Presentations from the workshop
Sara Ek
Spatially guided omics analyses of the analyses of the tumourtumourmicroenvironmentmicroenvironment
Karin Leandersson
Myeloid suppressor cells in human breast cancer
Kevin Litchfield
Non-classical sources of tumour specific antigen in checkpoint inhibitor response
Torkel Loman
How bacteria generate beneficial phenotypic heterogeneity through mixed positive/negative feedback loops
Adam L. MacLean
Modeling tumor-immune dynamics with stochastic delay differential equations
Chris Rackauckas
Data-Efficient Model Discovery with Scientific Machine Learning
Thomas Schön
Formulating exible probabilistic models
Giovanni Visonà
Getting personal with epigenetics: Towards machine-learning-assisted precision epigenomics
Reflections from the Focus Period
Some thoughts from the organizers of the Lund 2022 focus period and workshop
Sonja Aits, Kristian Soltesz and Eva Westin organized the ELLIIT focus period Lund 2022. Here are their thoughts on the event.
The views of three Lund University participants on the focus period and workshop
Karin Leandersson, Lavanya Lokhande and Jesper Sundell all participated to the ELLIIT focus period in Lund, 2022. They have now returned to their usual occupations with new scientific ideas, connections and plans for the future.
Some quick questions to invited speakers and focus period participants
The ELLIIT focus period in Lund 2022 has just finished. Participants from different fields of study and countries met in Lund to discuss data-driven modelling and learning for cancer immunotherapy.
Pictures from ELLIIT Focus period 2022 in Lund
Detailed program
May 3, 2022
17:00 - 19:00
Welcome reception
An opportunity to meet up before the workshop, and to find company for dinner.
Day 1 – May 4, 2022
08:45 - 09:15
AF-borgen
Opening
Annika Olsson, Dean of the Faculty of Engineering & Anders Rantzer, ELLIIT Focus Period Coordinator
09:15 - 10:00
Dynamical aspects of antigen recognition, tumor/immune/drug interactions, and spontaneous versus induced evolution of drug resistance during cancer treatment
Eduardo Sontag, University ECE and BioE
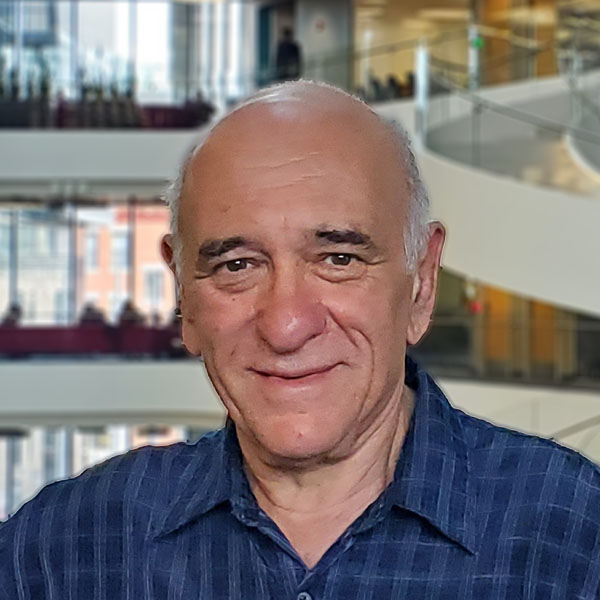
Abstract
This talk will consist of three related parts. The first part (Sontag, Cell Systems, 2017) addresses dynamic pathogen recognition. Since the early 1990s, many authors have independently suggested that self/nonself recognition by the immune system might be modulated by the rates of change of antigen challenges. This work introduces an extremely simple and purely conceptual mathematical model that allows dynamic discrimination of immune challenges. The main component of the model is a motif which is ubiquitous in systems biology, the incoherent feedforward loop, which endows the system with the capability to estimate exponential growth exponents, a prediction which is consistent with experimental work showing that exponentially increasing antigen stimulation is a determinant of immune reactivity. Combined with a bistable system and a simple feedback repression mechanism, an interesting phenomenon emerges as a tumor growth rate increases: elimination, tolerance (tumor growth), again elimination, and finally a second zone of tolerance (tumor escape). This prediction from our model is analogous to the “two-zone tumor tolerance” phenomenon experimentally validated since the mid 1970s. Moreover, we provide a plausible biological instantiation of our circuit using combinations of regulatory and effector T cells.
The second part of the talk will be based mostly upon (Greene, Gevertz, Sontag, ASCO JCO Clinical Cancer Informatics, 2019). It deals with the following topic. Resistance to chemotherapy is a major impediment to the successful treatment of cancer. Classically, resistance has been thought to arise primarily through random genetic mutations, after which mutated cells expand via Darwinian selection. However, recent experimental evidence suggests that the progression to resistance need not occur randomly, but instead may be induced by the therapeutic agent itself. This process of resistance induction can be a result of genetic changes, or can occur through epigenetic alterations that cause otherwise drug-sensitive cancer cells to undergo “phenotype switching”. This relatively novel notion of resistance further complicates the already challenging task of designing treatment protocols that minimize the risk of evolving resistance. In an effort to better understand treatment resistance, we have developed a mathematical modeling framework that incorporates both spontaneous and drug-induced resistance. Our model demonstrates that the ability (or lack thereof) of a drug to induce resistance can result in qualitatively different responses to the same drug dose and delivery schedule. The importance of induced resistance in treatment response led us to ask if, in our model, one can determine the resistance induction rate of a drug for a given treatment protocol. Mathematically, we show that the induction parameter in our model is theoretically identifiable, and we also provide a solution to an associated optimal control (Greene, Sanchez-Tapia, Sontag, Frontiers in Bioengineering and Biotechnology, 2020).
The last part of the talk is based on (Tran, Al-Radhawi, Kareva, Wu, Waxman, E.D. Sontag, Frontiers in Immunology, 2020, and paper in preparation with Tran, Sadeghi, et al.). The motivation for this work was the understanding of certain aspects of “metronomic chemotherapy”, which is believed to drastically enhance immunogenic tumor cell death, through mechanisms that are still incompletely understood. We developed a mathematical model to elucidate the underlying complex interactions between tumor growth, immune system activation, and therapy-mediated immunogenic cell death. Our model is conceptually simple, yet it provides a surprisingly excellent fit to empirical data obtained from GL261 mouse gliomas treated with cyclophosphamide on a metronomic schedule. The model includes terms representing immune recruitment as well as the emergence of drug resistance during prolonged metronomic treatments. Strikingly, a fixed set of parameters, not adjusted for individuals nor for drug schedule, excellently recapitulates experimental data across various drug regimens, including treatments administered at intervals ranging from 6 to 12 days, and immune activation data. The mathematical model suggests that immunostimulatory and immunosuppressive intermediates are responsible for the observed phenomena of resistance and immune cell recruitment, and thus for variation of responses with respect to different schedules of drug administration. Using optimal control tools, we then obtain a “mathematically derived optimal regimen” (MDOR). For this mouse model, we find that the MDOR that minimizes tumor size after 60 days is neither a classical metronomic schedule nor a maximum tolerated dose (MTD) schedule. Instead, our MDOR consists of administering a lower dose at the beginning of treatment, followed by a larger dose after 35 days. The discovered regimen is analogous to the “press-pulse’” theory in ecology, in which frequent and expansive mass species extinctions occur after “press’” disturbances (such as climate or sea level change) weaken and destabilize populations over many generations, and are then followed by a “pulse” disturbance that causes extensive mortality.
Biography
Eduardo Sontag’s major current research interests lie in several areas of control and dynamical systems theory, systems molecular biology, synthetic biology, computational biology, and cancer and immunology
He received his Licenciado degree from the Mathematics Department at the University of Buenos Aires in 1972, and his Ph.D. (Mathematics) under Rudolf E. Kalman at the University of Florida, in 1977. From 1977 to 2017, he was with the Department of Mathematics at Rutgers, The State University of New Jersey, where he was a Distinguished Professor of Mathematics as well as a Member of the Graduate Faculty of the Department of Computer Science and the Graduate Faculty of the Department of Electrical and Computer Engineering, and a Member of the Rutgers Cancer Institute of NJ. In addition, Dr. Sontag served as the head of the undergraduate Biomathematics Interdisciplinary Major, Director of the Center for Quantitative Biology, and Director of Graduate Studies of the Institute for Quantitative Biomedicine. In January 2018, Dr. Sontag was appointed as a University Distinguished Professor in the Department of Electrical and Computer Engineering and the Department of BioEngineering at Northeastern University. Since 2006, he has been a Research Affiliate at the Laboratory for Information and Decision Systems, MIT, and since 2018 he has been a member of the Faculty in the Program in Therapeutic Science at Harvard Medical School.
Eduardo Sontag has authored over five hundred research papers and monographs and book chapters in the above areas with over 47,000 citations and an h-index of 97. He is in the Editorial Board of several journals, including: Cell Systems, IET Proceedings Systems Biology, Synthetic and Systems Biology, International Journal of Biological Sciences, and Journal of Computer and Systems Sciences, and is a former Board member of SIAM Review, IEEE Transactions in Automatic Control, Systems and Control Letters, Dynamics and Control, Neurocomputing, Neural Networks, Neural Computing Surveys, Control-Theory and Advanced Technology, Nonlinear Analysis: Hybrid Systems, and Control, Optimization and the Calculus of Variations. In addition, he is a co-founder and co-Managing Editor of the Springer journal MCSS (Mathematics of Control, Signals, and Systems).
He is a Fellow of various professional societies: IEEE, AMS, SIAM, and IFAC, and is also a member of SMB and BMES. He has been Program Director and Vice-Chair of the Activity Group in Control and Systems Theory of SIAM, and member of several committees at SIAM and the AMS, including Chair of the Committee on Human Rights of Mathematicians of the latter in 1981-1982. He was awarded the Reid Prize in Mathematics in 2001, the 2002 Hendrik W. Bode Lecture Prize and the 2011 Control Systems Field Award from the IEEE, the 2002 Board of Trustees Award for Excellence in Research from Rutgers, and the 2005 Teacher/Scholar Award from Rutgers.
10:00 - 10:30
Coffee break
10:30 - 11:15
Systems-level immunomonitoring in children with cancer
Petter Brodin, Karolinska institutet
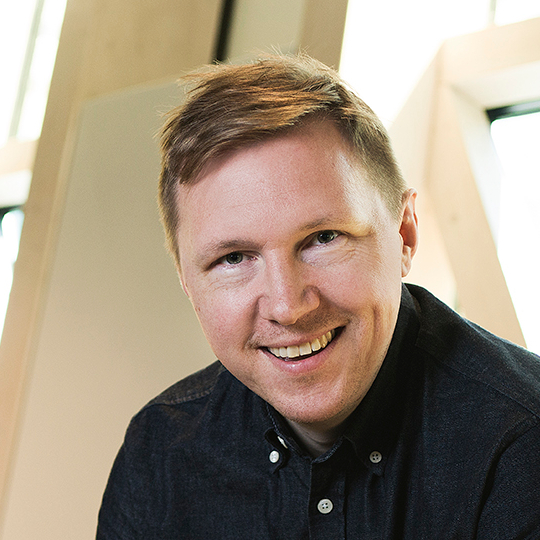
Abstract
The field of cancer immunotherapy has advanced in recent years and impressive results have been reported. Children with solid tumours have not benefitted as much from these advances as adults and paediatric tumours are often considered non-immunogenic, a view that now slowly changing. To enable immunotherapies in children with solid tumours, a better understanding of systemic immune responses is required. Findings made in adult patients cannot be directly translated given stark differences in immune composition and function between adults and children. We have recently uncovered several important aspects of immune system development early in life and inspired by these we have launched, the Immune system Against cancer in Children, (ISAC) study at the Karolinska University Hospital. Since March 2018, 217 patients with solid tumors have been enrolled and 4,549 samples have been collected from these. Here I will report our results from state of the art systems-level immunomonitoring combining Mass cytometry, plasma protein profiling, mRNA- and T- and B-cell receptor sequencing, to characterize the immune system states before, during and after treatment, investigate predictors of clinical outcome and evidence of tumour-specific immune responses.
Biography
Petter Brodin is professor and specialist physician at Karolinska Institutet where he is the leader of the Brodinlab. His team is aiming to understand human immune system variation in health and disease, and understand the factors that shape human immune systems. The team is also interested in defining better metrics of immune system health and develop methods for better immune system analyses in human patients.
As a physician at the department of pediatrics at the Karolinska University Hospital, Petter has a particular interest in understanding when and how human immune systems are shaped early in life, and the influences by environmental exposures such as the microbiome, infections, vaccines, nutritional components etc in this process.
Petter’s team is firmly convinced that to understand human immune systems and their variation it is essential that all system components are analyzed simultaneously and relationships between these taken into account. This have recently been made possible with the development of novel high-dimensional methods operating at single-cell resolution, such as Mass cytometry, mRNA-sequencing and integrative multiomics analyses. To analyze human immune systems at the systems-level, the team is developing novel experimental and computational methods.
11:15 - 12:00
How bacteria generate beneficial phenotypic heterogeneity through mixed positive/negative feedback loops
(replaces Immunotherapy in cancer treatment)
)
Torkel Loman, University of Cambridge)
(replaces Ana Carneiro, Lund University)
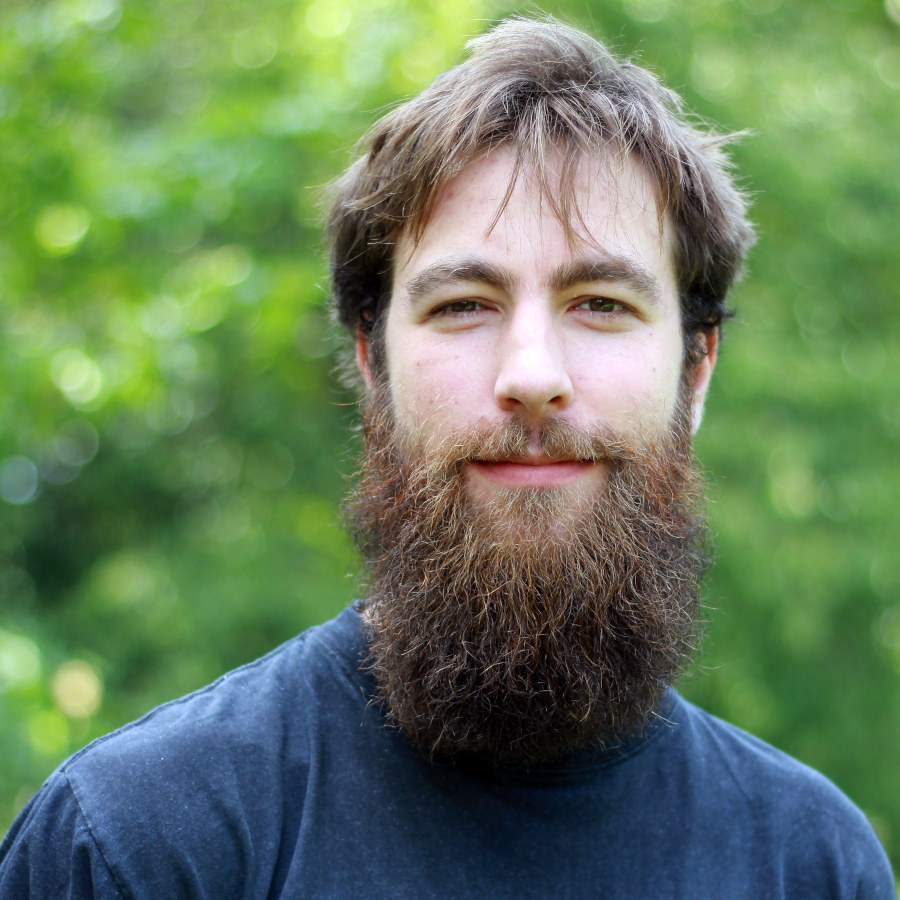
Abstract
Bacterial sigma factors (a class of transcription factors) are prominent regulators of stress response. Understanding sigma factor behaviours is important in settings where we wish to kill bacteria (e.g. food decontamination and disease treatment) through the application of some stress (e.g. heat or antibiotics). Every species typically maintain several different types of sigma factors, each governing a specific stress response. Through single-cell fluorescent microscopy studies, it has been noted that sigma factor responses often are highly heterogeneous, even across isogenic populations. It has been suggested that this is a part of a bet-hedging strategy, whereby displaying a varied response, the population can optimally adapt itself to future uncertainty. We have studied two different sigma factor systems in Bacillus subtilis, each displaying a distinct form of response heterogeneity. By combining stochastic mathematical modelling of their regulatory pathways with experimental measurements of their response activities, we explain how the two responses can be generated through the amplification of natural biochemical noise. Next, we note that most sigma factor circuits contain a mixed positive/negative feedback loop. Through a general model of such a circuit, we show how this motif can generate a range of different response behaviours (including both previously observed, and novel, ones). We show how the model’s parameters (i.e. the system’s biological properties) affect which behaviour is produced. This demonstrates a general mechanism to generate phenotypic heterogeneity, which can also be generalised to non-sigma factor systems containing mixed positive/negative feedback loops.
Biography
Torkel Loman is a final year PhD student at the Sainsbury Laboratory, at the University of Cambridge. In his research is uses mathematical models to study sensory pathways in bacteria, while also working in close cooperation with experimentalists. The primary focus is stochastic models and the investigation of how intrinsic cellular noise can be harnessed by the bacteria to create advantageous phenotypes. He is also a codeveloper of the Catalyst.jl chemical reaction network modelling package. Torkel has previously studied at the programs for engineering mathematics, molecular biology, and bioinformatics at Lund University.
12:00 - 13:00
Lunch and guided tour of Lundagård
Guided tour of Lundagård (half group); lunch (half group)
13:00 - 13:45
Lunch and guided tour of Lundagård
Guided tour of Lundagård (half group); lunch (half group)
13:45 - 14:30
Non-classical sources of tumour specific antigen in checkpoint inhibitor response
Kevin Litchfield, UCL Cancer Institute London
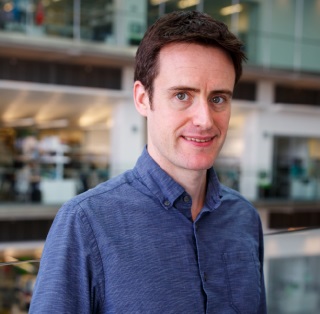
Abstract
Biomarkers associated with immune checkpoint inhibitor (CPI) response can be broadly categorised into four categories: sources of antigen leading to T-cell responses, host-derived factors, immune evasion and infiltration mechanisms. Our published meta-analysis of biomarkers included over 1000 patients (CPI1000 cohort) across multiple cancer types. These analyses established clonal tumour mutation burden (TMB) as the strongest predictor of CPI response (p=1.7×10-12) alongside TMB and CXCL9. However, over half of the patients in our cohort who respond to CPI treatment (52%) lacked high TMB and showed no evidence of an oncogenic viral infection, hence suggesting alternative sources of antigen are all important drivers of immune response. Alternative sources of antigen can originate from a diverse range of biological processes including: i) retained introns, ii) mutations which trigger upstream open reading frames (i.e., mutated peptides from the 5’ UTR translation), iii) stop codon loss mutations (i.e., mutated peptides from 3’ UTR translation), iv) intronic polyadenylation events), v) expression of endogenous retroviral elements, vi) cancer associated bacterial/viral epitopes and vii) dinucleotide variants. Data from this exploratory analysis will be coupled with peptide T-cell reactivity assays, to validate immunogenicity in local UCL patient TIL/PBMC samples. Here we will present latest insights from CPI1000+ and highlight the importance of emerging non-classical courses of tumour specific antigen.
Biography
Kevin trained in mathematics and bioinformatics, worked in the pharmaceutical industry at Novartis Oncology, completed a PhD in cancer bioinformatics, and recently completed his postdoctoral training with Prof. Charles Swanton at the Francis Crick Institute. Kevin is now a group leader at UCL Cancer Institute London , specialising in immune-oncology biomarker development and drug target identification.
14:30 - 15:00
Faster Single Cell Analysis
Rasmus Henningsson. Lund University
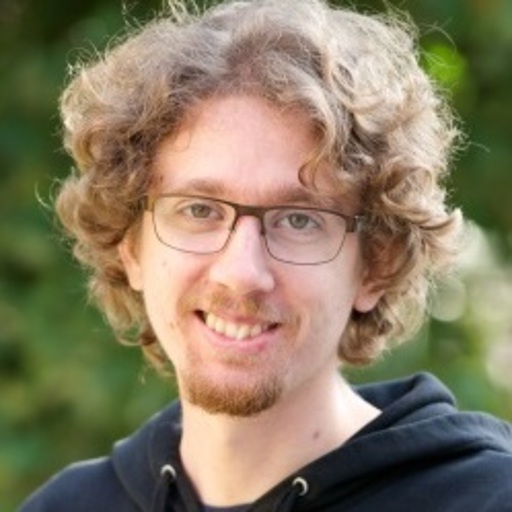
Abstract
Using Single Cell RNA Sequencing, it is today possible to generate data sets with gene expression levels for 30k genes in hundreds of thousands of cells. Explorative analysis of these rich data sets is important – but challenging using existing tools that are slow and require lots of RAM (even if many genes are thrown out). We show that even though the normalized data matrix is not sparse, we can still exploit the sparsity of the original data matrix to vastly reduce RAM usage and runtimes. In particular, we compute the truncated SVD of the normalized data matrix, which serves as noise reduction, dimension reduction and a starting point for downstream analysis.
Biography
Rasmus Henningsson’s research interests are centered around high-dimensional biological data in general and Leukemia in particular. He is currently developing new methods for dimension reduction, analysis and visualization of single cell RNA-seq data. He got his PhD degree in applied mathematics at Lund University in 2018, working on dimension reduction, viral evolution and Leukemia.
15:00 - 15:30
Getting personal with epigenetics: towards machine-learning-assisted precision epigenomics
Giovanni Visona, Max Planck Institute for Intelligent Systems Tübingen
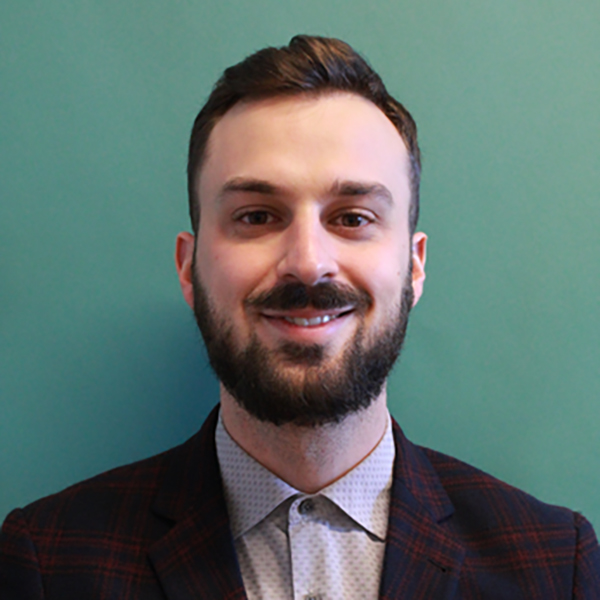
Abstract
Epigenetic modifications are dynamic control mechanisms involved in the regulation of gene expression. Unlike the DNA sequence itself, they vary not only between individuals but also between different cell types of the same individual. Exposure to environmental factors, somatic mutations, and ageing contribute to epigenomic changes over time, which may constitute early hallmarks or causal factors of disease. Epigenetic changes are reversible and, therefore, promising therapeutic targets. However, mapping efforts to determine an individual’s cell-type-specific epigenome are constrained by experimental costs. We developed eDICE, an attention-based deep learning model, to impute epigenomic tracks. In addition to benchmarking
results against state of the art models, I will present a proof of concept for the imputation of personalised epigenomic measurements on the ENTEx dataset, where eDICE correctly predicts individual- and cell-type-specific epigenetic patterns.
Biography
Giovanni graduated in medical physics at the University of Trent (Italy), and moved to machine learning with an MSc at the University of Edinburgh. He joined the Max-Planck Institute for Intelligent Systems in Tübingen at the end of 2019 for a PhD, and is now a part of the Marie Curie Innovative Training Network entitled “Machine Learning Frontiers in Precision Medicine”. In his work Giovanni focus on the application of deep learning and representation learning models to biomedical data, with a particular interest for sequencing data.
15:30 - 16:30
Coffee break and round table discussions
Day 2 – May 5, 2022
9:15 - 10:00
AF-borgen
Spatially guided molecular omics analyses of the tumour immune microenvironment
Sara Ek, Lund University
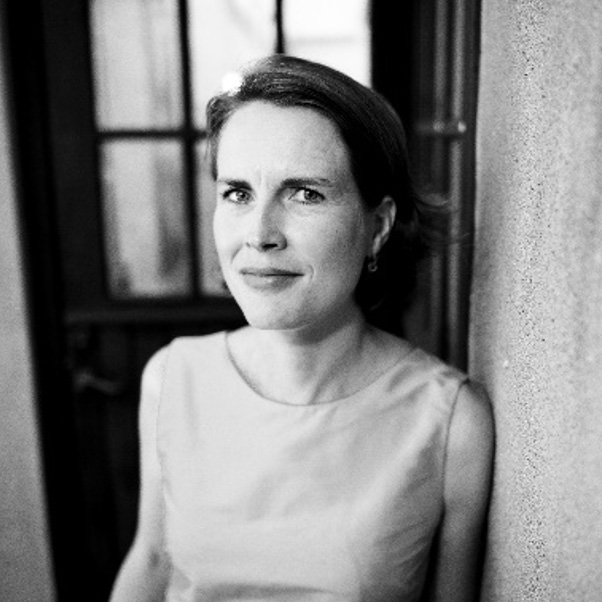
Abstract
Within the era of precision medicine, drugs are developed based on deep and precise biological understanding of disease, and the molecular variation between patients. As an example, immune-oncology drugs have revolutionized cancer care, and provided hope for patients with severe disease. However, the response to treatment with for example check-point inhibitors that release the break on the immune system, is difficult to predict using single biomarkers.
To fully take advantage of targeted treatment, more advanced companion diagnostic tools need to be developed that can predict therapeutic response and allow optimal patient stratification.
Recent development of spatially resolved omics technologies have drastically improved our capability to gain such insight. Cellular crosstalk and tissue heterogeneity can be studied in detail in an unprecedented manner, in large cohort of patients. The technology leap opens the possibility to investigate molecular data with retained spatial information.
So far, bioinformatic methods that allow integration of high-plex mRNA and protein data, clinical data and take the spatial-localisation and proximity of sampled cells into account are lacking. We intend to fill this gap by our ongoing research efforts.
Biography
Sara Ek is a Professor in Immunotechnology at Lund University. She has a keen interest in translational cancer research and with a specific focus of lymphoid malignancies and patient stratification for improved precision medicine efforts. She is the initiator and director of the SpatialOmics@LU facility in Lund and drive development of omics analyses of spatially resolved molecular data. She is engaged in strategic development of research at Lund University through her engagement in the Lund University Cancer Centre Board and the Faculty of Engineering research board. She is one of the co-founders of Immunovia, a company with a commercially available test, for early and non-invasive detection of pancreatic cancer. She is further heading two EU- MSCA Cofund doctoral/post-doctoral programs, the CanFaster programs, that are dedicated to translation of cancer research into clinically useful innovations.
10:00 - 10:30
Coffee
10:30 - 11:15
Computational modeling in Systems Immunology
Magnus Fontes, Institut Roche
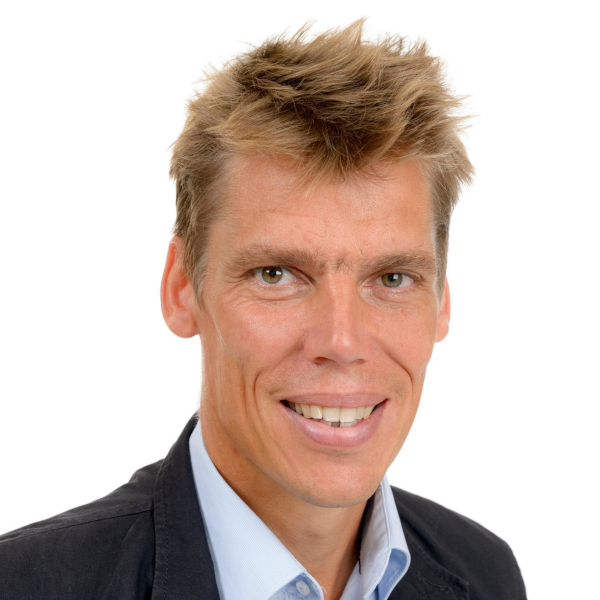
Abstract
I will describe current and high value challenges and opportunities for mathematical modeling in Cancer Immunology.
Biography
Magnus Fontes is PhD in Mathematics and an expert in bioinformatics, machine learning and mathematical & statistical modeling focused on the analysis and visualization of complex biomedical systems. His publications over the last decades cover cancer and infectious disease building on novel theoretical mathematical methods and algorithms. Fontes’industrial and academic experience include e.g.
Co-founder and member of the board of the bioinformatics software company Qlucore (www.qlucore.com)
- 2003-2014:Head of the Centre for Mathematical Sciences at Lund University, Lund, Sweden.
- 2014-2017:Director at the Centre for Bioinformatics,Biostatistics and Integrative Biology (C3BI), Institut Pasteur, Paris, France.
- 2018-2020 PrincipalScientist and Head of Systems Cancer Immunology, Cancer Immunology, Genentech, South San Francisco, CA, USA.
- 2020- General Manager of Institut Roche in Paris, France.
11:15 - 12:00
Data-Efficient and Model Discovery with Scientific Machine Learning
Chris Rackauckas, MIT
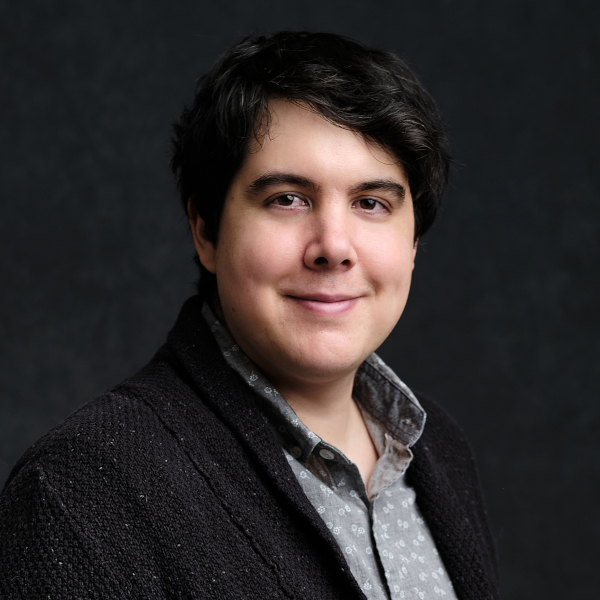
Abstract
Scientific machine learning (SciML) methods allow for the automatic discovery of mechanistic models by infusing neural network training into the simulation process. In this talk we will start by showcasing some of the ways that SciML is being used, from discovery of extrapolatory epidemic models to nonlinear mixed effects models in pharmacology. From there, we will discuss some of the increasingly advanced computational techniques behind the training process, focusing on the numerical issues involved in handling differentiation of highly stiff and chaotic systems. The viewers will leave with an understanding of how compiler techniques are being infused into the simulation stack to increasingly automate the process of developing mechanistic models.
Biography
Chris’ research is Scientific Machine Learning (SciML): the integration of domain models with artificial intelligence techniques like machine learning. By using the structured scientific (differential equation) models together with the unstructured data-driven models of machine learning, our simulators can be accelerated, our science can better approximate the true systems, all while enjoying the robustness and explainability of mechanistic dynamical models.
Chris is the lead developer of the DifferentialEquations.jl solver suite along with hundreds of other Julia packages, earning him the inaugural Julia Community Prize and front page features on many tech community sites. Chris’ work on high performance differential equation solving is the engine accelerating many applications from the MIT-CalTech CLiMA climate modeling initiative to the SIAM Dynamical Systems award winning DynamicalSystems.jl toolbox. He is the lead developer of JuliaSim, where the work is credited for the 15,000x acceleration of NASA Launch Services simulations and recently demonstrated a 60x-570x acceleration over Modelica tools in HVAC simulation. For these achievements Chris received the US Air Force Artificial Intelligence Accelerator Scientific Excellence Award. As lead developer of Pumas, he received the ISoP Mathematical and Computational Special Interest Group Award at the American Conference of Pharmacology (ACoP) 2019 for his work on improved clinical dosing via Koopman Expectations, along with the ACoP 2020 Quality Award for his work with Pfizer on GPU-accelerated quantitative systems pharmacology to accelerate preclinical analysis by 175x. Notably, Moderna adopted Pumas in 2020 to accelerate crucial clinical trials, noting “Pumas has emerged as our ‘go-to’ tool for most of our analyses in recent months”. For these achievements, Chris received the Emerging Scientist award from ISoP, the highest early career award in pharmacometrics.
12:00 - 13:00
Lunch
13:00 - 13:45
The Acute Myeloid Leukemia Stealth Mode Hypothesis
Thoas Fioretos, Lund University
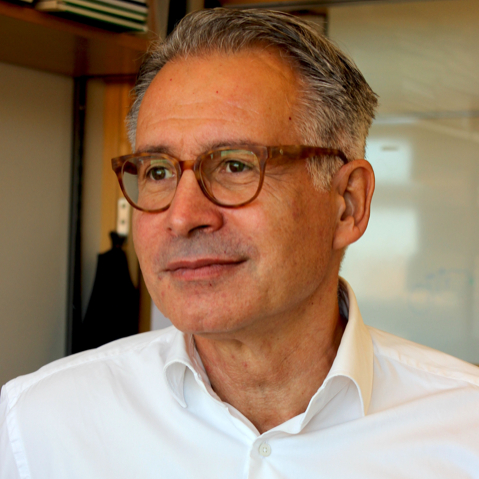
Abstract
Acute myeloid leukemia (AML) evolves in a step-wise fashion and in a very hostile environment surrounded by the immune system. In contrast to solid tumors originating at distant sites, AML stem cells need to launch multiple defense systems to escape the immune cells surrounding them already at their initial stage of development. I will argue that by studying AML as a model system, critical insights can be made on how cancer in general manage to evade the attack by the immune system.
Biography
Thoas Fioretos is a professor and senior consultant at the Department of Clinical Genetics, Lund University, Sweden. He is also the Director of national Clinical Genomics platform at SciLifeLab, a national facility offering expertise and service in next-generation sequencing for clinical research and developing new diagnostic assays for improved precision diagnostics. Professor Fioretos is also a recipient of a Wallenberg Clinical Scholar. His research is focused on translating genomic discoveries in acute leukemia into improved clinical decision-making and new therapies. Fioretos is a founding member of Genomic Medicine Sweden, aiming at implementing genomics-based precision medicine in Sweden, and is the co-coordinator of the Hematology Working Group within GMS. He has published >120 research articles, many in top tier journals, and is an Associate Editor of Genes Chromosomes and Cancer.
Fioretos has co-founded two spin-out companies active in bioinformatics (Qlucore AB) and antibody-based therapies of cancer (Cantargia AB), respectively.
13:45 - 14:30
Emergence and Robustness of chaos in deterministic systems
Artur Avila
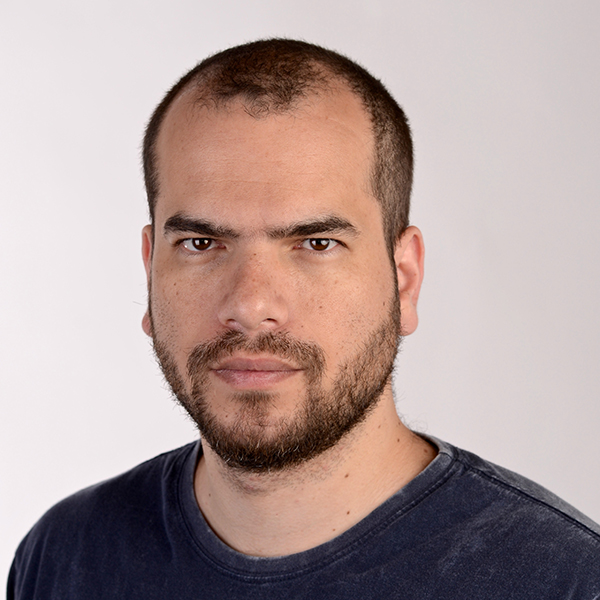
14:30 - 15:00
Coffee
15:00 - 15:45
Heat Kernel and embedding of metric spaces in Hilbert spaces
Gerard Besson
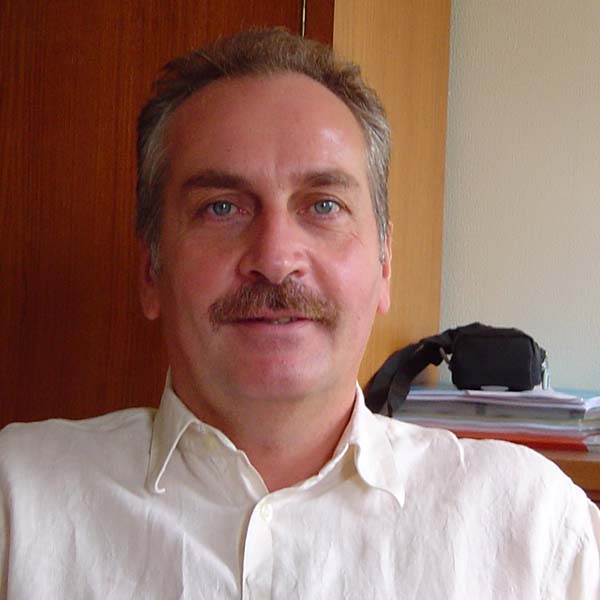
Abstract
We shall present a technique developped by P. Bérard, S. Gallot and myself in1995 to view a large family of closed manifolds as subsets of a canonical Hilbert space. This gives rise to a distance function between closed manifolds which we called “spectral distance”. It has been extended to (resonable) compact metric spaces and we then can explore the possible applications to data analysis. The talk is intended to be, as much as possible, mathematically light.
Biography
CURRICULUM VITAE
G. Besson
BESSON G erard
4, rue Mar echal Dode, 38000 Grenoble, France.
O ce tel : +33 476514851
Born on december 13th, 1955.
Married, 3 children.
Ecole Normale Sup erieure de St Cloud, 1974-79.
Agr egation, Mathematics, July 1977.
PhD Thesis (Th ese de 3 eme cycle), July 1979
My advisor was Marcel Berger and the title : \\Sur la multiplicit e de la
premi ere valeur propre des surfaces riemanniennes” .
Military Service, August 1979 – November 1980.
Research Fellow at the Ecole Polytechnique, March 15th, 1981 –
September 14th, 1981.
Hired at C.N.R.S. as Attach e de Recherche September 15th, 1981.
Position at Institut Fourier de Grenoble, Universit e Grenoble Alpes.
Promoted Charg e de Recherche, 1st class, September 1985.
Visiting professor at the University of Pennsylvania (Philadel-
phie). Acadamic year 1985-86.
Th ese d’Etat, Habilitation defended on July 2nd, 1987.
Courses at Ecole Nationale des Techniques Avanc ees (Paris). In
this ingeneering school I gave several courses on the study of the di eomor-
phisms of the circle.
Professor at Ecole Polytechnique from September 1st, 1993 until
August 31st, 2006. This is a temporary position which I held in complement
of my position at C.N.R.S in Grenoble.
Promoted Directeur de Recherches, C.N.R.S. 2nd class, October
1996.
Promoted Directeur de Recherches, C.N.R.S. 1st class, October
2005.
Alexandre Johannid es prize of the (french) Academy of Sciences,
2006.
Support from the Clay Institute My collaborators and myself wrote
book on Perelman’s works, 2009.
Chairman of the Institut Fourier from January 1st, 2011 till December
31st, 2015.
Promoted Directeur de Recherches CNRS de classe exception-
nelle, 1er echelon, October 2011.
European Research Council (ERC) Advanced Grant for the pro-
gram “Geometry and Topology of Open Manifolds”, February 2013
– January 2018.
Promoted Directeur de Recherches CNRS de classe exception-
nelle, 2 eme echelon, October 2016.
18:00
19:00
Turning Torso
Bus from AF to Turning Torso
Dinner
Day 3 – May 6, 2022
9:15 - 10:00
Myeloid suppressor cells in human breast cancer
Karin Leandersson, Lund University
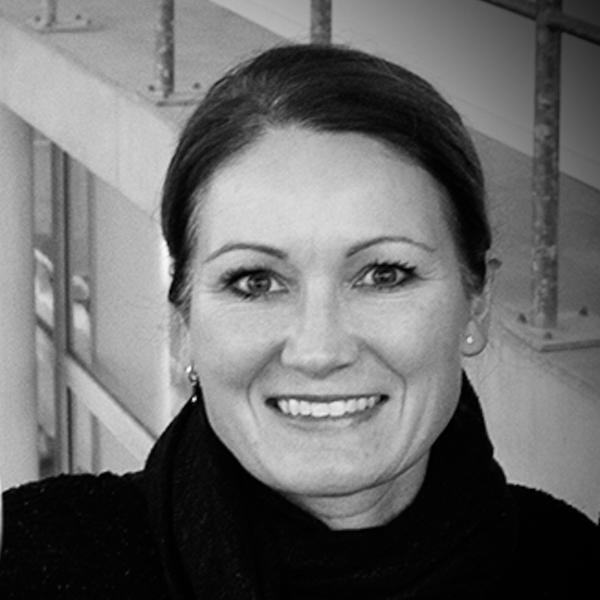
Abstract
The focus of this presentation is on myeloid innate immune cell populations, their effect on tumor progression and immune tolerance in cancer patients. Using translational approaches with clinical collaborations we have studied the principle behind innate systemic immune tolerance in breast cancer patients. Our studies range from novel molecular mechanisms regarding generation and function of myeloid derived suppressor cells, to resident lymph node macrophage subpopulations and their relation to lymphocyte activation. Our research is important for understanding why the innate- and thus adaptive immune response is suppressed in cancer patients, with the aim to reveal novel mechanisms for immunosuppression.
Biography
Karin Leandersson holds a M.Sc. in Biochemistry and a B.Sc. in Biology from the Natural Science faculty, Lund University, Sweden. She continued studying Immunology and received a PhD in Immunology at the Medical Faculty in 2002. During her PostDoc 2002-2007 she changed field now working on breast cancer and cancer biology. In 2007 Karin Leandersson became an Associate Professor in Immunology, and started her own group with a focus on human Tumor Immunology. Since 2017 Karin Leandersson is Professor in Human Tumor Immunology at the medical faculty Lund University, Sweden. Her research interest is the human immune system and its mutual interaction with cancer, with a focus on primary human tissues and immune cells, with an aim to be able to understand tumor immunology better in the human perspective.
10:00 - 10:30
Coffee
10:30 - 11:15
Formulating flexible probabilistic models
Thomas Schön, Uppsala University
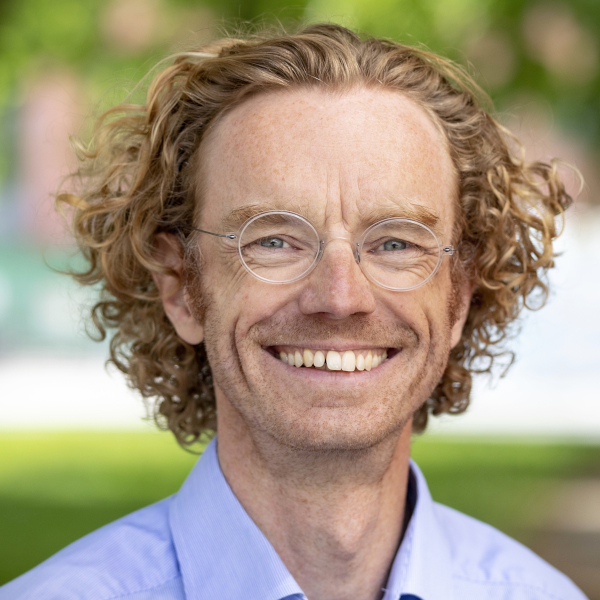
Abstract
One of the key lessons to take away from contemporary machine learning is that flexible models can offer the best predictive performance. This has implications in many situations. In this lecture I will try to make this concrete by looking at a few constructions that we are working with. I will start with a classification task from ECG interpretation and then continue to the more under-researched area of how to formulate and solve regression problems using deep learning. There are currently several different approaches used for deep regression and there is still room for innovation. I will illustrate this landscape in general and introduce our rather general deep regression method which has a clear probabilistic interpretation. This constructions provides good performance on several computer vision regression tasks, system identification problems and 3D object detection using laser data.
Biography
Thomas B. Schön is the Beijer Professor of Artificial Intelligence in the Department of Information Technology at Uppsala University. He has held visiting positions with the University of Cambridge (UK), the University of Newcastle (Australia) and Universidad Técnica Federico Santa Maria (Valparaiso, Chile). In 2018, he was elected to The Royal Swedish Academy of Engineering Sciences (IVA) and The Royal Society of Sciences at Uppsala. He received the Tage Erlander prize for natural sciences and technology in 2017 and the Arnberg prize in 2016, both awarded by the Royal Swedish Academy of Sciences (KVA). He is a fellow of the ELLIS society.
11:15 - 12:00
Combining text mining with high-content imaging to gain insight into lysosome function and cell death in cancer
Sonja Aits, Lund University
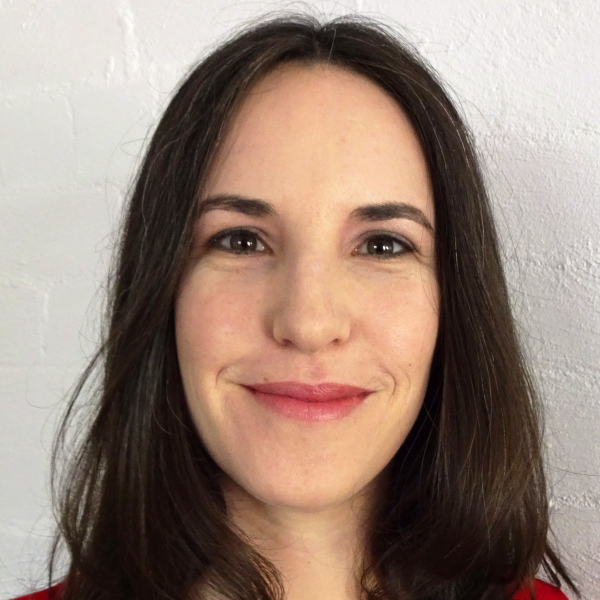
Abstract
Access to up-to-date medical knowledge is important for researchers, drug developers, health care staff and public health decision makers who naturally want to make evidence-based decisions. Unfortunately, the large number of texts that need to be read to extract this knowledge far exceeds the human capability. The problem is especially large when analyzing signaling pathways because pieces of knowledge that make sense only when combined are scattered over millions of research articles and patient records. Automated text mining, so-called natural language processing, with neural networks and dictionary-based systems can address this problem at least in part by extracting and connecting some types of information and highlighting texts that are of high priority to specific reader groups.
We combine this technology with other approaches, such as high-content imaging, to gain insights into the network of interconnected signaling pathways that control cell death, autophagy and lysosome function. These signaling pathways and cell functions are disrupted in practically all cancers and many other diseases. Lysosome disruption also critically affects the immune systems as lysosomes play a key role in antigen presentation, immune checkpoint regulation and lineage differentiation of immune cells.
Biography
Sonja Aits is a cancer cell biologist, specialized in cell death and lysosomes, who has during recent years focused on the development and application of machine learning tools for medical research. She obtained a PhD in Biomedicine from Lund University in 2010 and then worked 6 years as Postdoc in the group of Marja Jäättelä at the Danish Cancer Society Research Center in Copenhagen. After that, she spent 1.5 years as Visiting Senior Scientist in the group of Ricky Johnstone at the Peter MacCallum Cancer Centre in Melbourne, where she conducted a large genome-wide high-content microscopy screen. In 2018, she returned to Lund University as head of the “Cell Death, Lysosomes and Artificial Intelligence ” group at the Department of Experimental Medical Science. Her group develops deep learning tools for the analysis of medical data, primarily computer vision and natural language processing models, and combines them with “classical” bioinformatics approaches to elucidate lysosome- and cell death-related signaling networks and their disruption by diseases and environmental toxins. Her group has also part of the SciLifeLab/KAW National COVID-19 Research Program, developing tools for text mining of electronic health records and medical literature and for lung histology analysis. Besides her research, Sonja is one of the coordinators of AI Lund (https://www.ai.lu.se/), which organizes a wide range of AI-related activities for its over 2000 academic, industry and public sector employees, and study director of Lund University’s COMPUTE research school (https://www.compute.lu.se/). She has also developed a range of successful PhD courses within “AI for Medicine and Life Science” and is very engaged in public outreach.
LU researcher page: https://portal.research.lu.se/en/persons/sonja-aits
LinkedIn: https://www.linkedin.com/in/sonjaaits/
Twitter: https://twitter.com/AitsLab
Youtube: https://www.youtube.com/Aitslab
12:00 - 13:00
Lunch
13:00 - 13:45
Modeling stochastic tumor-immune dynamics for the design of new therapeutics
Adam MacLean, University of Southern California
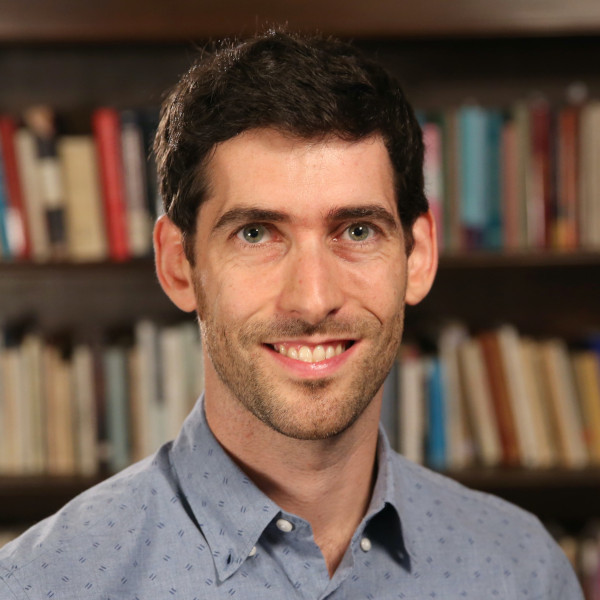
Abstract
Myeloid-derived suppressor cells (MDSCs) are pathologically activated innate immune cells with potent immunosuppressive activity. Their role in the tumor immune microenvironment is important and under-appreciated; their presence can significantly worsen outcomes in primary and metastatic disease. Informed by data from a phase I clinical trial for metastatic breast cancer, we have developed mathematical models of tumor-immune dynamics while undergoing treatment with combinations of a histone deacetylase inhibitor, and anti-PD-1 and anti-CTLA-4 therapies. Single-cell RNA-sequencing data were used to map the composition of the tumor-immune microenvironment under different treatment conditions, where subtle yet important shifts in immune populations were observed. Crucially, previous models have neglected the effects of MDSC on tumor response, including the observed recruitment/activation delays. Here we developed models of the tumor-immune dynamics using stochastic delay differential equations. Sensitivity analyses revealed the importance of MDSC parameters in controlling responses. We used clinical data on tumor response outcomes (RECIST) to fit individual patient models via Bayesian parameter inference, enabling the characterization of patient-specific responses to combination therapies during metastatic breast cancer. Finally, through the combination of dynamical systems modeling and machine learning, we are developing new tumor biomarkers of immune sensitization with which we can assess therapeutic responses in the clinic.
Biography
Adam MacLean is an Assistant Professor in the Department of Quantitative and Computational Biology at the University of Southern California. Adam studied mathematical physics at the University of Edinburgh, then completed his PhD in systems biology from Imperial College London. He worked as a postdoc at the University of Oxford and the University of California, Irvine, before joining USC in 2019. Adam’s lab at USC seeks to understand how cell fate decisions regulate tissues and organs during development, homeostasis, and cancer. These questions are addressed via the development of new mathematical models and machine learning tools, drawing on recent biological advances in single-cell measurement technologies, and computational advances in Julia for numerical systems biology. His work has appeared in PNAS, Stem Cells, and Cancer Research. He has won multiple fellowships and awards, including an NSF CAREER Award in 2021.
13:45 - 14:30
Learning endogenous T cell dynamics during cancer immunotherapy
Vanessa Jonsson
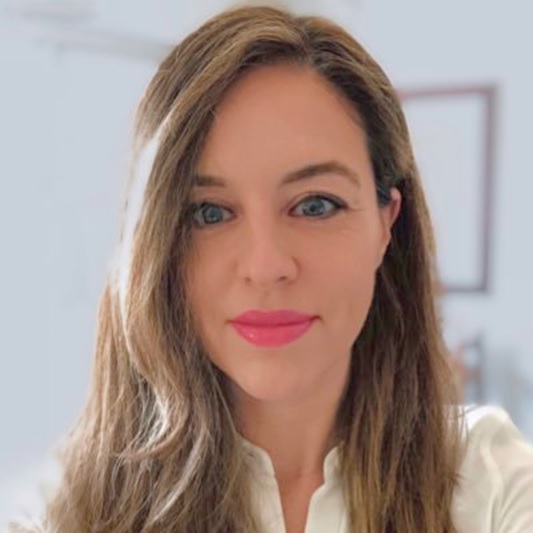
Abstract
CAR T cell therapy has transformed clinical care and management of patients with certain hematological cancers. However, it remains unclear whether the success of CAR T cell therapy relies solely on CAR T cell engagement with tumor antigen, or if it also requires the stimulation of an individual patient’s endogenous T cell response. We performed combined analysis of longitudinal, single cell RNA and T cell receptor sequencing on glioblastoma tumors, peripheral blood (PB), and cerebrospinal fluid (CSF) from a patient with recurrent multifocal glioblastoma that underwent a remarkable response followed by recurrence on IL13RA2-targeted CAR T cell therapy (Brown et al. 2016). Single cell analysis of a tumor resected prior to CAR T cell therapy revealed the existence of an inflamed tumor microenvironment including a CD8+ cytotoxic, clonally expanded and antigen specific T cell population that disappeared in the recurrent setting. Longitudinal tracking of T cell receptors uncovered distinct T cell dynamics classes in the CSF during CAR T cell therapy. These included T cell clones with transient dynamics, representing intraventricular CAR T cell delivery and endogenous T cell recruitment from the PB into the CSF; and a group of T cells in the cerebrospinal fluid, that tracked with clonally expanded tumor resident T cells and whose dynamics contracted concomitantly with tumor volume. Our results suggest the existence of an endogenous T cell population that was invigorated by intraventricular CAR T cell infusions, and combined with the therapy to produce a complete response.
Biography
Vanessa Jonsson is an Assistant Professor in the Departments of Applied Mathematics and Biomolecular Engineering, and Faculty Affiliate at the Genomics Institute at the University of California, Santa Cruz. Professor Jonsson leads a computational systems immunology research group, focussing on interdisciplinary research combining genomics, data driven modeling and immunology to understand the dynamic interactions between host immunity, human disease and therapy (e.g. cancer immunotherapy, viral infection/antibody response). Vanessa Jonsson completed her PhD in Control and Dynamical Systems at Caltech, where she was co-advised by Richard Murray and David Baltimore, followed by a postdoctoral fellowship jointly between Caltech/UCSF, and a research professorship at City of Hope National Medical Center. She is the recipient of the NIH/NCI K12 award in cancer immunology.