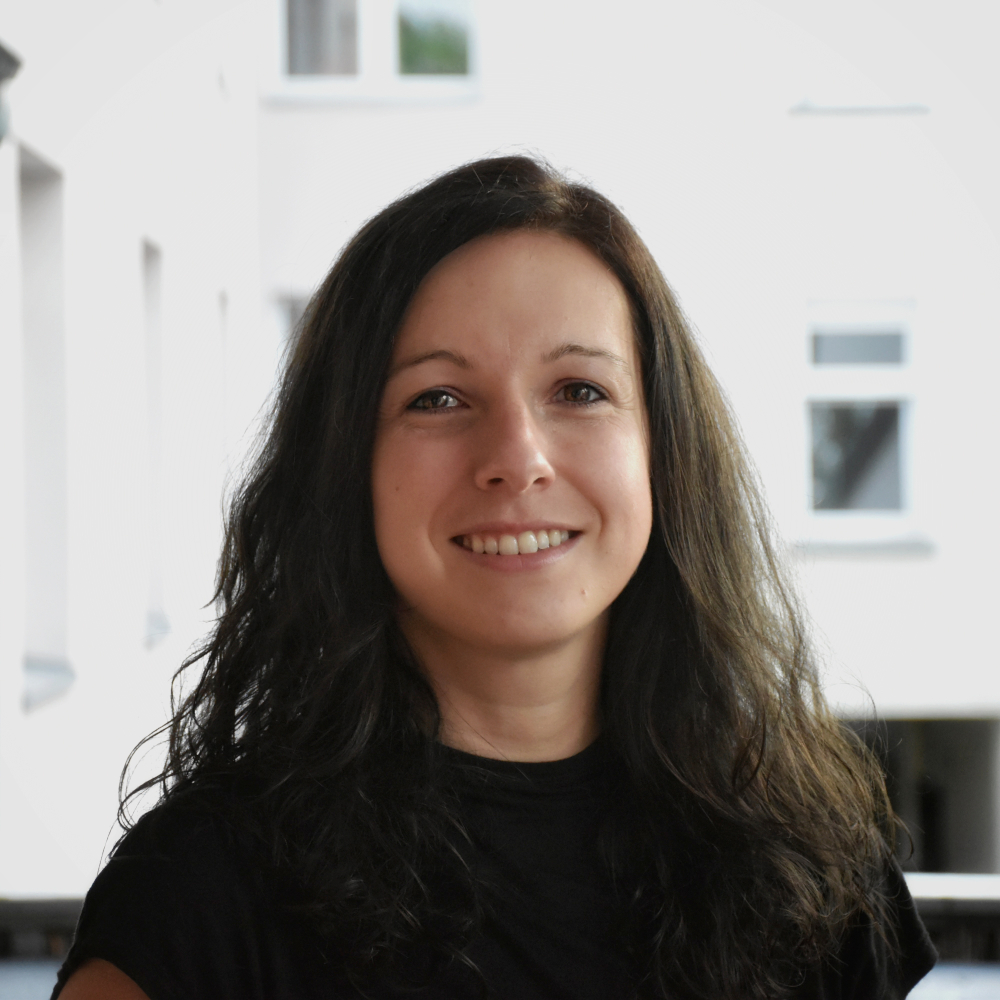
11:15 - 12:00
A Deep-learning-based Method for the Retrieval of Sun-Induced Plant Fluorescence from Airborne and Spaceborne Hyperspectral Imagery
Hanno Scharr, Forschungszentrum Jülich
Abstract
Sun-induced plant fluorescence (SIF) gives insights into the photosynthetic activity of plants. It is thus relevant not only for short-term agricultural tasks but also for plant-related climate factors. In view of ESA’s upcoming Fluorescence Explorer (FLEX) mission, we develop a method for SIF retrieval in the O2A band targeting two different sensors – (1) the airborne HyPlant sensor having similar specifications as the FLEX sensor, and (2) the spaceborne DESIS sensor on the International Space Station ISS. Our method aims at a tight integration of physical radiative transfer principles and self-supervised neural network training. To this end, a set of spatial and spectral constraints and a specific loss formulation are adopted. By this we provide the first method allowing to retrieve spaceborne SIF maps at 30 m ground resolution with a strong correlation to high-quality airborne SIF estimates.
Biography
Hanno Scharr received his Doctoral degree in Physics from Ruprecht-Karls University, Heidelberg, Germany in 2000 and a Habilitation in Computer Science from Goethe University, Frankfurt, Germany in 2014. From 2002 to 2003 he was Senior Scientist at Intel, Santa Clara, California. From 2003 to 2021 he led the Quantitative Image Analysis group at IBG-2: Plant Sciences, and since 2021 he heads IAS-8: Data Analytics and Machine Learning, both at Forschungszentrum Jülich, Germany. He is (co-)author of more than 150 scientific publications. His research interests include machine learning, computer vision, and image processing for applications in natural sciences.
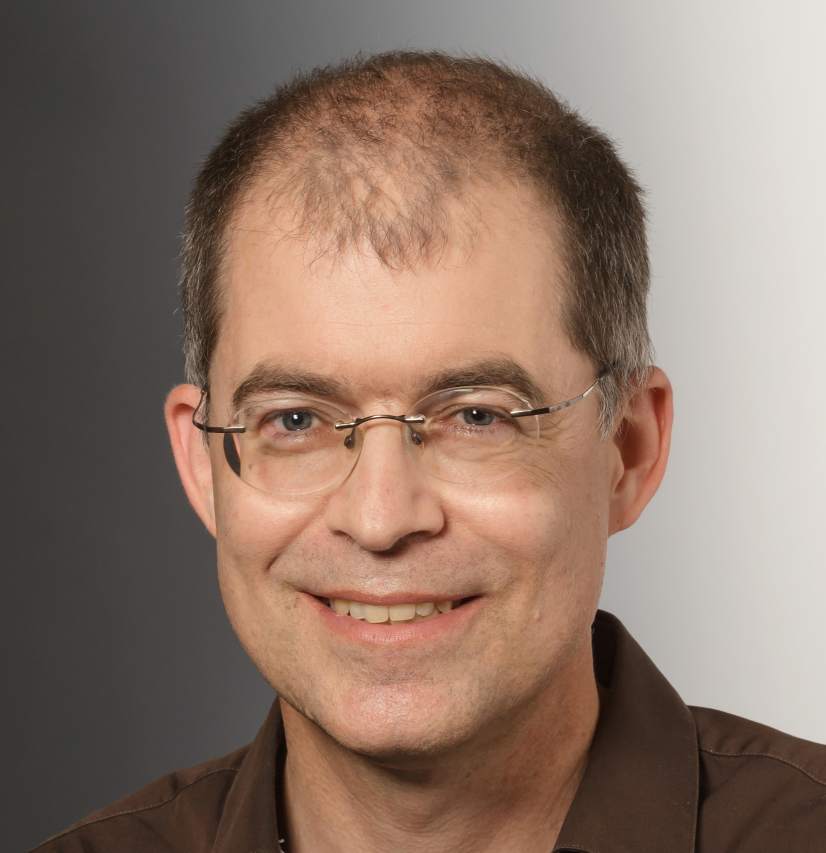
12:00 - 13:30
Lunch
13:30 - 15:00
Poster session
- Martin Andrae (LiU) Continuous Ensemble Forecasting with Diffusion
- Jim Buffat (ELLIIT Focus Period Visiting Scholar from Jülich) Coupling a radiative transfer emulator and self-supervised neural network training for the retrieval of sun-induced fluorescence from hyperspectral imagery
- Yiheng Du (SMIH) Hybrid approaches enhance hydrological model usability for local streamflow prediction
- Mikhail Ivanov (SMHI) Ensemble Enrichment and Time Interpolation via Deep Learning AND High Resolution Climate Change Information Through a Convolutional Neural Network
- Joseph Ko (ELLIIT Focus Period Visiting Scholar from Columbia University) Improving Representations of Ice Crystals in Atmospheric Models: Insights from in situ Imagery and Machine Learning
- Abhishek Lodh (SMHI) Ensemble Kalman filter based Land Surface Data Assimilation Developments at SMHI
- Swapan Mallick (SMHI) Leveraging Deep Learning for Uncertainty Estimation in Ensembles within the Copernicus Arctic Regional Reanalysis (CARRA-2) Domain
- Leonardo Olivetti (ELLIIT Focus Period Visiting Scholar from Uppsala University) A comparison of data-driven and numerical weather forecasting models on weather extremes
- Joel Oskarsson (LiU) Probabilistic Weather Forecasting with Hierarchical Graph Neural Networks
- Marc Rußwurm (ELLIIT Focus Period Visiting Scholar from Wageningen University) Geographic Location Encoding with Spherical Harmonics and Sinusoidal Representation Networks (Siren)
- So Takao (ELLIIT Focus Period Visiting Scholar from California Institute of Technology) GAUSSIAN PROCESSES ON CELLULAR COMPLEXES
- Daniel Yazgi (SMHI) Ensemble Enrichment and Time Interpolation via Deep Learning
15:00 - 15:30
Coffee
15:30 - 16:15
On the role of Machine Learning in climate change mitigation and adaptation – a reflection on challenges and opportunities in transdisciplinary climate research
Tina-Simone Neset, Linköping University
Abstract
As global annual temperatures for the first time breached the 1.5°C global warming mark (Copernicus Climate Change Service/ECMWF 2024), a threshold agreed on by 196 countries in the 2015 Paris agreement, climate change emerges as an ever more pressing challenge to the global community. High expectations are set on digital technologies and applications, including Machine Learning (ML), to accelerate efforts to mitigate greenhouse gas emissions and support climate governance by e.g., monitoring and assessing emissions, supporting and evaluating mitigation actions, and enforcing policy processes by providing transparency and accountability. Rising temperatures are simultaneously causing accelerated impacts across the globe, such as heat waves and flooding. These challenges call for concerted climate adaptation efforts to render society and ecosystems safe in a changing climate.
This presentation reflects on current challenges and opportunities related to ML for climate mitigation and adaptation from a transdisciplinary climate research perspective. It highlights examples of recent and ongoing research, and the collaborative processes required to ensure relevance and usability to policy makers, planners, and other societal actors.
Biography
Tina-Simone Neset is a Senior Associate Professor in Environmental Change and the Centre for Climate Science and Policy Research at Linköping University, Sweden. Her research concerns climate change, climate vulnerability & adaptation, and climate services, with a methodological focus on interactive visualization, participatory processes, and co-creation, and she leads and participates in multiple national and international research projects within climate and sustainability science. She currently leads a collaborative research project on AI-based image processing and text mining to support national impact-based weather warning systems and climate adaptation efforts. She has published >60 peer-reviewed scientific publications, and has supervised (main or co-supervisor) 8 PhD candidates, and >30 masters, bachelors and teachers exam theses. Neset is a board member of the Swedish foundation for strategic environmental research MISTRA, and for C-Research, Norrköping Visualization Centre-C, and has served on the evaluation committee for KLIMAFORSK for the Research Council Norway, and as reviewer for the Swedish Research Council FORMAS.
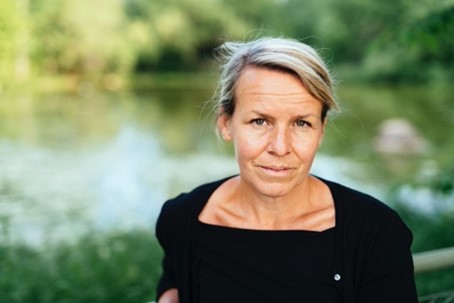
15:30 - 16:15
Deep Learning for Remote Sensing
Yonghao Xu, Linköping University
Biography
Yonghao Xu is an Assistant Professor at the Computer Vision Laboratory (CVL), Linköping University, Sweden. He received his Ph.D. degrees in photogrammetry and remote sensing from Wuhan University, China, in 2021. From 2021 to 2023, he was a Postdoctoral Researcher with the Institute of Advanced Research in Artificial Intelligence (IARAI), Austria.
He was a recipient of the First Place award in the IEEE Geoscience and Remote Sensing Society (GRSS) Data Fusion Contest in 2018. Since 2022, he has been working as the co-lead of the Benchmarking Working Group in the IEEE GRSS Image Analysis and Data Fusion Technical Committee. His research interests include remote sensing, computer vision, and machine learning.
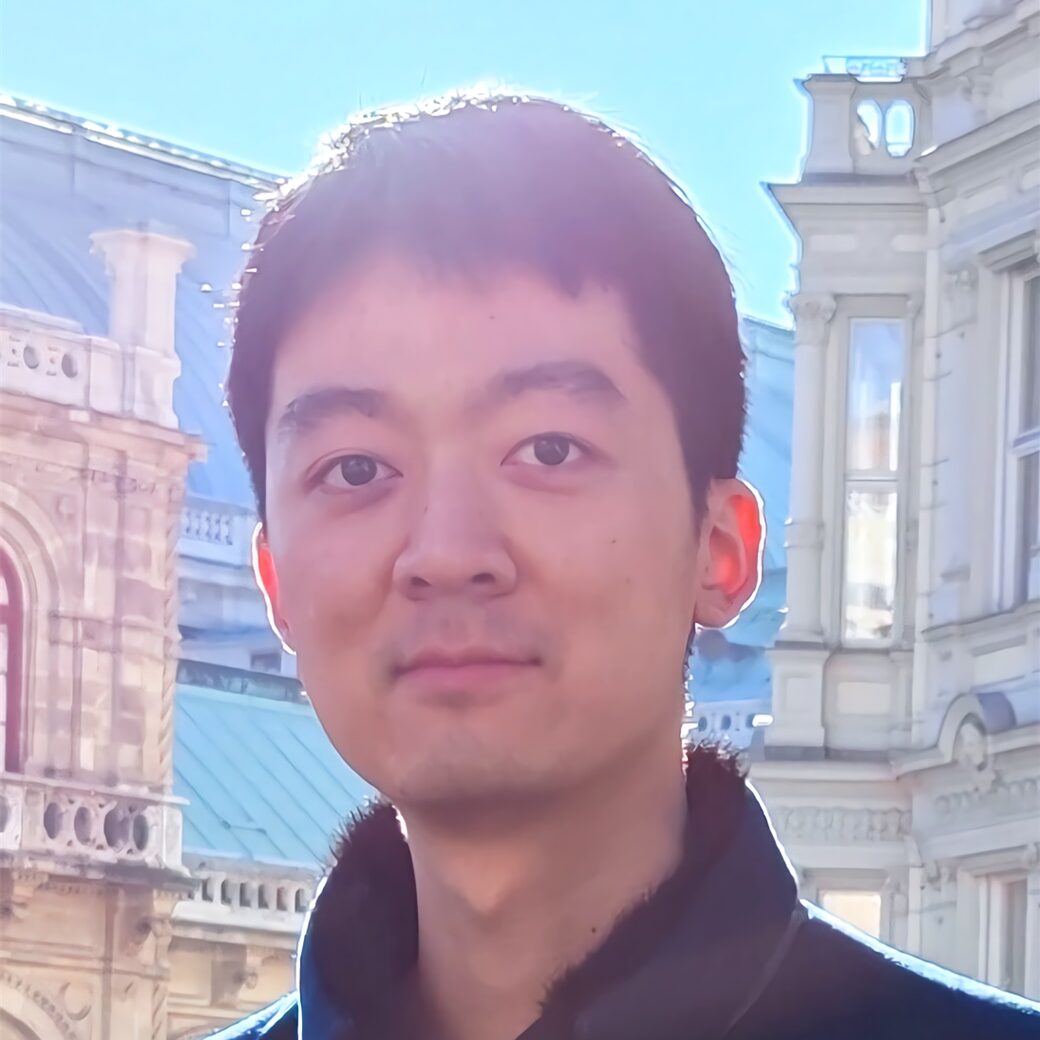
16:15 - 17:00
Semantic Perception and combined Symbolic and Subsymbolic AI for Reasoning about Climate Related changes in Urban Environments
Amy Loutfi, Örebro University
Abstract
In this talk I will be presenting a collection of works where semantic perception and machine reasoning have been combined to reason about possible futures related urban planning. The talk will cover various scenarios whereby semantic information not only improves human decision support but also can be leveraged from the AI system in order to improved both the machine learning performance as well as enable effective interactions with other non-human agents (e.g. robots). Additionally, the talk will also describe an on-going effort to leverage these techniques into concrete innovations for improved city planning.
Biography
Amy Loutfi is a Professor in Computer Science at Örebro University and leads the research group – AASS Machine Perception and Interaction Lab. She has a long experience working with industry and the public sector on research projects dealing with AI, robotics and human-robot interaction. Additionally, Amy has worked with a strong engagement in strategic issues in AI including WASP. In 2020, Amy was elected as a member of the Royal Swedish Academy of Engineering Sciences (Ingenjörsvetenskapsakademin, IVA)
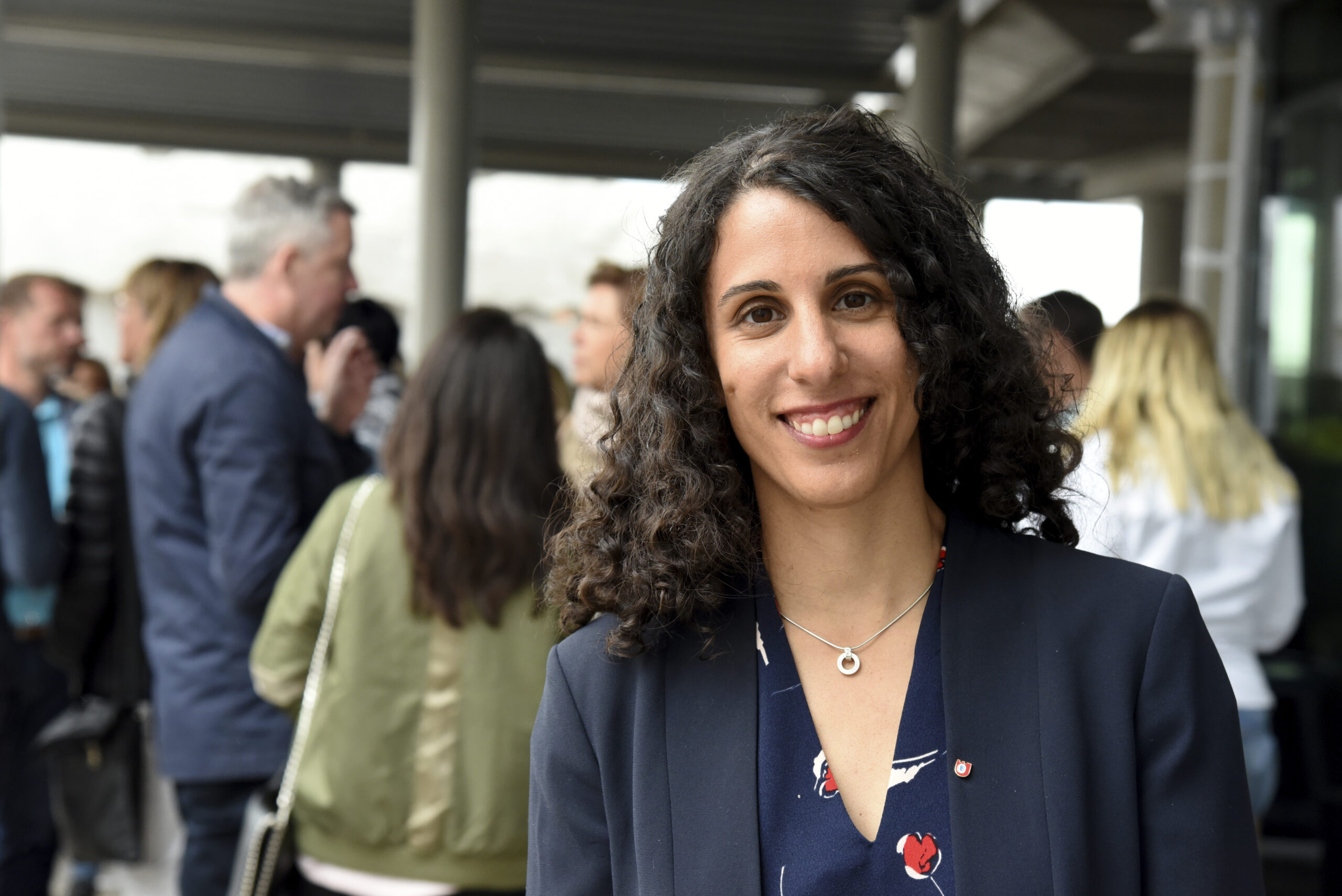
17:00 - 18:30
Break
18:30
Symposium Dinner
Livgrenadjärmässen, Brigadgatan 8, 587 58 Linköping.
Welcome drinks will be served from 18.30.
Dinner will be served at 19.15.
Day 3 – October 10, 2024
09:15 - 10:00
AtmoRep: a machine learning based multi-purpose model for atmospheric dynamics
Ilaria Luise, CERN
Abstract
AtmoRep is a first example of probabilistic multi-purpose model that extends the concept of representation learning to Earth system science, and in particular to atmospheric dynamics. The AtmoRep collaboration recently released a first prototype (www.atmorep.org) which, starting from a single pre-trained architecture used as a backbone, is able to achieve skilful results in multiple zero-shot applications such as nowcasting, temporal interpolation and scenario generation, compared to the state-of-the-art approaches. Thanks to a novel definition of the loss, the model is also probabilistic by design, as it outputs a set of ensemble members for each task, with well calibrated distributions as proven for weather forecasting. The talk will focus on the innovative aspects of the model architecture, the current results for the most relevant applications and the foreseen developments.
Biography
Ilaria Luise is a Senior Research Fellow at CERN, the European Center for Nuclear Research in Geneva. She works as a physicists within the Innovation Division of the CERN IT-Department. Her background is in high energy physics and big data management. She is one of the main developers of the AtmoRep project, which aims at exploring the potential of foundation models based on large scale Machine Learning trained on petabytes of Earth observations (ERA5) for weather and climate forecasting, in collaboration with the Juelich Supercomputing Center and the Department of Computer Graphics at the University of Magdeburg.
She is Co-PI of the EMP2 project at CERN, which is part of the CERN Innovation Programme on Environmental Applications (CIPEA). The EMP2 project aims at implementing the AtmoRep model into a digital twin engine. This is performed in collaboration with the EU funded InterTwin project and the Digital Twin initiative at CERN.
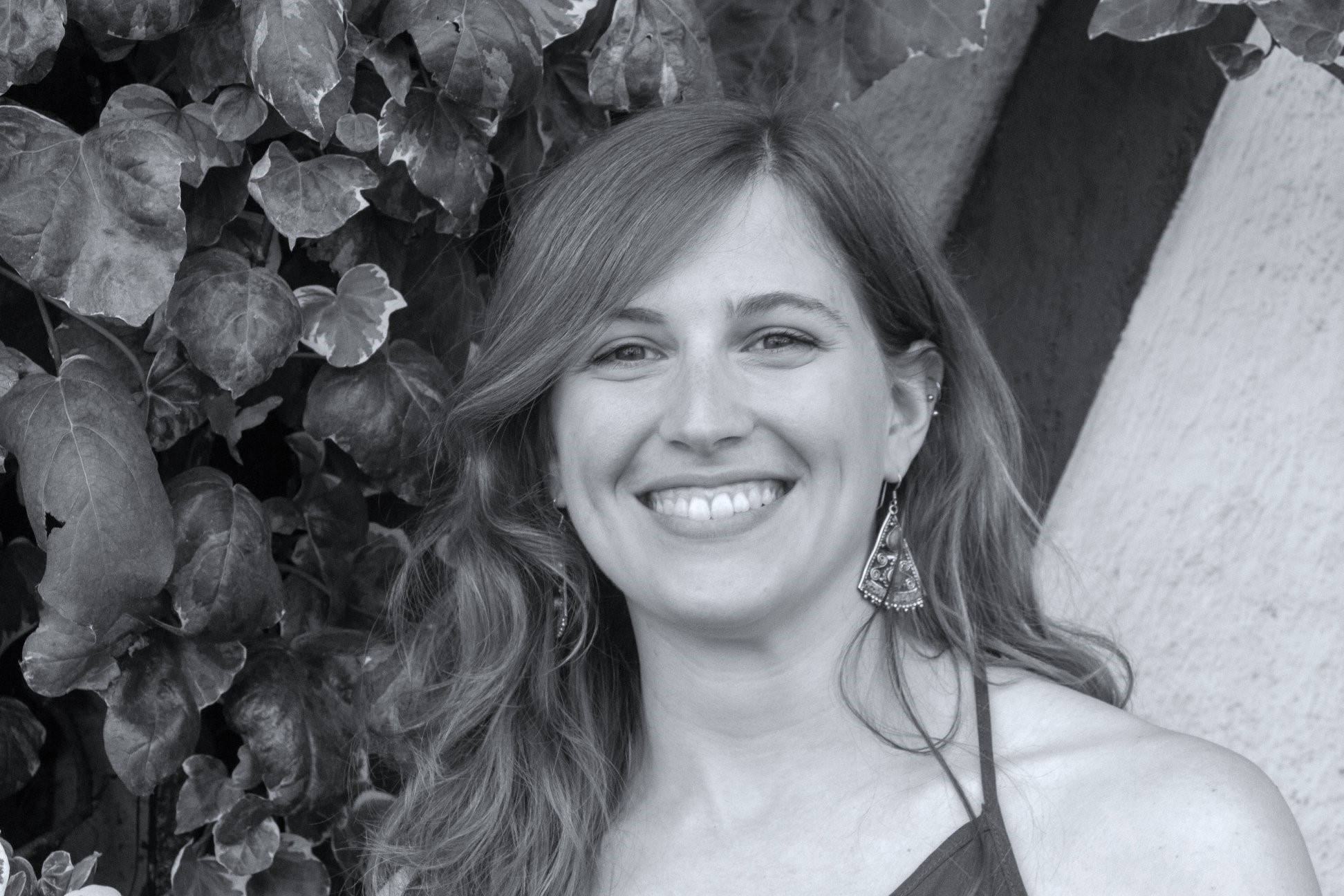
10:00 - 10:30
Coffee
10:30 - 11:15
Opportunities for Machine Learning to Accelerate Progress in Environmental Modelling
Marc Deisenroth, University Collage London
Abstract
Modelling complex environmental systems, such as weather or oceans, is extremely challenging. Recent advances in machine learning and AI to automatically learn complex relationships from data have opened up opportunities for data-driven methodologies to make a meaningful contribution to environmental science and even play the role of a transformative technology within environmental modelling. In this talk, I will focus on some of our recent work on using machine learning for data assimilation and medium-range weather forecasting
Biography
Professor Marc Deisenroth is the Google DeepMind Chair of Machine Learning and Artificial Intelligence at University College London, Deputy Director of the UCL Centre for Artificial Intelligence, and part of the UNESCO Chair on Artificial Intelligence at UCL. He also holds a visiting faculty position at the University of Johannesburg. Marc co-leads the Sustainability and Machine Learning Group at UCL. His research interests center around data-efficient machine learning, probabilistic modeling and autonomous decision making with applications in climate/weather science, nuclear fusion, and robotics. In 2018, Marc received The President’s Award for Outstanding Early Career Researcher at Imperial College. He is a recipient of a Google Faculty Research Award and a Microsoft PhD Grant.
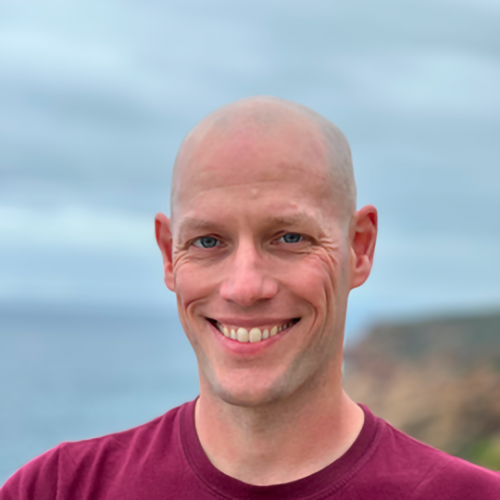
11:15 - 12:00
Transforming HydroMeteorology with Data Science: Algorithms, AI, and Practical Applications
Mengqian Lu, Hong Kong University of Science and Technology
Abstract
This presentation presents the application of data science and artificial intelligence to assistant and enhance hydrometeorological research. Our research utilizes data science and AI techniques to assist our understanding and improve predictions of complex hydrometeorological phenomena, including atmospheric rivers, mesoscale convective systems, and tropical storms, thereby facilitating more informed decision-making.
I will present case studies highlighting our team‘s development of algorithms and databases for analyzing and projecting atmospheric phenomena as climate continues to warm up, particularly focusing on atmospheric rivers – these represent key examples of organized and intensive moisture transport. Additionally, I will discuss our recent advancements in AI-driven downscaling techniques, used to project tropical storm risks and compare various state-of-the-art AI models.
The talk will encourage a broader discussion on how climate scientists can effectively integrate emerging data science tools into scientific exploration, addressing the challenges of navigating an increasingly complex array of available technologies. Furthermore, we will explore how modern algorithms and AI are reshaping hydrometeorology, discussing novel tools and insights for us to integrate into our ongoing work.
Biography
Mengqian received her Ph.D. from Columbia University in New York in 2014 and is currently an Associate Professor in the Department of Civil and Environmental Engineering at the Hong Kong University of Science and Technology. She will assume the role of Chairman of the International Association for Hydro-Environment Engineering and Research Hong Kong Chapter in September 2024. She is also the founder of the “Climate, Weather, and Water Resources Forum” and a core member of the technical organizing committee for the Hong Kong Climate Adaptation and Response Conference.
Mengqian’s research primarily focuses on the integration of hydrometeorology with artificial intelligence. Her research is at the forefront of meteorology, water resources, and climate risk, with a recent focus on the understanding and prediction of hydrometeorological extremes—such as heavy rainfall, floods, and tropical cyclones—owing to their significant societal impacts and the opportunity they present for pioneering engineering solutions. Her work is categorized into five principal areas: Atmospheric Rivers, Moisture Recycling Networks, East Asian Monsoon, Extreme Weathers, and Water Resources Management. She has published 38 papers in top journals, of which 7 have been selected as featured articles by various journals, highlighted by the American Geophysical Union, or reported in mainstream magazines. In 2023, Mengqian received the Asian International Innovation and Invention Gold Award for creating a one-stop hub for a thriving data ecosystem.
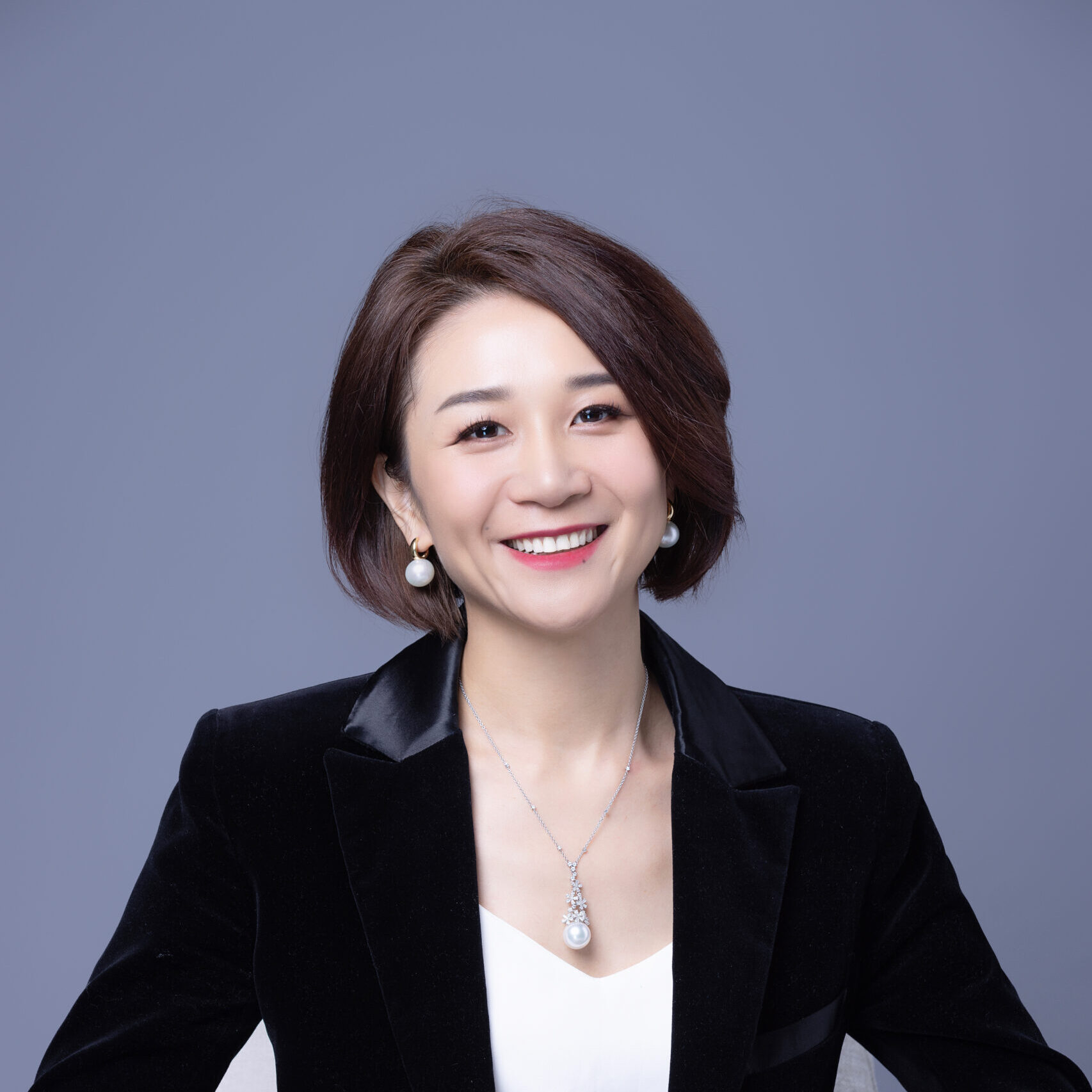
12:00 - 13:30
Lunch
13:30 - 15:00
Q&A and closing
15:00 - 15:30
Coffee
Organizing Committee
Fredrik Lindsten
Associate Professor
Linköping University
Michael Felsberg
Professor
Linköping University
Natascha Kljun
Professor
Lund University
Johan Lindström
Senior Lecturer
Lund University